Abstracts
Price distributions forecast has become a relevant subject for risk and pricing literature. Special concern resides on tail probabilities, which usually present more severe observations than Normal distributions would predict. This work aims to verify ifthe volatility implied in real-dollar options contains useful information about unexpected large-magnitude returns. Implied volatility is also checked as a predictor for realized volatility. Our results indicate that implied volatilities provide useful information on unusual returns and also work as a good predictor for observed volatility. Moreover, we implement an early-warning system and implied volatilities seem to signalize large-magnitude returns.
Informational Content; Predictive Power; Early Warning
A previsibilidade das distribuições de preços de ativos financeiros consiste em tema relevante tanto na literatura de risco como de apreçamento de ativos. Interesse especial reside na investigação acerca das probabilidades dos eventos de cauda, cujas realizações apresentam impacto de maior severidade comparativamente ao previsto pela distribuição Normal. Este trabalho tem como objetivo verificar se as volatilidades implícitas de opções de dólar detêm conteúdo informacional e poder preditivo em relação a retornos de grande magnitude. Nossos resultados sugerem que essas expectativas de mercado, de fato, carregam informação relevante sobre retornos futuros, além de conter poder preditivo significativo.
Investigating unusual changes in real-dollar exchange rate* * The authors would like to thank the anonymous referee for his/her valuable comments. Needless to say, any remaining errors are ours. The views expressed in this paper are those of the authors and do not necessarily reflect those of the Banco Central do Brasil.
Frederico Pechir GomesI; Marcelo Yoshio TakamiII; Vinicius Ratton BrandiIII
IBanco Central do Brasil. E-mail: frederico.pechir@bcb.gov.br
IIBanco Central do Brasil. E-mail: marcelo.takami@bcb.gov.br
IIIBanco Central do Brasil. E-mail: vinicius.brandi@bcb.gov.br
ABSTRACT
Price distributions forecast has become a relevant subject for risk and pricing literature. Special concern resides on tail probabilities, which usually present more severe observations than Normal distributions would predict. This work aims to verify ifthe volatility implied in real-dollar options contains useful information about unexpected large-magnitude returns. Implied volatility is also checked as a predictor for realized volatility. Our results indicate that implied volatilities provide useful information on unusual returns and also work as a good predictor for observed volatility. Moreover, we implement an early-warning system and implied volatilities seem to signalize large-magnitude returns.
Keywords: Informational Content; Predictive Power; Early Warning.
JEL Code: D40;F31;G14.
RESUMO
A previsibilidade das distribuições de preços de ativos financeiros consiste em tema relevante tanto na literatura de risco como de apreçamento de ativos. Interesse especial reside na investigação acerca das probabilidades dos eventos de cauda, cujas realizações apresentam impacto de maior severidade comparativamente ao previsto pela distribuição Normal. Este trabalho tem como objetivo verificar se as volatilidades implícitas de opções de dólar detêm conteúdo informacional e poder preditivo em relação a retornos de grande magnitude. Nossos resultados sugerem que essas expectativas de mercado, de fato, carregam informação relevante sobre retornos futuros, além de conter poder preditivo significativo.
1. INTRODUCTION
Academics, regulators and market players all agree on the benefits from a stable financial system, capable of recognizing, measuring and controlling financial risks. As Pownall and Koedijk (1999) point out, several regulatory changes have been recently put into practice worldwide in order to achieve this goal. Their purpose is to outline the advantages associated with the use of risk management tools in financial systems, reducing the potential damages originated by banking crises and systemic shocks. The authors divide these regulatory changes in two categories: the minimum capital requirements for financial institutions, recommended by the Basel Committee,1 1 The Basel Committee on Banking Supervision, established at the end of1974, is composed ofmembers from Belgium, Canada, France, Germany, Italy, Japan, Luxembourg, the Netherlands, Spain, Sweden, Switzerland, United Kingdom and United States. The Committee encourages convergence towards common approaches and common standards regarding the supervision of financial systems. and the dissemination of risk culture among market participants, including the adoption of the widespread Value-at-Risk technique as a risk management tool.
