Abstracts
The genetic code is degenerate, i.e. some amino acids are coded for by more than one codon. Although coding for the same amino acid, synonymous codons are not equally used in different genomes, and even in a single genome the synonymous codon usage can vary widely among genes, or even along the gene sequences. With the recent advent of full genome sequences we are starting to unravel the reasons for these deviations. In this review we will cover some of the proposed factors that might cause different codon usage bias and the selective forces influencing it.
codon usage; codon bias; translation; expression level; selection
O código genético é degenerado, isto é, o mesmo amino ácido pode ser codificado por vários codons. Apesar de codificarem o mesmo amino ácido, estes codons sinônimos não são utilizados da mesma forma em genomas diferentes, e mesmo em um único genoma o padrão de uso dos codons sinônimos pode variar muito entre os genes, ou ainda ao longo de um único gene. Com a recente introdução de seqüências genômicas completas as razões destes desvios no uso de codons estão começando a ser entendidas. Neste artigo nós vamos apresentar alguns dos fatores propostos para as variações no uso de codons sinônimos e as forças seletivas que podem influenciar tais variações.
REVIEW
Factors influencing codon usage bias in genomes
Hannah M. W. Salim; Andre R. O. Cavalcanti* * e-mail: aroc@pomona.edu
Biology Department, Pomona College, 175 W 6th Street, Claremont, CA 91711, USA
ABSTRACT
The genetic code is degenerate, i.e. some amino acids are coded for by more than one codon. Although coding for the same amino acid, synonymous codons are not equally used in different genomes, and even in a single genome the synonymous codon usage can vary widely among genes, or even along the gene sequences. With the recent advent of full genome sequences we are starting to unravel the reasons for these deviations. In this review we will cover some of the proposed factors that might cause different codon usage bias and the selective forces influencing it.
Keywords: codon usage, codon bias, translation, expression level, selection
RESUMO
O código genético é degenerado, isto é, o mesmo amino ácido pode ser codificado por vários codons. Apesar de codificarem o mesmo amino ácido, estes codons sinônimos não são utilizados da mesma forma em genomas diferentes, e mesmo em um único genoma o padrão de uso dos codons sinônimos pode variar muito entre os genes, ou ainda ao longo de um único gene. Com a recente introdução de seqüências genômicas completas as razões destes desvios no uso de codons estão começando a ser entendidas. Neste artigo nós vamos apresentar alguns dos fatores propostos para as variações no uso de codons sinônimos e as forças seletivas que podem influenciar tais variações.
1. Introduction
The genetic code is the set of rules that define the correspondence between nucleotide triplets - codons - in DNA and amino acids in proteins. One of the main characteristics of the code is that it is degenerate, i.e., multiple synonymous codons specify the same amino acid.
Of the 20 amino acids in the genetic code, nine are coded for by two synonymous codons, one is coded by three (as is the stop signal), five are coded for by four, three are coded for by six, and only two amino acids are coded for by one codon.
Because they all code for the same amino acid, it would be predicted that all synonymous codons for any chosen amino acid would appear randomly distributed along the genes. Take for example, the three codons that specify isoleucine. Given that they all code for the identical amino acid, it would be predicted that AUU, AUC, and AUA would all appear in DNA strands one-third of all the times that isoleucine is coded for.
