Abstract
Abstract: In this paper, we introduce a new three-parameter distribution by compounding the Nadarajah-Haghighi and geometric distributions, which can be interpreted as a truncated Marshall-Olkin extended Weibull. The compounding procedure is based on the work by Marshall and Olkin 1997. We prove that the new distribution can be obtained as a compound model with mixing exponential distribution. It can have decreasing, increasing, upside-down bathtub, bathtub-shaped, constant and decreasing-increasing-decreasing failure rate functions depending on the values of the parameters. Some mathematical properties of the new distribution are studied including moments and quantile function. The maximum likelihood estimation procedure is discussed and a particle swarm optimization algorithm is provided for estimating the model parameters. The flexibility of the new model is illustrated with an application to a real data set.
Key words
Exponential distribution; Failure rate function; Geometric distribution; Maximum likelihood estimation; Nadarajah-Haghighi distribution.
INTRODUCTION
Nadarajah and Haghighi (2011)NADARAJAH S AND HAGHIGHI F. 2011. An extension of the exponential distribution. Statistics 45: 543-558. introduced and studied the mathematical properties of an extension of the exponential distribution that allows for increasing, decreasing and constant hazard rate functions (hrfs). The model is referred to as the Nadarajah-Haghighi (NH) distribution. Its cumulative distribution function (cdf) is given by
where is the scale parameter and is the shape parameter. If follows the Nadarajah-Haghighi distribution, we shall denote by . The corresponding probability density function (pdf) is given by
The NH distribution presents several advantages if compared with some well-known generalizations of the exponential model, such as the gamma, Weibull and exponentiated exponential (EE) distributions. For example, unlike these distributions, the NH distribution allows for an increasing hrf when its corresponding density is monotonically decreasing. The second advantage is the ability to model data that have their mode fixed at zero. Another advantage is based on a mathematical relationship with the Weibull distribution, where the NH model can be interpreted as a truncated Weibull distribution. These three facts combined may attract more complex applications in the literature of lifetime distributions.
The NH distribution is not the only extension of the exponential distribution. In addition to the gamma and Weibull distributions, many other generalizations have been proposed over the years. One of them is
where and . Note that the EE distribution, discussed in Gupta, Gupta, and Gupta (1998)GUPTA RC, GUPTA PL AND GUPTA RD. 1998. Modeling failure time data by Lehman alternatives. Commun Stat Theory Methods 27(4): 887-904., is actually a particular case of equation(3) when . Nadarajah and Kotz (2006)NADARAJAH S AND KOTZ S. 2006. The exponentiated type distributions. Acta Applicandae Mathematica 92: 97-111. and Barreto-Souza, Santos, and Cordeiro (2010)BARRETO-SOUZA W, SANTOS AH AND CORDEIRO GM. 2010. The beta generalized exponential distribution. J Stat Comput Simul 80: 159-172. proposed two important extensions of the exponential model, which are the beta exponential (BE) and beta generalized exponential (BGE) distributions, respectively. The BE and BGE distributions are special cases of the beta family of distributions, pionnered by Eugene, Lee, and Famoye (2002)EUGENE N, LEE C AND FAMOYE F. 2002. Beta-normal distribution and its applications. Commun Stat Theory Methods 31: 497-512.. The beta family still contains other generalizations of the exponential model such as the beta Weibull (BW) (Lee, Famoye, and Olumolade 2007)LEE C, FAMOYE F AND OLUMOLADE O. 2007. Beta-Weibull distribution: some properties and applications to censored data. J Mod Appl Stat Methods 6: 17. and beta modified Weibull (BMW) (Silva, Ortega, and Cordeiro 2010)SILVA GO, ORTEGA EM AND CORDEIRO GM. 2010. The beta modified Weibull distribution. Lifetime Data Anal 16: 409-430. distributions. The Kumaraswamy class, introduced by Cordeiro and Castro (2011)CORDEIRO GM AND DE CASTRO M. 2011. A new family of generalized distributions. J Stat Comput Simul 81: 883-898., also contains several models that extend the exponential distribution, such as the Kumaraswamy Weibull (KwW) (Cordeiro, Ortega, and Nadarajah 2010)CORDEIRO GM, ORTEGA EM AND NADARAJAH S. 2010. The Kumaraswamy Weibull distribution with application to failure data. J Franklin I 347: 1399-1429. and Kumaraswamy generalized Rayleigh (KwGR) (Gomes et al. 2014)GOMES AE, DA-SILVA CQ, CORDEIRO GM AND ORTEGA EM. 2014. A new lifetime model: the Kumaraswamy generalized Rayleigh distribution. Journal of Statistical Computation and Simulation 84: 290-309. distributions. References about other generalizations of the exponential model are widespread and the reader can see those listed in the above papers.
In this paper, we introduce a new continuous distribution, which is an extension of the NH model, by compounding the NH and geometric distributions. The new model is therefore another extension of the exponential distribution and is referred to as the Nadarajah-Haghighi geometric (NHG) distribution. The proposed distribution is more flexible for modeling lifetime data, namely in reliability, in terms of its failure rate shapes, which maybe be constant, decreasing, increasing, upside-down bathtub and bathtub shaped. The compounding procedure follows the pioneering work by Albert W Marshall and Olkin (1997)MARSHALL AW AND OLKIN I. 1997a. A new method for adding a parameter to a family of distributions with application to the exponential and Weibull families. Biometrika 84: 641-652.. In the same way, several classes of distributions were proposed by compounding some useful lifetime and power series (PS) distributions in the last few years. Chahkandi and Ganjali (2009)CHAHKANDI M AND GANJALI M. 2009. On some lifetime distributions with decreasing failure rate. Comput Stat Data Anal 53: 4433-4440. introduced the exponential power series (EPS) class of distributions, which contains as special cases the exponential Poisson (EP), exponential geometric (EG) and exponential logarithmic (EL) distributions. Morais and Barreto-Souza (2011)MORAIS AL AND BARRETO-SOUZA W. 2011. A compound class of Weibull and power series distributions. Comput Stat Data Anal 55: 1410-1425. defined the Weibull power series (WPS) class, which includes, as sub-models, the EPS distributions. The WPS distributions can have increasing, decreasing and upside down bathtub hrfs. The generalized exponential power series (GEPS) distributions were proposed by Mahmoudi and Jafari (2012)MAHMOUDI E AND JAFARI AA. 2012. Generalized exponential-power series distributions. Comput Stat Data Anal 56: 4047-4066. following the same approach of Morais and Barreto-Souza (2011)MORAIS AL AND BARRETO-SOUZA W. 2011. A compound class of Weibull and power series distributions. Comput Stat Data Anal 55: 1410-1425.. Silva et al. (2013)SILVA RB, BOURGUIGNON M, DIAS CR AND CORDEIRO GM. 2013. The compound class of extended Weibull power series distributions. Comput Stat Data Anal 58: 352-367. studied the extended Weibull power series (EWPS) family, which includes as special models the EPS and WPS families. Bourguignon, Silva, and Cordeiro (2014)BOURGUIGNON M, SILVA RB AND CORDEIRO GM. 2014. A new class of fatigue life distributions. J Stat Comput Simul 84: 2619-2635. extended the Birnbaum-Saunders distribution through the class of Birnbaum-Saunders power series (BSPS) distributions. In a recent paper, (Silva and Cordeiro 2015)SILVA RB AND CORDEIRO GM. 2015. The Burr XII power series distributions: A new compounding family. Brazilian Journal of Probability and Statistics 29(3): 565-589. introduced the Burr XII power series (BXIIPS) family of distributions.