Nevertheless, banking crises observed in recent years indicate that risk management tools were not able to assess the real magnitude of the risks present in turbulent periods. As stated by Blejer and Schumacher (1998), this might be related to the fact that most of the risk models were developed under naive modeling assumptions, such as the assumption of the normality of asset returns distribution. It is well known, however, that fat-tailness consists on a stylized fact of financial time series distributions. As a consequence, the probability of a negative result may be underestimated in the tail, where accuracy should be even more demanded, due to higher severity of the corresponding events.
The relevant issue is, therefore, how to overcome those drawbacks, in a way that extreme movements could be better modeled and tail bias avoided. Among the several techniques available, it is worth mentioning Stress Testing, which examines the effects on portfolios of huge and unusual movements (high severity and low frequency) in financial variables and is often dealt with the use of the results from the Extreme Value Theory (EVT). In this regard, it is worth mentioning the development of methodologies applied to the anticipation of crises and to the search for early warning signals is a fast-growing field of study.
In general, those techniques are focused on the analysis of macroeconomic variables. Berg and Pattillo (1998), Frankel and Rose (1996) and Kaminsky et al. (1998), among others, postulate that the monitoring of macroeconomic figures, such as current account balance, level of international reserves, debt to GDP ratio, can be useful when the purpose is to predict the occurrence of financial crises.
Alternatively, there has recently been a growing literature concerned on the information contained in market prices. In short, supported by market efficiency and completeness assumptions, some authors2 2 See Malz (2000) for more details. believe that asset prices provide relevant and valuable information about future prices behavior, especially in the near term. That is the case, for instance, of those who extract market information using the technique first presented by Breeden and Litzenberger (1978), implemented through the estimation of risk-neutral densities (RND). The authors state that in a risk-neutral environment one could imply a state-price density from options contracts that could be interpreted as the probability density over the underlying asset price. Craig and Keller (2004), for example, find that RND extracted from American-style options on foreign exchange function quite well as an estimator for the period over which the options are thickly traded. Moreover, they find that simple option valuation models fit the densities as well as the more sophisticated ones.
The purpose of the current work is to test one technique created to obtain, from the information contained in financial asset prices, useful information about unusual future price movements. More specifically, the aim is to verify if real-dollar options implied volatilities provide useful information about large-magnitude returns in the future. Besides testing this informational content, the implied volatilities' predictive power is also checked. As in Andrade and Tabak (2001), it is verified if the information about subsequent realized volatilitycontained inimplied volatilityoutperforms the information provided by past returns. Finally, as cited earlier, it is proposed a practical warning system to capture the informational content of implied volatilities in real-dollar options, as in Malz (2000).
The choice of implied volatility as the observed variable is based on earlier literature findings3 3 Christensen and Prabhala (1998), Jorion (1995) and Navatte and Villa (2000). characterizing this price as a predictor4 4 As noted by Ahoniemi (2006), predominant studies reject the hypothesis of an unbiased predictor. According to Neeley (2004), common answers for the bias may be overlapping data, the use of low frequency data or the non-pricing of volatility premia. See Doran and Ronn (2006) for further debate on the subject. for realized volatility. Besides, Malz (2000) suggests that the information contained in option prices may properly work as a signal for stress events once these instruments provide market participants with the possibility of hedging their positions against huge price changes.
The remaining part of this work is organized as follows. Section 2 describes the data used to implement the study and detail the methodology chosen to compute implied volatility. In Section 3, the informational content of implied volatilities is checked and the predictive power test is presented. In Section 4, a practical warning system is described and its results discussed. Finally, Section 5 presents our concluding remarks.
2. DATA DESCRIPTION
The real-dollar options are traded at Bolsa de Mercadorias e Futuros (BM&F), the Brazilian derivatives exchange.5 5 According to a report released in 2004 by the Futures Industry Association, BM&F is the 6 th derivatives exchange in the world when considered only the trading of futures contracts. They are European-style calls and the underlying asset is the foreign exchange spot rate (amount of BRL per USD 1,000). The contracts do not contemplate physical delivery since the Brazilian FX regulation prohibits the delivery of a foreign currency. Options on real-dollar futures are also traded at BM&F, but were not used in this study because of the lack of liquidity.