However, beginning in the early 1980s, studies have indicated this is not the case.1-3 Synonymous codons do not appear to be used equally and randomly to code for an amino acid. Some codons are repeatedly preferred over others; this phenomenon is termed codon usage bias. Codon usage frequencies in fact vary among genomes, among genes, and within genes.4
To further the study of codon bias, numerous measures to quantify codon usage have been developed. These include the widely used measures CAI and ENC, among others. The Codon Adaptation Index5 (CAI) was developed in 1987 and uses a reference set of genes in a given species to determine which codons are preferred. The CAI score for a gene is calculated from the frequency of use of all codons in that gene. The index can be used to compare codon usage in different genes and in different organisms.5 The Effective Number of Codons6 (ENC) index was developed three years later in 1990. The measure quantifies how far the codon usage of a gene departs from equal usage of synonymous codons using codon usage data and is independent of gene length and amino acid composition. Unlike other measures of codon bias, such as CAI, ENC does not rely on organism-specific data and is easily applied to the study of new organisms. Both of these indices demonstrate that the usage of codons coding for an amino acid can show no bias - all codons appear an equal number of times when coding for a particular amino acid - or complete bias in that only one codon out of all synonymous codons appears in a gene, or genome, to code for that amino acid. While CAI delivers values ranging from 0 (no bias) to 1.0 or larger (complete bias), ENC values range from 61 (no bias; each of the 61 sense codons used equally) to 20 (complete bias; only one codon is used for each amino acid).6
Observed codon bias, combined with the assumption that codons are in fact synonymous, raises the question of why codon preference has evolved. If selection has not driven the evolution of codon usage bias on the grounds that increased fitness will result, why did codon preferences originate and remain conserved in almost all genes and genomes? Numerous investigations have sought to explain the factors that determine codon usage bias, including: translation optimization, gene expression, rates of evolution, protein secondary structure, location within a gene, and replication conditions. Ultimately, although codon usage bias is determined by many different factors, it appears that the original assumption is incorrect. The term "synonymous codons" is innately misleading; not all codons are created equal. The use of one codon over its synonyms does affect fitness, and selection has primarily driven the evolution of codon bias.7
2. Factors Affecting Codon Usage Bias
2.1. Selection for optimized translation
Translation is very energetically expensive;8 inefficient and inaccurate translation wastes limited cellular resources. Throughout the evolution of genomes, mutations that reduce the energy required for translation have been favored.9 The phenomenon of codon usage bias is thus often explained by selection for translational optimization. This hypothesis contends that the use of optimal codons can increase both the efficiency and the accuracy of translation.10-13
Codon usage is biased toward the use of certain tRNAs that are most abundant in the tRNA pool or toward codons that bind their cognate tRNAs more strongly than other synonymous codons.14-16 Codon bias avoids slowly translated codons, which are more prone to incorporate the wrong amino acid.17,18 Evidence from studies of E. coli and other prokaryotes supports this hypothesis, showing that the use of codons cognate to the most abundant tRNAs from a genome increases the rate at which a peptide chain grows and the overall accuracy of translation in that genome.10,11 Host-phage relationships have revealed that the codon usages of Staphylococcus aureus phages, T4 phages, and Aeh1 phages are all highly influenced by, if not almost identical to, the codon biases of their hosts. This suggests that the phages codon usages are largely determined by the most abundant tRNAs of their hosts.19
Some recent work has indicated that the optimization of translation efficiency and accuracy is achieved by having more abundant tRNAs, but of fewer different types. This allows for specific tRNAs that have accurate codon:anticodon interactions to be employed more often than those that are ineffective.15
The theory of selection for translation efficiency, although accepted as a broad real concept, does not go undisputed. For example, it was found that, considering the large role that expression breadth (the number of tissues in which a gene is expressed) of genes plays in determining codon bias, translation selection has no effect on codon preference.20
Other recent studies have revealed that the model of selection for translation efficiency may simply be much more complicated than originally thought. One major area of debate is the question as to why some tRNAs are more abundant than others in the first place. In a study of 102 bacterial species, it was found that, although codon usage bias in highly expressed genes seems to result from the selection of optimal codons associated with the most frequent tRNA genes, the increase in frequency of these tRNA genes also results from codon usage bias.15 This leads to the concept of a co-evolution, which has left the expression of highly expressed genes a more efficient process, or to the idea that different tRNA abundances evolved directly from pre-existing codon biases.15 Also, major codons are always preferentially used by highly expressed genes, regardless of protein secondary structure. This suggests that tRNA abundance is a consequence of codon bias, not the determining factor of it.21
2.2. Expression
One widely studied force behind codon usage bias is gene expression. Gene expression is the process by which the information within double-stranded DNA is transcribed into messenger RNA (mRNA) and then, following post transcriptional modification, it is translated by ribosomes to produce a protein polypeptide. A highly expressed gene is a gene that is expressed often, producing greater than average levels of protein. A broadly expressed gene is one that is expressed in many tissues.
Studies of the genomes of a wide variety of organisms have revealed a correlation between gene expression level and codon usage bias, namely that high gene expression leads to high bias.1 In genes that are translated often and at high volumes, codon bias appears to be especially high because the cost of a missense error is elevated. The ability to produce more accurately translated sequences faster through codon bias in highly expressed genes is thus selected for.