The rest of the paper is organized as follows. Section 2 introduces the NHG distribution and presents some special models. Further, we demonstrate that the NHG density function can be expressed as a mixture of densities of minimum of the baseline order statistics and study some of its properties. In Section 3, the estimation procedure is approached by maximum likelihood via a particle swarm optimization approach. Section 4 deals with bootstrap percentile intervals. Numerical results from Monte Carlo simulation experiments are investigated in Section 5. We also perform simulations to assess the use of bootstrap percentiles (simple and double) for construction of confidence intervals for the parameters. An application to real data is performed in Section 6. Finally, concluding remarks are presented in Section 7.
CONSTRUCTION OF THE NHG DISTRIBUTION
Let be independent and identically distributed (iid) NH random variables with cdf(1) and pdf(2). We assume that has a zero-truncated geometric distribution independent of the ’s with probability mass function (pmf) given by
with . Defining , the conditional random variable has cdf
The NHG distribution is defined by the marginal cdf of X
Hereafter, the random variable according(4) with parameters and is denoted by NHG. The pdf of is
It can be shown that
Even when , Equation (5) is a density function. We can then define the NHG distribution by (5) for any . The study of the new distribution is important since it extends some distributions previously considered in the literature. In fact, the NH distribution is obtained by taking , please see (Nadarajah and Haghighi 2011)NADARAJAH S AND HAGHIGHI F. 2011. An extension of the exponential distribution. Statistics 45: 543-558.. The EG distribution (Adamidis and Loukas 1998)ADAMIDIS K AND LOUKAS S. 1998. A lifetime distribution with decreasing failure rate. Stat Probabil Lett 39: 35-42. follows by taking and , whereas the EEG distribution (Adamidis, Dimitrakopoulou, and Loukas 2005)ADAMIDIS K, DIMITRAKOPOULOU T AND LOUKAS S. 2005. On an extension of the exponential-geometric distribution. Stat Probabil Lett 73: 259-269.. is obtained when for any . Clearly, the EEG distribution extends the EG distribution. For and , Equation (1) reduces to the exponential distribution. When ( tending to from by the left), the NHG distribution distribution converges to a distribution degenerated at zero, i.e., . Hence, the parameter can be interpreted as a degeneration parameter. Figure 1 displays the pdf of for selected parameter values.
Proposition 1. For any , the pdf of the NHG distribution is monotonous if and and the pdf is strictly decreasing if and .
Proof. The proof of this result follows directly by derivation criteria.
Proposition 2.The NHG density function is log-convex if and , and it is log-concave if and .
Proof. Let . It implies that for . We have . Now, rewriting the NHG density function as a function of say, we obtain
As a simple derivation exercise, deriving with respect to , it is possible to observe that .
The second derivative of with respect to is given by
The main motivation for the new distribution is based on four points:
-
Ability (or the inability) of the NHG distribution to model data that have their mode fixed at zero.
-
As we shall see later, the NHG hrf can be constant, decreasing, increasing, decreasing-increasing-decreasing, upside-down bathtub, bathtub-shaped or constant.
-
If is a Marshall-Olkin extended Weibull random variable with shape parameter and scale parameter , then the density in (5) is the same as that one of the random variable truncated at zero; that is, the NHG distribution can be interpreted as a truncated Marshall-Olkin extended Weibull distribution.
-
It can be applied in some interesting situations as follows:
Time to relapse of cancer under the first-activation scheme. Here, is the number of carcinogenic cells for an individual left active after the initial treatment and is the time spent for the th carcinogenic cell to produce a detectable cancer mass, for ;
Time to the first failure. Suppose that the failure of a device occurs due to the presence of an unknown number of initial defects of same kind, which can be identifiable only after causing failure and are repaired perfectly;
Reliability. From the stochastic representations and , we note that the NHG model can arise in series and parallel systems with identical components, which appear in many industrial applications and biological organisms.
Proposition 3. The distribution of the form (4) is geometric extreme stable.
Proof. The proof follows easily using the arguments by Albert W. Marshall and Olkin (1997)MARSHALL AW AND OLKIN I. 1997b. A new method for adding a parameter to a family of distributions with application to the exponential and Weibull families. Biometrika 84: 641-652.. So, we omitted the details.
Proposition 4. The density function of can be expressed as a mixture of densities of minimum order statistics of .
Proof. For any positive real number , and for , we have the generalized binomial expansion (Prudnikov, Brychkov, and Marichev 1986)PRUDNIKOV P, BRYCHKOV A AND MARICHEV OI. 1986. Integrals and series: special functions. Volume 2. CRC Press..
where is the gamma function. Applying (5) to (6), yields
where and is the density function of , for fixed , given by
Remark 1. The cdf and pdf of are given by
and
The distribution with cdf (7) is called the complementary Nadarajah-Haghighi-geometric (CNHG) distribution. It is a suitable model in a complementary risk problem based in the presence of latent risks, which arise in several areas such as public health, actuarial science, biomedical studies, demography and industrial reliability (Basu and Klein 1982)BASU AP AND KLEIN JP. 1982. Some recent results in competing risks theory. Lecture Notes-Monograph Series 2: 216-229.. However, in this work, we do not focus on this alternative class of distributions.
Proposition 5. Let NHG. Then:
-
i) The cdf of the nth order statistic corresponding to the pdf (5) is given by
-
ii) The density function of the nth order statistic is given by
Proof. The proof of Proposition 4 is trivial.
The NHG survival function is given by
and the corresponding hrf (for ) becomes
where is the NH hrf.
Note that the ratio is increasing in for and decreasing in for . Further, we have
Proposition 6. For , the NHG distribution has an increasing hrf if and , and it has a decreasing hrf if and . It is constant if and .
Proof. The proof follows trivially by derivation criteria.