All the data are daily and cover the period from June 1st, 1999 to March 30th, 2008. The data from 1994 to 1999 were avoided due to the strict control the Brazilian Central Bank had over the foreign exchange market. After January, 1999, the Brazilian authorities decided to adopt a free float regime. In the case of the signaling test, presented in Section 4, it only begins on June 14th, 2000, approximately a year after the start date in June, 1999.
To obtain implied volatilities from the real-dollar calls, the following data are necessary: a) real-dollar futures (F), as the amount of BRL per USD 1,000 maturing on the same day the option expires; b) the strike exchange rate (K), also as the amount of BRL per USD1,000; c) number of business days as a fraction of a 252-day year (t); d) the continuously compounded domestic risk free interest rate (r), obtained from the DI futures contracts;6 6 Future contracts on the Brazilian inter-bank rate. Unlike what happens with interest rate futures in the US and Europe, where the underlying asset is a fixed income security maturing after the futures maturity, in Brazil our main interest rate future contract, DI futures, may be considered similar to a fixed income security negotiated in the spot market with daily adjustments (mark to market). and e) the price of the last traded call (c).
Due to differences in maturities and strike prices, several calls on real-dollar exchange rate are traded on a daily basis at BM&F. As a result, different implied volatilities are obtained for the same underlying asset. However, it is well known that, for a given asset, only one volatility applies. In order to solve this problem, Lemgruber (1995) recommends 2 alternatives: (i) to calculate a weighted-average implied volatility; and (ii) to use the implied volatility associated with the at-the-money (ATM) option. As suggested by Beckers (1981), ATM options are better than any other approaches based on weighted-averages. Additionally, these options are usually the most traded instruments, best reproducing market expectations. For that reason, Jorion (1995) chooses the ATM calls and puts to compute implied volatilities. However, considering that put options are very illiquid instruments in the Brazilian derivatives market, we decided to use solely call options.
The analysis here discussed was implemented based on three different estimates for the implied volatility: (i) the average of the daily implied volatilities weighted by the number of trades; (ii) the average of the daily implied volatilities weighted by each option's gamma, defined as the sensitivity of delta to the underlying asset price changes; and (iii) the implied volatility associated with the ATM option.7 7 The ATM option here is the one with the present value of K closest to the spot price ( S).
Some filters were included before the calculation of the implied volatilities. Traded calls with time to maturity inferior to 6 business days were eliminated in order to avoid distortions in volatility, as indicated by Malz (2000). Another reason is documented in Matos et al. (2004), where they show that Brazilian typical real-dollar options behave like Asian options,8 8 Asian options have their payoff dependent on the average price of the underlying asset during a predefined period. as maturity gets closer. Such particular feature arises due to contract specifications, because the underlying asset is referred to an official exchange rate computed by the Brazilian Central Bank as a weighted-average of market prices on actual trades - PTAX 800.
Besides, the analysis was restricted to the more liquid series. Because the series with longer maturities are very illiquid, only the two first maturities were used in the calculation of the implied volatilities chosen to perform the informational content test. In order to perform the predictive power test, only the first maturity was considered.
With the data filtered, the implied volatility was calculated based on the Garman and Kohlhagen (1983) formula, as shown in Section 2.1. Once the implied volatilities were obtained, the negative ones were eliminated, given the fact that this would represent an arbitrage opportunity. With the remaining data, the calculation of the daily average is implemented. The gamma (Γ) used as a weighting factor was obtained through the use of the following formula for a European-style call:
with
and
From November 1st, 1999 to March 24st, 2008,2,044 implied volatilities on real-dollar options were obtained and used to perform the informational content, the predictive power and the signaling tests. Additional data were used only for estimation purposes. It is important to notice that the underlying asset is traded during some days when the derivatives market is closed (e.g. December, 31st).
2.1. Computing Implied Volatilities
After the original derivation of Black and Scholes (1972), Merton (1973) was the first one to derive a formula to value European options on assets paying a continuous dividend yield. Assuming that currency positions provide yields similar to dividends, equal to the risk-free rate in the foreign currency, Garman and Kohlhagen (1983) show that the same formula can be used in the currency option market. Using analogous rationale, Black (1976) demonstrates that future prices present a stochastic behavior similar to stocks paying a continuous dividend yield and derives a pricing formula for options on currency futures. Hull (2003) shows that futures and spot options prices with similar features should be equally priced whenever future options mature at the same time as its underlying future contract.