Recent research, however, indicates that high codon bias is not necessarily indicative of highly expressed genes. For example, a study of the human genome found that some lowly expressed genes, as well as highly expressed genes, are characterized by high codon bias.22 Another study in humans examined the relationship between gene expression level and gene expression breadth and codon bias and showed that codon usage is more strongly related to breadth of expression than to maximum expression level.16 On the other hand, a study in the aspen tree, Populus tremula, used path analysis to correct calculations for the directly and indirectly correlated effects that expression level, expression breadth, rates of protein evolution, and protein length have on one another when studying codon bias. This study found that, when the influence of other variables is removed, expression level has the largest direct effect on codon usage bias.20
2.3. Location within genes
The degree of codon usage bias in a gene can vary based on codon location within a gene sequence. In Saccharomyces cerevisiae and the genomes of four prokaryotes, codon bias increases along genes in the direction of translation.23 This location-based pattern of codon preference has been explained by two different hypothesis.
First, the existence of low-usage codon clusters slows translation extensively; when these clusters are located at the 3 end of a gene, so much ribosomal slowing occurs upstream that it is as if the entire sequence were composed of low-usage codons.24 Thus, the increased number of optimal codons at the 3 end of a gene increases the speed of translation and works to prevent ribosomal pile-up.
Second, the abundance of optimal codons may increase along the length of a gene sequence in order to prevent nonsense errors that would become increasingly expensive. In E. coli this pattern of increasing codon bias is stronger in longer genes than in shorter genes, and codon bias is positively correlated with gene length.23 This suggests that as a gene becomes longer, and more energy is required for translation, it is increasingly important to prevent nonsense errors at the 3 end of a gene that would terminate translation prematurely and make the peptide synthesized up to that point useless.
In Drosophila melanogaster codon bias within genes exists in a symmetrical M-shaped pattern, with decreased bias in the middle of genes.23 This has been explained by the authors using the Hill-Robertson effect,23 in which there is interference between selection at different loci.25
2.4. Rate of evolution
Studies on Saccharomyces cerevisiae, Drosophila melanogaster, Escherichia coli, and Salmonella typhimurium have revealed a significant negative correlation between codon usage bias and the rate of nucleotide substitution at silent sites.5,17,26,27 The study that looked at Escherichia coli and Salmonella typhimurium found that, additionally, highly expressed genes have high codon bias and low rates of synonymous substitution.5
Codon preferences reflect a balance between mutational biases and natural selection for translational optimization, and as mentioned before, optimal codons help to increase translation efficiency and accuracy.28 Since optimal codons are favored by selection, and a synonymous substitution to a non-optimal codon would actually decrease fitness, selection among synonymous codons constrains the rate of silent substitution in some genes.17,26
2.5. Secondary structure
The secondary structural constraints of DNA also play an active role in determining the codon preferences of genes. This holds true in the genomes of most organisms, including those of chickens, Drosophila melanogaster, Caenorhabditis elegans, Saccharomyces cerevisiae, and the bacterial genes of E. coli, H. influenzae, B. subtilis, and M. genitalium. This was interpreted to suggest that these structural constraints, such as DNA and mRNA flexibility capabilities and folding stabilities, play a more important role in determining codon usage than translational constraints do.29
The transcription of DNA is highly constrained by the ability of DNA strands to bend and be flexible during transcription.30 These structural properties are influenced by base sequence and length, which may reflect or influence codon bias, and which often correlate to gene expression levels. DNA that cannot condense tightly into wrapped chromatin, is more accessible to RNA polymerase and thus more highly expressed.30
Recent studies also reveal that the choice of different codons in the section of an mRNA sequence responsible for coding for protein secondary structures contributes to the folding stability.31 This folding stability can go on to affect translation accuracy and efficiency. Additionally, this suggests that mRNA folding stability might be important in regulating gene expression by influencing codon bias in highly and lowly expressed genes. Studies show that the stability of mRNA folded structure works to discriminate between the highly and lowly expressed genes coding for irregular portions of protein secondary structure on the basis of amino acid usage of S. cerevisiae.21
2.6. Nucleotide composition