Figure 2 displays some plots of the NHG hrf for some parameter values. The parameter does not change the shape of the hrf since it is a scale parameter. It is evident that this hrf can be decreasing, increasing, upside-down bathtub shaped or bathtub-shaped. It is difficult to determine analytically the parameter ranges corresponding to these shapes. It is interesting to point out that the NHG hrf can also be decreasing-increasing-decreasing. So, this distribution is quite flexible and can be used effectively in analyzing real data in several areas. Thus, the beauty and importance of the new distribution lies in its ability to model monotone as well as non-monotone failure rates, which are quite common in reliability and biological studies.
Theorem 7. Suppose that the conditional survival function of a continuous random variable X is given by
If the parameter has the exponential distribution with pdf
then the compound distribution of has the NHG distribution.
Proof.
which is the NHG survival function.
The th moment of is given by
where is an integral to be numerically evaluated. This integral can be easily computed numerically in software such as Julia and R.
The quantile function (qf) corresponding to equation (4) is given by
Simulating the NHG random variable is straightforward. Let be a uniform variate on the unit interval . Thus, the random variable is given by
follows (5), i.e, NHG.MAXIMUM LIKELIHOOD ESTIMATION
In this section, we determine the maximum likelihood estimates (MLEs) of the parameters of the new distribution from complete samples only. Let be observed values from the NHG distribution with parameters and . Let be the parameter vector. The total log-likelihood function for is given by
By taking the partial derivatives of the log-likelihood function with respect to the parameters in , we obtain the components of the score vector :
where
Setting and equal to zero and solving the equations simultaneously yields the MLE of . These equations cannot be solved analytically and statistical software can be used to solve them numerically using iterative methods such as the Newton-Raphson type algorithms.
The normal approximation for the MLE of can be used for constructing approximate confidence intervals and for testing hypotheses on the parameters and . Under conditions that are fulfilled for the parameters in the interior of the parameter space, we can approximate the distribution of by the multivariate normal , where is the unit expected information matrix. The asymptotic behavior remains valid if , where is the observed information matrix, is replaced by the average sample information matrix evaluated at , i.e., . The elements of the observed information matrix can be obtained from the authors upon request.
OPTIMIZATION ALGORITHM
In computer science, the particle swarm optimization (PSO) is a computational method for optimization of parametric and multiparametric functions.
Further details on the philosophy of the PSO method are given in the book Swarm Intelligence (Kennedy, Kennedy, and Eberhart 2001)KENNEDY J, KENNEDY JF AND EBERHART RC. 2001. Swarm intelligence. Morgan Kaufmann..
The PSO algorithm optimizes a problem by having a population of candidate solutions and moving these particles around in the search-space according to simple mathematical formulae over the particle’s position and velocity. The movement of the particles in the search space is randomized. For each iteration of the PSO algorithm there is a leader particle, which is the particle that minimizes the objective function in the corresponding iteration. The remaining particles arranged in the search region will follow the leader particle randomly and sweep the area around this leading particle. In this local search process, another particle may become the new leader and the other particles will follow this new leader randomly. Each particle arranged in the search region has a velocity vector and position vector and its movement in the search region is given by changes in these vectors. The PSO algorithm is presented below, where is the objective function to be minimized, is the number of particles in the swarm (set of feasible points, i.e. search region), each particle having a vector position in the search-space and a vector velocity defined by . Let be the best known position of particle and the best position of all particles.
-
For each particle do:
-
Initialize the particle’s position with a uniformly distributed random vector: , where and are the lower and upper boundaries of the search-space.
-
Initialize the particle’s best known position to its initial position: .
-
If update the swarm’s best known position:
-
Initialize the particle’s velocity: .
-
-
Until a termination criterion is met (e.g. number of iterations performed, or a solution with adequate objective function value is found), repeat:
-
For each particle do:
-
- Pick random numbers: .
-
- For each dimension do:
-
* Update the particle’s velocity: .
-
-
- Update the particle’s position:
-
- If do:
-
* Update the particle’s best known position:
-
* If update the swarm’s best known position: .
-
-
-
-
Now holds the best found solution.
The parameter is called inertia coefficient and, as the name implies, controls the inertia of each particle arranged in the search region. The quantities and control the acceleration of each particle and are called acceleration coefficients. The PSO algorithm described above, which is implemented in the programming language R, is presented below.
This algorithm with few modifications will be implemented in the AdequacyModel package, which is available on the website of R. The algorithm above is quite general and can be applied to maximize any function involving or not a database. Using pso, a given function is maximized taking into consideration vectors of restrictions delimiting the search-space. In truth, the pso function above is constructed to minimize any function. However, to maximize is equivalent to minimize . A brief description of the arguments of the pso function are listed below.
-
func: Objective function that we want to maximize;
-
S: Number of particles considered. By default, the number of particles is equal to 150;
-
liminf e limsup: Vectors that restrict the region-search inferiorly and superiorly, respectively.
-
e: Error considered. The algorithm stops if the variance in the last 10 iterations is less than or equal to e;
-
data: By default data=NULL, but when the func is a log-likelihood function, data is a data vector;
-
N: Maximum number of iterations.
One advantage of the PSO method is that we do not need to concern ourselves with initial shots. Problems with initial shots are frequent in methods such as the BFGS when the objective function involves flat or nearly flat regions.
The example below shows clearly this problem and the use of the function pso, especially how to specify the objective function for the argument func. The PSO method is described in the A.
Example:
Consider Easom function , and . The Easom function is minimized at , with . The use of the pso function to minimize the above function is
easom <- function(par,x){ x1 = par[1] x2 = par[2] -cos(x1)*cos(x2)*exp(-((x1-pi)^2 + (x2-pi)^2)) } set.seed(0) pso(func=easom,S=350,lim_inf=c(-10,-10),lim_sup=c(10,10))
In both minimizations there is convergence. For minimization through BFGS method, we use the optim function of the R language with the argument method="BFGS". For more details on the optim function, do help(optim). Figure 3(a) presents four estimates obtained by the BFGS method, whose points are those of minimum obtained by this method, using the BFGS procedure with different initial shots. In the legend are described the initial shots. In these four estimates for different shots the optim function indicates convergence.
Before performing the pso function, the seed of the Mersenne-Twister generator proposed by Matsumoto and Nishimura (1998) is fixed at zero, i.e, set.seed(0). Is it possible to perceive clearly through Figure 3 that the minimization method using the PSO function pso is much more efficient and approaches better the global minimum.
BOOTSTRAP CONFIDENCE INTERVALS
The bootstrap method was introduced in 1979 by Efron (1979)EFRON B. 1979. Bootstrap methods: another look at the jackknife. The Annals of Statistics 7: 1-26.. This method was inspired by a previous methodology based on resampling called jackknife. Efron (1979)EFRON B. 1979. Bootstrap methods: another look at the jackknife. The Annals of Statistics 7: 1-26. summarized the methodologies based on resampling existing until then and established a new research area.