Despite comprising many simplistic assumptions about model's parameters, those B&S-based pricing models are still very popular among practitioners. Nevertheless, the literature has been generalizing some of them and more recent works have suggested alternative sophisticated modeling with the aim to incorporate more realistic assumptions.
Hilliard et al. (1991) model foreign and domestic interest rates following Vasicek (1977) and present an alternative approach to assess future prices volatilities. Their results indicate greater efficiency when compared to a constant dividend yield model. Cunha Jr. and Lemgruber (2003) apply similar methodology to the Brazilian currency options market and find that their results constitute an evidence that the proposed modeling provides better adjustment to market prices than those of traditional fixed dividend yield approaches.
Hull and White (1987), Scott (1987) and Wiggins (1987) are examples of works that have addressed the valuation of options on assets presenting stochastic volatility.9 9 Jorion (1995) reminds that if volatility is indeed stochastic, the arbitrage argument behind B&S will not stand and, therefore, B&S option pricing model shall be considered inconsistent. Duan (1995), in the same line, derived an option model where the price returns follow a GARCH diffusion process. Melino and Turnbull (1991) examine currency options on G-7 exchange rates and conclude that stochastic volatility models are best fitted to market prices than B&S with time series parameters estimators. It is worth to mention that Chesney Marc (1989), however, develop a similar analysis using implied volatilities instead of historical ones. His results lead to an opposite conclusion, with a worse fit provided by stochastic volatility models.
The approaches above mentioned involve high computational costs related to the numerical solution of partial differential equations. For that reason, Stein and Stein (1991) and Heston (1993) addressed the problem proposing the use of analytical answers. Da Costa and Yoshino (2004) test the adequacy of the Heston (1993) model for the Brazilian currency options market. The analytical formula is calibrated through the minimization of the quadratic error when compared to market prices. The authors suggest that the simplest models may work very well for ATM options. For deep-out-of-the-money options, they say, even the Heston (1993) sophisticated approach fails to explain market prices.
In this work, we use Black (1976) model for European options on futures, which may be similar to Garman and Kohlhagen (1983) whenever future values are priced at their fair values. The implied volatilities are obtained by making market prices equal to the one obtained by using the option pricing formula:
where:
F is the future price;
K is the strike price;
t is the time to option expiration;
r is the risk free rate, and;
σ is the asset volatility.
3. INFORMATIONAL CONTENT AND FORECASTING ACCURACY
3.1. Informational Content
One of the most preliminary investigations between realized volatility and their expectations measured through options contracts is classified by Jorion (1995) as informational content analysis. Following Day and Lewis (1992), the author regress one-week-ahead realized volatility in the foreign exchange market against implied volatility. It is important to notice that, since the maturities of both volatilities mismatch, the slope coefficient will not necessarily indicate forecasting accuracy. Though, whenever it indicates positive significance, this will suggest that option prices may carry relevant and useful information about future volatilities.
In this article we test for the presence of informational content on the implied volatility against two other competing volatilities: TGARCH(1,1)10 10 Threshold GARCH. Please refer to Glosten et al. (1993) for further details. and the sample standard deviation. The standard deviation11 11 Sample standard deviation of the previous 21 daily log-returns. is the benchmark for a very simple and naive forecasting model and the in-sample TGARCH(1,1) model is considered to be a much more sophisticated time series model that provides a better fit to the data and also account for the presence of asymmetric impacts on conditional volatility. The TGARCH(1,1) model used is:
where Rt is the daily log-return,
Table 1 presents the results of TGARCH (1,1) estimation for the period from 06-01-1999 to 03-242008. Standard errors are shown in parenthesis. We see that the process is stationary and shocks are highly persistent. Also, as the gamma is negative, positive innovations (et > 0) are meant to have a larger impact on return's volatility.
Then, according to Andrade and Tabak (2001), we aplly the informational content as follows:
where:
Rt+1 is the one-day-ahead log-return;
is the standard deviation estimate;
is the conditional standard deviation TGARCH(1,1);
is the implied volatility;
is the estimated residual12
12
accounts for the TGARCH(1,1)-volatility's movements not explained by the implied-volatility's.
of the GMM regression
;
is the estimated residual ofthe GMM regression
;
is the estimated residual of the GMM regression
and;
is the estimated residual of the GMM regression
.