Codon bias may also be shaped by preferences on the level of nucleotide sequence, specifically the GC content of coding regions. Different organisms display different propensities toward GC poor or GC rich genomes. The genome of the ciliate Oxytricha trifallax has a GC content of only 39%, with a preference for synonymous codons containing A or T,32 while the aspen tree, Populus tremula, prefers codons ending in G or C.20
Varying compositional patterns have been shown to be pervasive in eukaryotes,33 and GC content has been found to be correlated to codon usage bias,34 gene length,35 gene density,36 replication timing,37 and methylation38 among other things. Despite the extensive research on nucleotide composition, the absolute cause behind these trends has yet to be determined. Hypotheses have been proposed, however, including the ideas that nucleotide patterns may be determined by selection,39,40 mutational bias,41,42 or recombination, since there is an association between recombination and GC-rich chromosomal regions.43,44
2.7. Protein length
When mRNA concentration is controlled for, and thus genes of similar expression levels are compared, protein length and codon usage bias are positively correlated in both Saccharomyces cerevisiae and Escherichia coli.12,45,46 The opposite correlation has been described for Drosophila melanogaster genes.46 Translational selection has been used to explain both of these correlations. The cost of translating a protein is proportional to its length, so there is greater pressure for the selection of the most accurate codons in longer genes to avoid missense errors, explaining the positive correlation.12,45 It has also been argued that selection may act to decrease the length of highly expressed genes, especially in eukaryotes, explaining the negative correlation.46
Recently, a new index of codon bias was developed to control for the influence of gene length on codon bias. Measurement Independent of Length and Composition (MILC) is a measure that is resistant to changes in gene length and overall nucleotide composition, reducing the noise introduced to measurements.47
2.8. Environment
Environmental conditions, including the types of tissues in which genes are expressed and the specific cellular conditions within these tissues, also play a role in influencing codon preferences. A study of genes expressed in multiple human tissues found that codon usage differs for sets of genes expressed in different tissues and is directly affected by the actual amount of tRNA molecules in each tissue.16 This harkens directly back to the hypothesis of selection for translational optimization. A second study in human tissues found that varying abundances of tRNA isoacceptors are found in different tissues, suggesting a relationship between tRNA abundance and codon usage in different tissues. For instance, the TCT and CCT Arg isoacceptors were found to be preferred over the ICG and YCG isoacceptors in selected nonbrain tissues (liver, thymus, and lymph node), suggesting a preference for reading AGA and AGG codons.48 This suggests a regional variation in codon bias above and beyond the already mentioned selection for translational performance.
The conditions under which a gene is replicated also appear to affect codon preferences. For example, it has been shown that an overrepresentation of rare codons is seen in genes expressed under starvation conditions.15 This suggests that during the evolution of genomes, different conditions providing different restrictions on gene expression have influenced codon preferences. In yeast the functional constraints acting on genes might have varied greatly due to the effect of growing environment during Saccharomyces evolution.27 It appears that, in vivo, intracellular factors contribute to the final formation of proteins with influence from ribosomal traffic, chaperones, stress proteins, and foldases. These differing factors also correlate to varying codon preferences.49
2.9. Time
Another determinant of codon bias is the time and speed of expression, that is, when during the life of a cell and how quickly does replication takes place. Fast-growing bacteria have more abundant, less diverse tRNAs, leading to higher codon bias in highly expressed genes.15 In other fast-growing organisms, proteins involved in transcription and translation are often highly expressed and biased in their codon usage; they tend to have significantly high CAI values.50 In slow-growing organisms with low codon biases, CAI is a less effective indicator of highly expressed genes.51
An organism itself does not have to be habitually slow or fast-growing to illicit codon bias trends. The time of replication plays an important role in codon biases within genes and genomes.28 In Populus tremula tissues in which cells are currently undergoing growth and division at a rapid pace, association between codon bias and gene expression is significantly stronger than in cells of the same tissues growing at a slower pace.20 With so many possible environmental and temporal factors affecting codon bias, it is difficult to predict and characterize when and to what extent codon preferences will occur.