The idea of replacing complicated and often inaccurate simulation methods based on resampling approaches has attracted several researchers to develop methodologies based on bootstrap for various purposes. With the popularization of the bootstrap method, some researchers have begun to establish mathematical conditions under which the bootstrap is justifiable.
In the literature there are many jobs that make use of bootstrap methodologies. In general, the bootstrap method is used to correct the biases of the estimators, in construction of confidence intervals, hypothesis tests, estimation of journal errors of estimators, among others.
The bootstrap methods present two approaches, namely the parametric bootstrap and nonparametric bootstrap. Parametric bootstrap refers to the case where the resampling is performed based on a known or established distribution , where is an estimator of . On the other hand, in bootstrap nonparametric, there is the lack of a true distribution . The resampling is based on the empirical distribution function . Resample of is equivalent to resample data with replacement.
BOOTSTRAP PERCENTILE INTERVAL
Let be an estimator of the scale based on observations and its estimate. Let be the same estimator based on observations resampling from the original sample with replacement and its estimate. For simplicity, suppose is a continuous random variable. Denoting the th quantile of the distribution of the random variable by , we obtain
As the amount is invertible and depends only on the sample, we can construct the confidence interval for by rewriting the events above, i.e., we can replace the events and by and , respectively. Thus, the confidence interval of level is given by the limits
In many situations, we do not know the distribution of . In such cases, suppose that there is some transformation , such that has a symmetric distribution. Suppose also that we can obtain the confidence interval of level to . According to Davison and Hinkley (1997)DAVISON A AND HINKLEY D. 1997. Bootstrap methods and their application. Volume 1. Cambridge: Cambridge University Press., we can use bootstrapping to obtain an approximation of the distribution of using the distribution of . Thus, we estimate the quantile of by the -th ordered value of estimated by where is the number of bootstrap samples. Similarly, the quantile of can be estimated by the -th ordered value of . Let be the -th quantile of . Since has a symmetrical distribution, then also has a symmetrical distribution, as soon as it is true that . Based on the symmetry of , we have and . As and are quantiles of and we can obtain these quantiles, the lower and upper limits of the confidence intervals are given by and , respectively, which lead to the limits
whose transformation to are given by
Note that we do not know the transformation . The confidence interval of level for the parameter does not involve and it can be evaluated without knowledge of this transformation. The interval (8) is known as the bootstrap interval percentile. According to (Davison and Hinkley 1997, 203)DAVISON A AND HINKLEY D. 1997. Bootstrap methods and their application. Volume 1. Cambridge: Cambridge University Press., the percentile method can be applied to any statistic.
INTERVAL DOUBLE BOOTSTRAP PERCENTILE
When using the percentile method, we can obtain a coverage different than the desired level (). The interesting thing is that we can continue making use of bootstrap to correct this discrepancy. This fact reveals the flexibility of the bootstrap method.
The idea to obtain more accurate confidence intervals is to make use of diagrams double bootstrap, i.e., for each replica of the original bootstrap there will be conducted another bootstrap. Consider the situation where only a trust boundary is of interest and it is the upper limit with nominal confidence level , where
We ignore the errors of simulation, which is really evaluated is the confidence limit . The bias of the bootstrap percentile follows from the fact that , which implies
Davison and Hinkley (1997)DAVISON A AND HINKLEY D. 1997. Bootstrap methods and their application. Volume 1. Cambridge: Cambridge University Press. proposed to correct the bias by adding a correction to , However, an approach more successful is to adjust the index . Thus, we can replace by and estimate which the fitted value () must be used. Therefore, we obtain satisfying
Note that the solution depends on , i.e., . Since the distribution is unknown, we can estimate by . Let by a sample obtained randomly with replacement from and a new sample obtained by refitting with empirical distribution functions given by and , respectively. Let and be the statistics evaluated at and , where and are the estimates, respectively. We denote as the conditional probability in and as the conditional probability in . We can obtain using the bootstrap version of equation (9) given by
From the definition (10), a scheme involving a second level of bootstrap is given by
Davison and Hinkley (1997)DAVISON A AND HINKLEY D. 1997. Bootstrap methods and their application. Volume 1. Cambridge: Cambridge University Press. proved that the coverage is corrected for . For unilateral confidence limits, we have or . For the bilateral confidence interval, the coverage is corrected to .
In general, especially in non-parametric problems, the determination of (Equation 11) can not be done exactly. Thus, approximate methods must be used. A basic algorithm is given as follows. Suppose we have samples of and denote these estimates by , where is the -th empirical distribution function.
Set
The values can be determined by approximation. We generate samples of and for each of them we obtain the estimated values , for . So,
where is the indicator function for an event . The Monte Carlo version of (11) is given by
where is the quantile of . The simplest way to obtain is sorting the values , and setting . The -th quantile of is used to obtain the corrected quantile of . The double bootstrap percentile bilateral interval algorithm is presented below:
-
For a given sample (original sample) calculate the quantity ;
-
Generate samples and obtain , for ;
-
Generat new bootstrap samples for each of the samples in the previous step, and for each one, calculate , with ;
-
Determine
where is the indicator function; -
Order vector with positions and obtain the lower and upper quantile of , which are given, respectively, by and ;
-
Order values (i.e, ) and build the confidence interval for the original sample using quantile estimates evaluated in the previous step. Considering the values of the ordered statistics , for , the limits of the interval of level are given by
SIMULATIONS AND HARDWARE USED
The pso function is computationally intensive, especially when the objective function involves some data sets. This situation is common when the goal is to maximize a log-likelihood function. The problem arises with great intensity when we use the pso function iteratively as is the case of Monte Carlo simulations. The simulations presented are extremely itensive because they will be studied using bootstrap percentile simple and double (two levels of bootstrap) to obtain interval estimates for the NHG parameters. For the case of double bootstrap, each replica Monte Carlo will have a bootstrap and for each bootstrap will be conducted another bootstrap render the simulations impractical in some hardware.
The simulations are performed using the R language (version 3.2.0) in the hardware from the National Center for High Performance Processing of São Paulo, Brazil (CENAPAD-SP). The CENAPAD-SP provides a powerful computing environment, based on machines RISC (IBM) and Intel/Itanimum2 (SGI) with operating system based in Unix. The theoretical processing capacity of these two environments totals around 33 TFLOPS beyond of 250 TB external disk. Its use is measured in Service Units, what are accounted as users run commands and process jobs. In particular, the simulations performed make use of the SGI Altix ICE 8400 LX system installed in CENAPAD-SP that have 64 CPU’s and 384 cores Intel Xeon Six Core 5680 of 3.33GHz, 1152 GB of RAM and Infiniband interconnect. The theoretical processing capability of the system is approximately 5 TFLOPS.