The previous regressions were applied by means of the GMM (Generalized Method of Moments). It provides a robust estimator in that it does not require information of the exact distribution of the disturbances and was used essentially for: i) identification problem13
13
One or more explanatory variables may be correlated to the disturbance and this results in biased estimators.
treatment and ii) heteroskedasticity and autocorrelation consistent covariance estimation. The GMM estimator selects parameter estimates so that the correlations between the instruments and disturbances are as close to zero as possible, i.e., the moment conditions are expressed by , where
. Furthermore, by choosing the weighting matrix in the criterion function appropriately, GMM can be made robust to heteroskedasticity and/or autocorrelation of unknown form. We used the first lag of each explanatory variable as instrumental variables.
Table 2 presents the coefficient estimates for equations (8) through (11) using the ATM (at-the-money) estimation of implied volatilities (IV). The results are non-conclusive regarding the explanatory power, i.e., they indicate that the explanatory power of the implied volatilities are not superseded by and do not supersede the standard deviation estimate's or the conditional standard deviation TGARCH(1,1)'s.
Moreover, we aim to assess the informational content of implied volatilities based on Malz (2000) approach, which applies Granger causality test in order to capture lagged relationship higher than one-day length. Despite Granger causality does not provide a notion of causality in an economic sense, it may be very useful to indicate the lead-lag relationship between two variables. We determined maximum length of 10 business days and the optimal lag length is chosen by AIC (Akaike Information Criteria) using univariate VAR (Vector Auto Regressive) model. We obtained a lag equal to 8 business days for every regression, which is close to the lag of 5 arbitrarily chosen by Malz (2000), assuming that price adjustments occur within one trading week.
The Granger causality test basically verifies whether the conditional forecast variance of a dependent variable can be reduced significantly by including past information of another variable in the equation along lagged values of the dependent one itself. As shown in Greene (2003), tests comparing restricted and unrestricted equations can be based on a simple F test in the single equations ofthe VAR model.
The restricted equation is defined as below, comprising only lagged values of the dependent variable. The use of squared returns, as proposed by Malz (2000), is intended to capture large magnitude returns, focusing on kurtosis rather than skewness of the return distribution:
The unrestricted equation is defined as:
The test statistic is defined as follows:
where ut and vt are the residuals from the unrestricted and restricted regressions, respectively and T is the sample size. The statistic λ has an asymptotic F (k, T 2k 1) distribution. If the critical value of the F distribution for a specified confidence level is lower than λ the test will reject the null hypothesis stating that the new independent variable included in the unrestricted regression fails to Granger cause the dependent variable.
Table 3 contains the results for eq.(12) and eq.(13) comparison. In all the three cases, Granger causality is verified at a very low significance level, corroborating previous results on one-day ahead regressions which suggest that implied volatilities provide useful information on the prediction of future squared log-returns. The adjusted R-squared, also displayed in the table, indicate that previous squared returns in conjunction with implied volatilities estimates present significant explanatory power on future squared returns
Another test is proposed in order to identify the additional value of implied volatilities over historical TGARCH estimates. The unrestricted equation includes both TGARCH (1,1) and IV estimates as endogenous variables in the VAR model, along with the squared return:
Restricted equation is defined as follows:
Table 4 contains the results for (15) and (16) comparison. Granger causality is also verified at a very low significance level, in line with previous results, corroborating that implied volatilities provide additional valuable information rather than time series estimates. The adjusted R-squared ofunrestricted regressions indicates the significance of the variables and the expected increase in the explanatory power when compared to the above-mentioned results.
3.2. Predictive Power
Initially, we applied the same approach used by Jorion (1995), in which the realized volatility is regressed against the ATM implied volatility (17) and against the average expected TGARCH(1,1) (18):
where σ t,T is the realized volatility between t and the expiration date T, measured as the standard deviation of daily returns σ IV,t is the implied volatility in date t for the period between t and T and TGARCHt is the average expected TGARCH(1,1).