2.10. Neutral alternatives
It has been argued that neutral processes, like gene conversion or mutational biases, could explain certain characteristic patterns of codon bias. For example, it is known that transcription is mutagenic;52 this could cause genes that are transcribed frequently (i.e. genes with a higher expression level) to have larger codon bias as a side effect. However, a study using the Drosophila and the C. elegans genomes showed that this transcription-coupled mutational process could not explain the observed codon bias in these species and that synonymous codon usage in these organisms is shaped by natural selection.53,54
Another neutral process, biased gene conversion, is sometimes invoked to explain the correlation between codon bias and protein sequence evolution.55 In a recent study of duplicated genes in the yeast genome, the authors showed that gene conversion plays only a minor role in decreasing the rate of evolution of proteins, while codon bias and functional constraints are the major determinants of evolutionary rate.56 Furthermore, they suggest that gene conversion alone should not be able to maintain sequence similarity in the long-run, while codon bias or other functional constraints are able to decrease sequence evolution in the long-term, even in the absence of gene conversion.56
In summary, although in theory neutral process could explain some aspects of codon usage bias, the majority of the evidence suggests that natural selection acting on traits with very small phenotypic effects is responsible for the emergence and maintenance of codon usage biases.
3. Concluding Remarks
The availability of complete genome sequences allows the observation of a more complete portrait of codon preferences within and between genomes. The picture that is emerging is a complex one with codon bias being influenced by a gamut of factors that just now are starting to be unraveled.
Different studies show that synonymous codon usage can be influenced by: selection for translation accuracy or efficiency, expression level of the gene, selective forces acting on the gene sequence, location inside the gene, composition of the genomes or genome regions, gene length, time and location where a gene is expressed, mRNA structure and stability and protein secondary structure. Much more work and the sequences of more genomes will be necessary to untangle the effects of all these factors.
Acknowledgements
From Andre Cavalcanti: Prof. Ricardo Ferreira was my research advisor since I was an undergraduate until I finished my PhD. I cant think of a better mentor and friend. His support was fundamental in all steps of my academic life. I would like to congratulate him and send my best wishes for his 80th birthday, and take the opportunity to thank him for his advise, guidance and friendship throughout my scientific career.
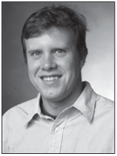
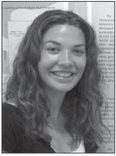
Received: July 30, 2007
Web Release Date: December 3, 2007
- 1. Gouy, M.; Gautier, C.; Nuc. Acids Res. 1982, 10, 7055.
- 2. Bennetzen, J.L.; Hall, B.D.; J. Biol. Chem. 1982, 257, 3026.
- 3. Staden, R.; McLachian, A. D.; Nuc. Acids Res. 1982, 10, 141.
- 4. Hooper, S. D.; Berg, O. G.; Nuc. Acids Res. 2000, 28, 3517.
- 5. Sharp, P. M.; Li W. H.; Nuc. Acids Res. 1987, 15, 1281.
- 6. Wright, F.; Gene 1990, 87, 23.
- 7. Dey, S.; J. Genet. 2004, 83, 113.
- 8. Akashi, H.; Gojobori. T.; Proc. Natl. Acad. Sci. USA 2002, 99, 3695.
- 9. Heizer, E. M.; Raiford, D. W.; Raymer, M. L.; Doom, T. E.; Miller, R. V.; Krane, D. E.; Mol. Biol. Evol. 2006, 23, 1670.
- 10. Bulmer, M.; Genetics 1991, 129, 897.
- 11. Akashi, H.; Genetics 1994, 136, 927.
- 12. Coughlan, A.; Wolfe, K. H.; Yeast 2006, 16, 1131.
- 13. Drummond, D.; Raval, A.; Wilke, C.; Mol. Biol. Evol. 2006, 23, 327.
- 14. Andersson, S.G.E.; Kurland, C.G.; Microbiol. Rev. 1990, 54, 198.
- 15. Rocha, E. P. C.; Genome Res. 2004, 14, 2279.
- 16. Kotlar, D.; Lavner, Y.; BMC Genomics 2006, 7, 67.
- 17. Powell, J. R.; Moriyama, E. N.; Proc. Natl. Acad. Sci. USA 1997, 94, 7784.
- 18. Gilchrist, M. A.; Wagner, A.; J. Theor. Biol. 2006, 239, 417.
- 19. Sau, K.; J. Microbiol. Immunol. Infect. 2007, 40, 24.
- 20. Ingvarsson, P.; Mol. Biol. Evol. 2007, 24, 836.
- 21. Kahali, B.; Basak, S.; Ghosh, T. C.; Biochem. Biophys. Res. Commun. 2007, 354, 693.
- 22. Lavner, Y.; Kotlar, D.; Gene 2005, 345, 127.