The jobs that are submitted for processing on SGI Altix ICE 8400 LX are managed and have resources allocated by the PBSPro software (Altair Portable Batch System Professional 11.0.2), which also has the function of managing the queues of jobs submitted for processing in the cluster. The cluster runs the opreracional system SUSE Linux Enterprise Server 11 SP1 (x8664) kernel 2.6.32.13 64 bits. Access to the cluster is through a local machine using SSH (Secure Shell), which is part of the suite of protocols TCP/IP that makes secure remote administration of Unix-type servers.
It is possible to use in an efficient way all of the computing resources of SGI Altix ICE 8400 LX running parallel computing by OpenMPI (Message Passing Interface), which is nothing more than a journal for parallel computing in data communication. The use of journal OpenMPI in R is through the Rmpi SNOW doSNOW and foreach libraries available on the license terms GPL-2 (GNU General Public License).
This pattern allows using R by dividing each bootstrap in several cores of different nodes in the cluster. Thus, the code executes sequentially until it finds a parallel builder, i.e., there is a flow of execution main (master thread) and when required new threads are triggered to divide the work and, finally, it is performed a join for recovery the results. Divide each replica of bootstrap does not cause problems because each replica bootstrap is mathematically independent of another.
For the simulations we consider 10,000 replicas of Monte Carlo, and for each replica it is carried out two levels of bootstrap percentile. The first level of bootstrap is composed of 500 samples () and the second level is carried out 250 new samples (). The simulations are extremely intensive since for each Monte Carlo replica, we perform an optimization by PSO algorithm and within each replica of bootstrap (first level of bootstrap) another optimization is performed by PSO algorithm, closing a total of 5 million optimizations. Table I gives the times of the execution of R scripts, in hours, on hardware mentioned above. Note that for in the double bootstrap scheme, some simulations exceeded 11 days.
Times of executions of Monte Carlo simulations to evaluate the interval estimates obtained by single and double percentile bootstrap.
The performance of interval estimates is evaluated by percentile bootstrap and double percentile bootstrap at a nominal level of for different sample sizes ( and ). The real model has fixed parameters and .
For the first level of bootstrap, the journal errors of the MLEs are obtained by the PSO method. Small errors in the estimates are obtained for different sample sizes as shown in Figure 4. The performance of interval estimates by single and double bootstrap is assessed by the percentage of coverage, i.e., it is considered the percentage of confidence intervals containing the true parameter. It is noted that build interval estimates by single percentile bootstrap is not a good strategy for obtaining random intervals for the model parameters. In all cases, there are much lower coverages for the nominal levels. However, using the second level of bootstrap to correct the estimate obtained by simple percentile bootstrap method represents a good improvement in the interval estimates. Table II gives the improvement in coverage when using the double percentile bootstrap method. It is also possible to notice a small increase in the range of interval estimates by using the second level of bootstrap. Anyway, the small amplitudes do not represent a problem.
Errors evaluated by bootstrap and the maximum likelihood using the PSO method with 500 bootstrap replicates.
Coverage and range of interval estimates by simple bootstrap, double and nominal level of 95%.
EMPIRICAL EXAMPLE
For this application, we use a real data set referred to the times between failures (thousands of hours) of secondary reactor pumps. The data are presented in Table III. A descriptive analysis of the data is given in Table IV.
There are certainly other competitive distributions to fit these data. It is also reasonable to understand that generated distributions can be used in practice and depending on the problem a distribution will provide a better fit than others.
For this application, we consider the NHG(), NH() and ENH() models. The cdfs are given in Table III.
In order to determine the shape of the most appropriate hazard function for modeling, a graphical analysis data may be performed. In this context, the total time in test (TTT) plot proposed by Aarset (1987)AARSET MV. 1987. How to identify a bathtub hazard rate. IEEE T Reliab 36: 106-108. can be used. Let be a random variable with non-negative values, which represents the survival time. The TTT curve is obtained by constructing the plot of versus , for , where , are the order statistics of the sample [see (Mudholkar and Hutson 1996)MUDHOLKAR GS AND HUTSON AD. 1996. The exponentiated Weibull family: some properties and a flood data application. Commun Stat Theory Methods 25: 3059-3083.].
We use the R programming language version (3.0.2). In particular, we use the Adequacy Model package in version 1.0.8 available for download at http://cran.r-project.org/web/packages/AdequacyModel/index.htmlunder the terms of the GPL-2 and GPL-3. The script was created and is maintained by two authors of this paper. The plots can be easily obtained using the TTT function of the AdequacyModel. For more details on this function, see help(TTT). The TTT plot for the current data is displayed in Figure 5, which alternates between convex and concave, suggesting, according to Aarset (1987)AARSET MV. 1987. How to identify a bathtub hazard rate. IEEE T Reliab 36: 106-108., that the better risk function to the current data has decreasing form.
Figure 6 displays the estimated distributions to the data obtained in a nonparametric manner using kernel density estimation with the Gaussian filter. Let be independent and identically distributed observations, where each of them follows an unknown f distribution. The kernel density estimator is given by
where is the kernel function usually symmetrical, , and is a smoothing parameter known in the literature as bandwidth. Numerous kernel functions are available in the literature. The journal normal distribution is the most widely used because it has convenient mathematical properties. Silverman (1986)SILVERMAN BW. 1986. Density estimation for statistics and data analysis. Volume 26. CRC press. demonstrated that for the journal normal, the ideal bandwidth is , where is the journal deviation of the sample.
The TTT plot for the time between failures (thousands of hours) of secondary reactor pumps.
Gaussian kernel density estimation for the data of the time between failures (thousands of hours) of secondary reactor pumps.
The AdequacyModel package provides a computational support for researchers who want to work with continuos distributions, mainly in the survival analysis area. The AdequacyModel package is used to evaluate some adequacy statistics such as the AIC (Akaike Information Criterion), CAIC (Consistent Akaikes Information Criterion), BIC (Bayesian Information Criterion), HQIC (Hannan-Quinn information criterion), KS (Kolmogorov-Smirnov), (Anderson-Darling), (Camér-von Misses) and KS (Kolmogorov-Smirnov) statistics. The last two measures are described by Chen and Balakrishnan (1995)CHEN G AND BALAKRISHNAN N. 1995. A general purpose approximate goodness-of-fit test. J Qual Technol 27: 154-161.. The goodness.fit is the function in the AdequacyModel package used to evaluate these statistics. Other details can be obtained with the command help(goodness.fit).
Chen and Balakrishnan (1995)CHEN G AND BALAKRISHNAN N. 1995. A general purpose approximate goodness-of-fit test. J Qual Technol 27: 154-161. corrected statistics and based on the suggestions from Stephens (1986)STEPHENS MA. 1986. Tests based on EDF statistics. Goodness-of-Fit Techniques, RB D’Agostino and MS Stephens, Eds Marcel Dekker .. We use these statistics, where we have a random sample with empirical distribution function and want to test if the sample comes from a specified distribution. The statistics and are given by
respectively, where is the empirical distribution function, is the specified distribution function evaluated at the MLE of . Note that the statistics and are given by difference distances of and . Thus, the lower the statistics values the more evidence we have that generates the sample.