To compute the forecast of the average conditional variance , we followed the derivation in Heynen et al. (1994), which derives average expected GARCH(1,1) volatilities differing in times to maturity (see the Appendix A
APPENDIX A
):
The forecasting series is then computed from the parameters estimation of the in-sample TGARCH(1,1) used in the previous section. The first lag of the explanatory variable is used as instrumental variable and the results of the GMM regressions (17) and (18) are presented in Table 5.
The results of Table 5 show that there is no evidence of superiority of one competing volatility against the other. Therefore, we applied an encompassing test likewise the approach undertaken in the information content analysis. Now, the aim is to compare the predictive performance of the implied volatility only against TGARCH(1,1) taking the realized volatility as the dependent variable:
where is the estimated residual of the GMM regression
and
is the estimated residual of the GMM regression
.
We used the first lag of each explanatory variable as instrumental variables and the results of the GMM regressions (20) and (21) are presented in Table 6. The ATM implied volatility is found to be an efficient volatility predictor at the 5% significance level, i.e., one cannot reject that the ATM implied volatility may contain incremental information regarding the TGARCH(1,1), as α1 and γ2 are significantly different from zero at the 5% significance level, while a2 is non-significant.
3.3. Sub-sample Analysis
As a test of robustness, we divided the entire sample into two sub-samples and performed the informational content and predictive power analysis on each one. Sub-samples were established based on the dynamics of real-dollar exchange rate, which was highly influenced by the presidential election turbulence in Brazil during the second semester of 2002: i) rising exchange rate level (from November 1999 to October 2002) and ii) decreasing exchange rate level (from November 2002 to March 2008).
As a whole, the results did not change significantly. For the second sub-sample, which comprises more than a half of the entire sample, results are completely robust, as they lead to the same conclusions. The results for the first sub-sample, however, are not conclusive with regards to the predictive efficiency, i.e., one cannot affirm that the ATM implied volatility contains incremental information when compared to the TGARCH(1,1), and vice-versa.14 14 In our view, the lack of predictive efficiency in the first sub-period (from November 1999 to October 2002) may be due to a market-learning process, as the floating exchange rate regime had been implemented a couple of months before (February 1999).
4. SIGNALING FOR TAIL EVENTS
In the previous sections we have shown that implied volatility on real-dollar options contains relevant information about future large-magnitude returns. From now on, our intention is to use this information to build a practical tool that may work as a warning system for stress events.
The goal here is to follow Malz (2000) and measure the probability of a week-ahead large movement in real-dollar exchange rate conditional to observed high and rising implied volatility, and then verify whether or not there is independence between signal and future event.
The signaling tool consists, basically, of verifying the independence between two distinct events. The first one, which determines the subset of weeks A, is related to the occurrence of high and rising implied volatility in the one week. Once it is verified the occurrence of this event, a signal is considered to have been sent. The second event, which determines the subset B, refers to the occurrence of high absolute returns of the underlying asset (real-dollar exchange rate) one week ahead.
Because of the results presented in the predictive power section, where ATM options implied volatilities are highly superior in terms of R-squared when compared to the other estimates of implied volatility, the signaling test is performed based only on the ATM estimate.
The signaling is tested always based on weekly data observed every Wednesday,15 15 In the case the market is closed on Wednesday, data from Tuesdays are used. as suggested by Malz (2000) in order to avoid the day-of-the-week bias. Therefore, to define a high implied volatility, a series of daily implied volatilities is used to calculate, for each day, the average and the standard deviation of the previous 252 observations. If the estimate for a specific Wednesday is one standard deviation higher than the average, both computed for that specific date, the implied volatility is considered to be high.
To obtain a rising implied volatility it was necessary to compute log variations of implied volatilities between Wednesdays. Those values are then compared to the product of 0.674516 16 Representing the 75 th percentile of the standard Normal distribution. and the standard deviation of last 252 daily implied volatility log variations, scaled up to week dimension through the square root rule. This last standard deviation encompasses the concept of vol of vol (volatility of volatility), which in certain way reveals the magnitude of the variability of the daily implied volatility estimations in recent observations. If the implied volatility variation calculated from Wednesday to Wednesday is higher than this last product, it is considered to be rising.
After that, weekly returns on the underlying asset, measured between Wednesdays, are considered to be high whenever its absolute value is higher than 2.33 (99th percentile) times the standard deviation of the last 252 daily log returns, scaled up into week dimension by the squared root rule.