- 23. Qin, H.; Wu, W. B.; Comeron, J. M.; Kreitman, M.; Li, W. H.; Genetics 2004, 168, 2245.
- 24. Zhang, S.; Goldman, E.; Zubay, G.; J. Theor. Biol. 1994, 170, 339.
- 25. Hill, W. G.; Robertson, A.; Genet. Res. 1966, 8, 269.
- 26. Sharp, P. M.; Li, W. H.; J. Mol. Evol. 1989, 28, 398.
- 27. Kawahara, Y.; Imanishi, T.; BMC Evol. Biol. 2007, 7, 9
- 28. Akashi, H.; Curr. Opin. Genet. Dev. 2000, 11, 660.
- 29. Karlin, S.; J. Mol. Biol. 1996, 262, 459.
- 30. Willenbrock, H.; Ussery, D. W.; BMC Mol. Biol. 2007, 8, 11.
- 31. Jia, M.; Luo, L.; Biochem. Biophys. Res. Commun. 2006, 343, 177.
- 32. Cavalcanti, A.R.O.; Stover, N.A.; Orecchia, L.; Doak, T.G.; Landweber, L.F.; Chromosoma 2004, 113, 69.
- 33. Nekrutenko, A.; Li, W. H.; Genome Res. 2000, 10, 1986.
- 34. Sharp, P. M.; Averof, M.; Lloyd, A. T.; Matassi, G.; Peden, J. F.; Philos. Trans. R. Soc. Lond. B, Biol. Sci. 1995, 349, 241.
- 35. Duret, L.; Mouchiroud, D.; Gautier, C.; J. Mol. Evol. 1995, 40, 308.
- 36. Mouchiroud, D.; DOnofrio, G.; Aïssani, B.; Macaya, G.; Gautier, C.; Bernardi, G.; Gene 1991, 100, 181.
- 37. Watanabe, Y.; Fujiyama, A.; Ichiba, Y.; Hattori, M.; Yada, T.; Sakaki, Y.; Ikemura, T.; Hum. Mol. Genet. 2002, 11, 13.
- 38. Jabbari, K.; Bernardi, G.; Gene 1998, 224, 123.
- 39. Bernardi, G.; Bernardi, G.; J. Mol. Evol. 1986, 24, 1.
- 40. Bernardi, G.; Gene 2000, 259, 31.
- 41. Filipski, J.; FEBS Lett. 1987, 217, 184.
- 42. Wolfe, K. H.; Sharp, P. M.; Li, W. H.; Nature 1989, 337, 283.
- 43. Fullerton, S. M.; Carvalho, A.B.; Clark, A.G.; Mol. Biol. Evol. 2001, 18, 1139.
- 44. Meunier, J.; Duret, L.; Mol. Biol. Evol. 2004, 21, 984.
- 45. Eyre-Walker A.; Mol. Biol. Evol. 1996, 13, 864.
- 46. Moriyama, E. N.; Powell, J. R.; Nuc. Acids Res. 1998, 26, 3188.
- 47. Supek, F.; Vlahovicek, K.; BMC Bioinformatics 2005, 6, 182.
- 48. Dittmar, K. A.; Goodenbour J. M.; Pan, T.; PLOS Genetics 2006, 2, e221.
- 49. Komar, A. A.; FEBS Lett. 1997, 415, 6.
- 50. Carbone, A.; Kepes, F.; Zinovyev, A.; Mol. Biol. Evol. 2005, 22, 547.
- 51. Willenbrock, H.; Friis, C.; Juncker, A. S.; Ussery, D. W.; Genome Biol. 2006, 7, R114.
- 52. Francino, M. P.; Ochman, H.; Mol. Biol. Evol. 2001, 18, 1147.
- 53. Duret, L.; Mouchiroud, D.; Proc. Natl. Acad. Sci. USA 1999, 96, 4482.
- 54. Duret, L.; Curr. Opin. Genet. Dev. 2002, 12, 640.
- 55. Kellis, M.; Birren, B. W.; Lander, E.S.; Nature 2004, 428, 617.
- 56. Lin, Y.; Byrnes, J.; Hwang, J.; Li, W.; Proc. Natl. Acad. Sci. USA 2006, 103, 14412.
Publication Dates
-
Publication in this collection
08 Apr 2008 -
Date of issue
2008
History
-
Accepted
03 Dec 2007 -
Received
30 July 2007