The R language is also used to obtain the MLEs by the PSO heuristic method of global optimization discussed in the previous section. Currently, the version 1.0.8 of the AdequacyModel package does not support the PSO methodology. However, possibly the journal algorithm or a modification will be present in future versions of the package. The journal errors of the MLEs of the model parameters are evaluated by non-parametric bootstrap. For the calculations, we consider 500 bootstrap samples () [see (Davison and Hinkley 1997)DAVISON A AND HINKLEY D. 1997. Bootstrap methods and their application. Volume 1. Cambridge: Cambridge University Press. and (Efron and Tibshirani 1993)EFRON B AND TIBSHIRANI R. 1993. An introduction to the bootstrap. Volume 57. New York: CRC press.].
Let be a random sample. Let also be the distribution function of the sample, where (unknown) is the true parameter and a estimator of . A bootstrap sample (non-parametric bootstrap) is obtained sampling with replacement from the original sample. The procedure generates a new sample (bootstrap sample). Let be the estimate of based on the bootstrap sample and be the fixed number of bootstrap samples. The bootstrap estimate of the journal error of is given by
where,
Table V presents the MLEs of the parameters of the distributions obtained by the PSO method. They are also given for the estimated journal errors obtained via nonparametric bootstrap. Also, we construct interval estimates by double percentile bootstrap for the model parameters. The estimates are presented in Table VI for a confidence level of 95%. We consider 500 samples for the first level and 250 bootstrap samples for the second level. Roughly speaking, all the competing models are able to fit the reactor pumps data. However, the adequacy statistics showed in Table VII indicate that the NHG model yields a better fit to these data than the other models.
The MLEs of the parameters for the fitted NHG, ENH, NH and EXP models through of the PSO method (bootstrap journal errors in parentheses).
Interval estimates by double percentile bootstrap for the model parameters at a confidence level of 95%.
Estimated for models fitted to the times between failures (thousands of hours) of secondary reactor pumps.
CONCLUDING REMARKS
We study the NHG distribution, which can be viewed as an improved extension of the Nadarajah-Haghighi (NH) distribution. The NHG density function can take various forms depending on its shape parameters. Moreover, its failure rate function can be decreasing, increasing, upside-down bathtub, bathtub-shaped, constant and decreasing-increasing-decreasing. Then, it can be used quite effectively in analyzing real data in practice. The estimation of the parameters is approached by the maximum likelihood method. The new distribution may attract wider applications for modeling failure times due to fatigue and lifetime data in fields such as engineering, finance, economics, and insurance, among several others. Two applications to real data illustrate the potentiality of the family. Future research could be addressed to study the complementary Nadarajah-Haghighi-geometric distribution. This class of distributions is a suitable model in a complementary risk problem based in the presence of latent risks, which arise in several areas such as public health, actuarial science, biomedical studies, demography and industrial reliability.
ACKNOWLEDGEMENTS
We would like to thank two referees for insightful comments and suggestions leading to improvements in the article. The financial support from the Brazilian governmental institutions Fundação de Amparo à Ciência e Tecnologia de Pernambuco (FACEPE) , Conselho Nacional de Desenvolvimento Científico e Tecnológico (CNPq) and Coordenação de Aperfeiçoamento de Pessoal de Nível Superior (CAPES) is gratefully acknowledged.
A Code in R language for the PSO method
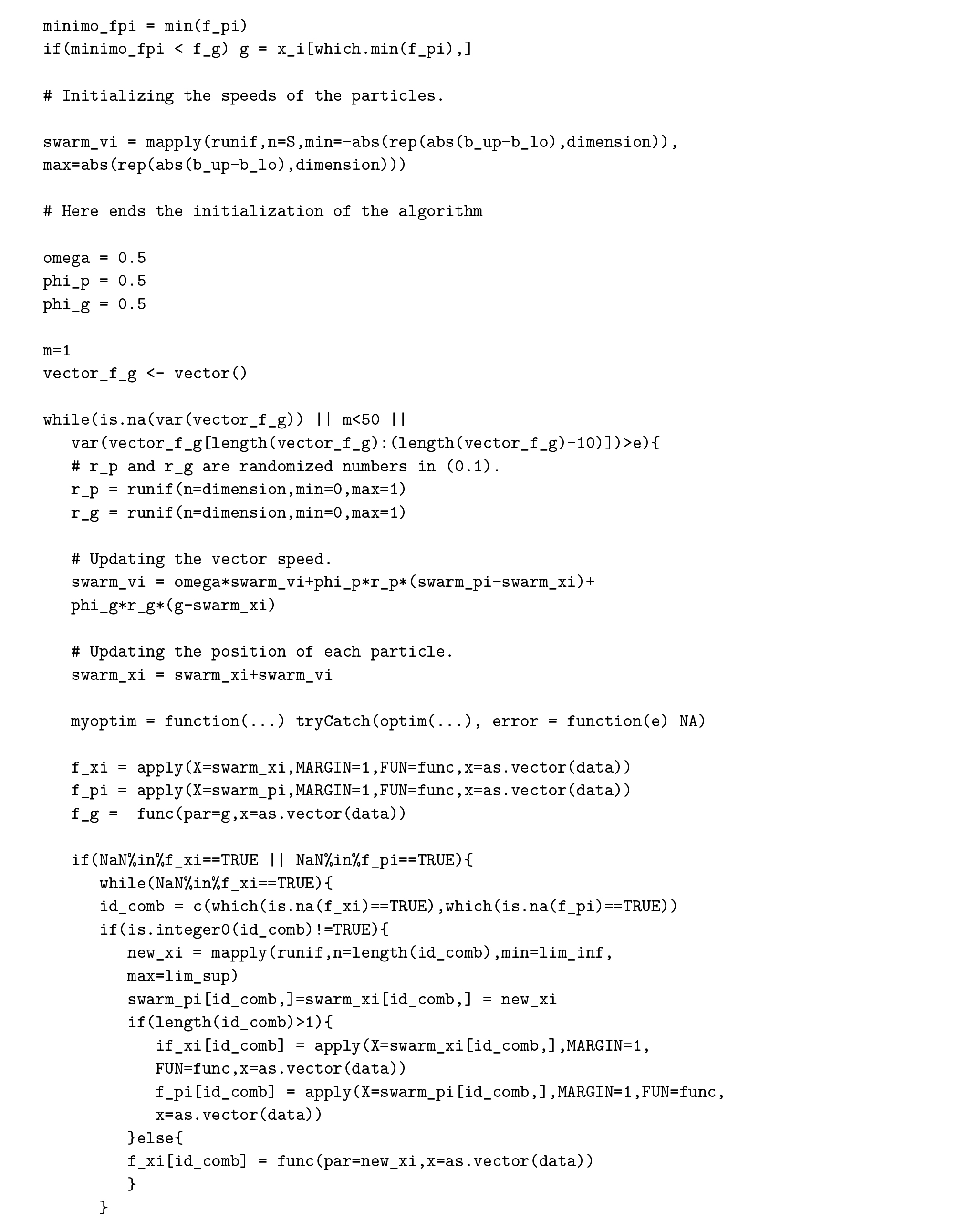
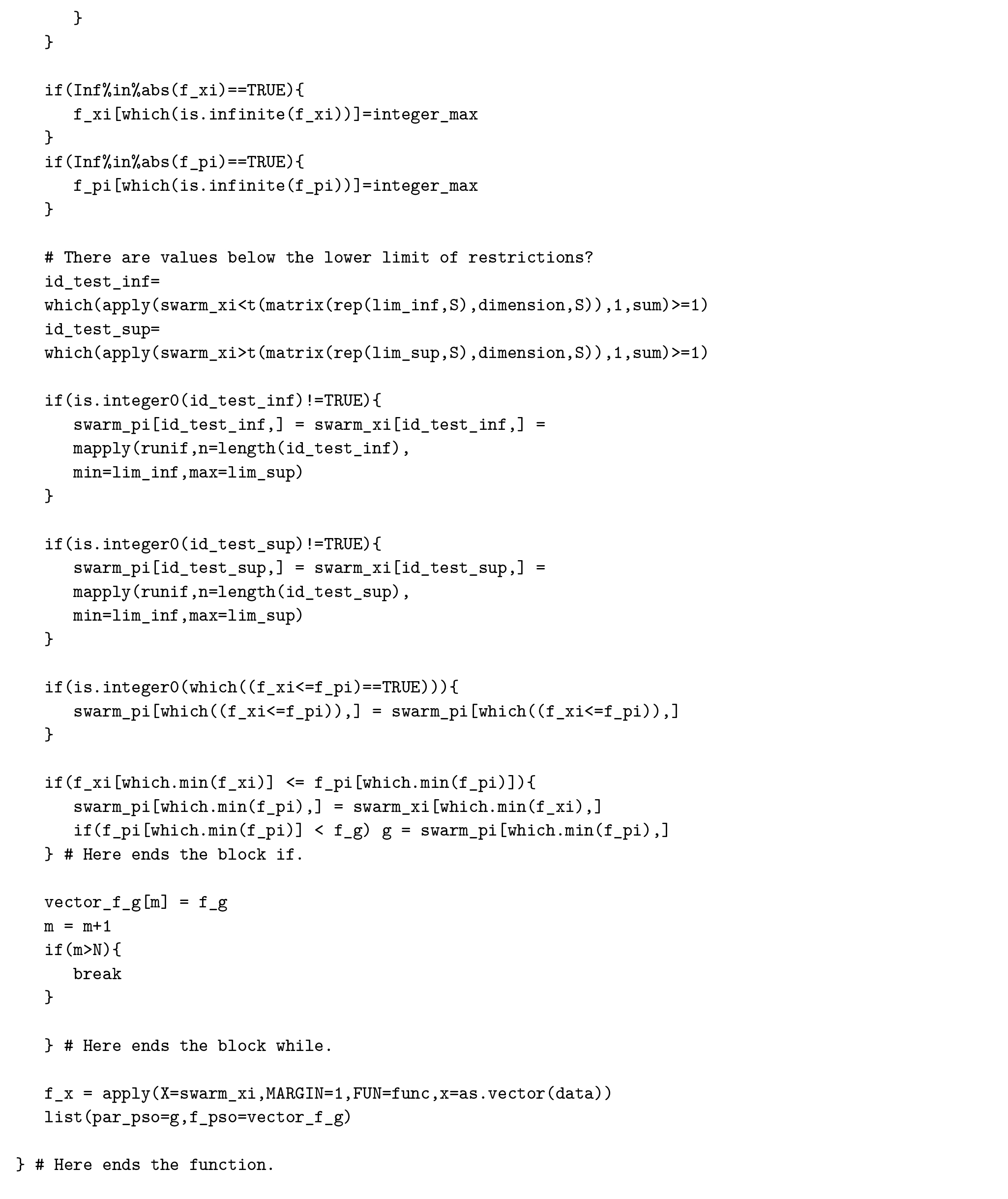
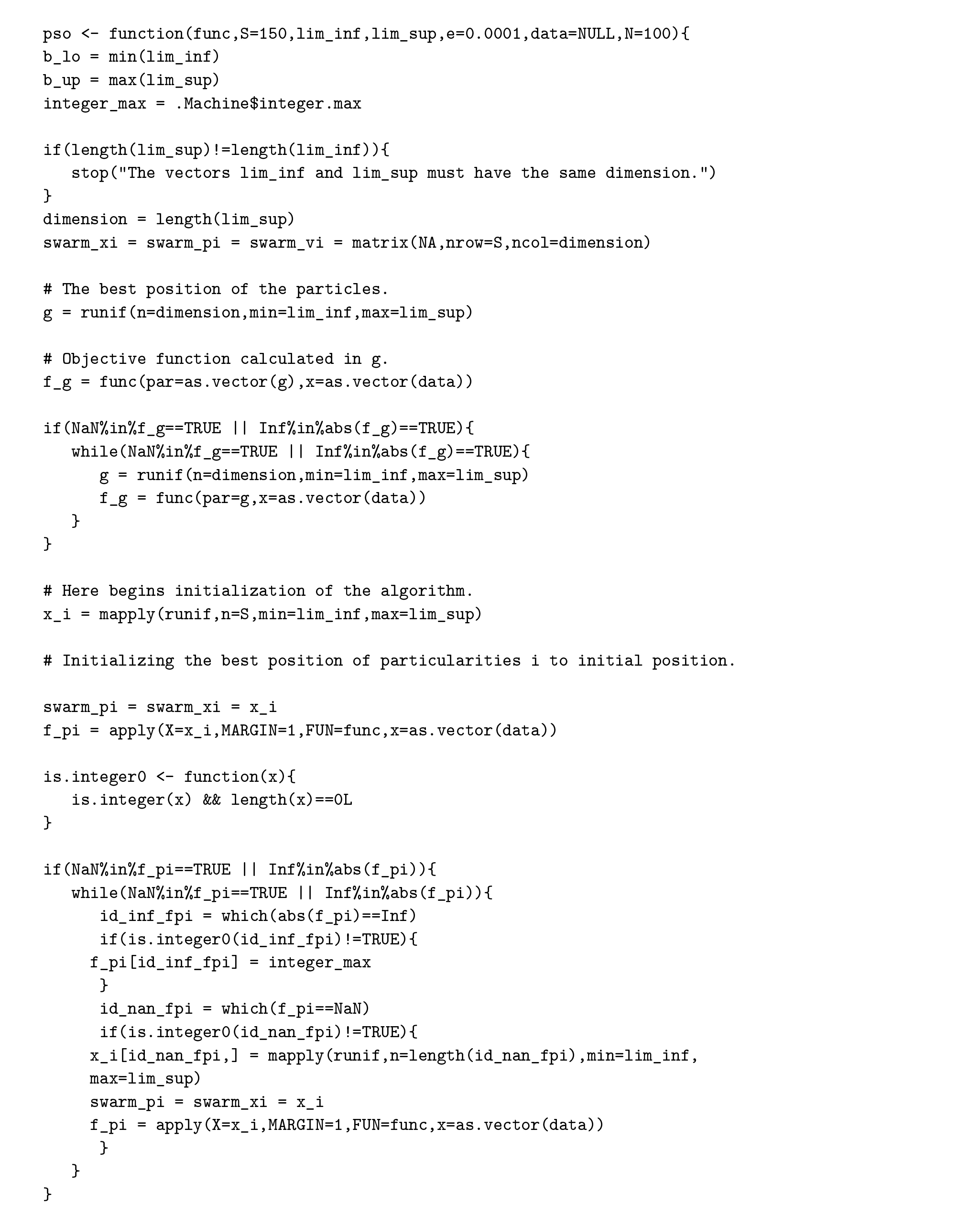
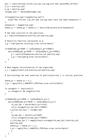
REFERENCES
- AARSET MV. 1987. How to identify a bathtub hazard rate. IEEE T Reliab 36: 106-108.
- ADAMIDIS K, DIMITRAKOPOULOU T AND LOUKAS S. 2005. On an extension of the exponential-geometric distribution. Stat Probabil Lett 73: 259-269.
- ADAMIDIS K AND LOUKAS S. 1998. A lifetime distribution with decreasing failure rate. Stat Probabil Lett 39: 35-42.
- BARRETO-SOUZA W, SANTOS AH AND CORDEIRO GM. 2010. The beta generalized exponential distribution. J Stat Comput Simul 80: 159-172.
- BASU AP AND KLEIN JP. 1982. Some recent results in competing risks theory. Lecture Notes-Monograph Series 2: 216-229.
- BOURGUIGNON M, SILVA RB AND CORDEIRO GM. 2014. A new class of fatigue life distributions. J Stat Comput Simul 84: 2619-2635.
- CHAHKANDI M AND GANJALI M. 2009. On some lifetime distributions with decreasing failure rate. Comput Stat Data Anal 53: 4433-4440.
- CHEN G AND BALAKRISHNAN N. 1995. A general purpose approximate goodness-of-fit test. J Qual Technol 27: 154-161.
- CORDEIRO GM AND DE CASTRO M. 2011. A new family of generalized distributions. J Stat Comput Simul 81: 883-898.
- CORDEIRO GM, ORTEGA EM AND NADARAJAH S. 2010. The Kumaraswamy Weibull distribution with application to failure data. J Franklin I 347: 1399-1429.
- DAVISON A AND HINKLEY D. 1997. Bootstrap methods and their application. Volume 1. Cambridge: Cambridge University Press.
- EFRON B. 1979. Bootstrap methods: another look at the jackknife. The Annals of Statistics 7: 1-26.
- EFRON B AND TIBSHIRANI R. 1993. An introduction to the bootstrap. Volume 57. New York: CRC press.
- EUGENE N, LEE C AND FAMOYE F. 2002. Beta-normal distribution and its applications. Commun Stat Theory Methods 31: 497-512.
- GOMES AE, DA-SILVA CQ, CORDEIRO GM AND ORTEGA EM. 2014. A new lifetime model: the Kumaraswamy generalized Rayleigh distribution. Journal of Statistical Computation and Simulation 84: 290-309.
- GUPTA RC, GUPTA PL AND GUPTA RD. 1998. Modeling failure time data by Lehman alternatives. Commun Stat Theory Methods 27(4): 887-904.
- KENNEDY J, KENNEDY JF AND EBERHART RC. 2001. Swarm intelligence. Morgan Kaufmann.
- LEE C, FAMOYE F AND OLUMOLADE O. 2007. Beta-Weibull distribution: some properties and applications to censored data. J Mod Appl Stat Methods 6: 17.
- MAHMOUDI E AND JAFARI AA. 2012. Generalized exponential-power series distributions. Comput Stat Data Anal 56: 4047-4066.
- MARSHALL AW AND OLKIN I. 1997a. A new method for adding a parameter to a family of distributions with application to the exponential and Weibull families. Biometrika 84: 641-652.
- MARSHALL AW AND OLKIN I. 1997b. A new method for adding a parameter to a family of distributions with application to the exponential and Weibull families. Biometrika 84: 641-652.
- MORAIS AL AND BARRETO-SOUZA W. 2011. A compound class of Weibull and power series distributions. Comput Stat Data Anal 55: 1410-1425.
- MUDHOLKAR GS AND HUTSON AD. 1996. The exponentiated Weibull family: some properties and a flood data application. Commun Stat Theory Methods 25: 3059-3083.
- NADARAJAH S AND HAGHIGHI F. 2011. An extension of the exponential distribution. Statistics 45: 543-558.
- NADARAJAH S AND KOTZ S. 2006. The exponentiated type distributions. Acta Applicandae Mathematica 92: 97-111.
- PRUDNIKOV P, BRYCHKOV A AND MARICHEV OI. 1986. Integrals and series: special functions. Volume 2. CRC Press.
- SILVA GO, ORTEGA EM AND CORDEIRO GM. 2010. The beta modified Weibull distribution. Lifetime Data Anal 16: 409-430.
- SILVA RB, BOURGUIGNON M, DIAS CR AND CORDEIRO GM. 2013. The compound class of extended Weibull power series distributions. Comput Stat Data Anal 58: 352-367.
- SILVA RB AND CORDEIRO GM. 2015. The Burr XII power series distributions: A new compounding family. Brazilian Journal of Probability and Statistics 29(3): 565-589.
- SILVERMAN BW. 1986. Density estimation for statistics and data analysis. Volume 26. CRC press.
- STEPHENS MA. 1986. Tests based on EDF statistics. Goodness-of-Fit Techniques, RB D’Agostino and MS Stephens, Eds Marcel Dekker .
Publication Dates
-
Publication in this collection
08 Apr 2019 -
Date of issue
2019
History
-
Received
15 May 2018 -
Accepted
30 July 2018