Independence is verified through Chi-square and Fisher's Exact tests based on the 2x2 contingency table presented below. Both of them tests for the null hypothesis of independence between events A and B. Therefore, the rejection of the null hypothesis lead to the interpretation that the implied volatility does provide a good warning signal for large magnitude returns.
The test statistic of the chi-square test has an asymptotic chi-square distribution with 1 degree of freedom and is obtained as follows:
with Njk as the number of elements in the jkth cell and with the dots indicating totals of columns and cells. The value for the above contingency table is 12.46, with an associated p-value of 0.042%, suggesting that implied volatility signals for real-dollar large magnitude returns at a 99.38% confidence level.
Fisher (1922) has shown that Chi-square test, due to its asymptotic feature, provides inaccurate results when the expected numbers for the contingency table are small. Alternatively, the author recommends the application ofa hypergeometric test, which p-value associated with the null hypothesis of independence is given by the following hypergeometric probability function:
Accordingly, as mentioned before, we have also performed the Fisher's exact test for independence and with a p-value of 0.326% the test rejects the null hypothesis of independence at the 1% significance level.
Robustness is checked by varying the thresholds used to identify high returns and high and rising implied volatilities. Originally, Malz (2000) used 2.33 standard deviations for high returns, and 1.00 and 0.6745 standard deviations for high and rising implied volatilities, respectively. Here, we use different thresholds ranging from 0.6745 to 2.33. Generally, the null hypothesis cannot be rejected whenever low thresholds (0.6745 and 1.00) are used to identify high returns.
4.1. Controlling for Serial Correlation
Notwithstanding these results, we share Malz (2000) concerns that the tests performed earlier in this section have major limitations when applied to financial time series. Data sample frequency and volatility clustering, which are broadly acknowledged stylized facts for financial returns, may bias the sample or suggest for spurious relationship. Hence, although we interpret our results as strong evidence for market signaling, we understand that further development on the implementation of signaling tools in financial markets must be done.
Therefore, we propose an improvement in Malz's methodology by implementing a small change in the second event of the signaling tool, which determines the subset B of weeks. Now, this subset does not only refer to the occurrence of high absolute returns but also the occurrence of rising returns one week ahead. We understand that this modification can control for serial correlation in the sense that subsequent returns ofthe same magnitude will never represent a signaling event.
Rising returns are those that present an absolute difference from last absolute return higher than 0.6745 multiplied by the standard deviation of the last 252 daily log returns, scaled-up to week dimension through the square root rule. P-values obtained from the Chi-square and Fisher's exact tests are 0.396% and 1.241%, respectively, corroborating previous results that implied volatility signals for large magnitude returns. Robustness is also checked as before and, overall, the null hypothesis cannot be rejected whenever very high thresholds (2.33) are used to identify high implied volatilities.
5. CONCLUDING REMARKS
The purpose of the current work is to test one of the techniques created to obtain, from the information contained in financial asset prices, useful information about unusual future price movements. Its specific aim is to verify if real-dollar options' implied volatilities can provide useful information about large-magnitude returns in the future. Besides testing this informational content, the implied volatilities' predictive power is also checked. Finally, it is proposed, based on Malz (2000), a practical warning system to capture the informational content of implied volatilities in real-dollar options.
With regards to the informational content analysis, Granger causality is verified at a very low significance level, corroborating previous results on one-day ahead regressions which suggests that implied volatilities provide useful information about large-magnitude returns in the future. In the case of the predictive power test, all implied volatilities measures are found to be efficient volatility predictors at the 1% significance level. This result demonstrates the capability of implied volatilities to predict future realized price variations on the underlying assets.
Finally, the signaling test indicates that the monitoring of implied volatilities on real-dollar options can be used to build an efficient and practical warning system for stress events in the future.
Bibliography
Ahoniemi, K. (2006). Modeling and forecasting implied volatility: An econometric analysis of the VIX index. Helsinki School of Economics, Discussion Paper 129.
Amin, K. & Jarrow, R. A. (1991). Pricing foreign currency options under stochastic interest rates. Journal of International Money and Finance, 10:310-329.
Andrade, S. C. & Tabak, B. M. (2001). Is it worth tracking dollar/real implied volatility? Revista de Economia Aplicada, 5(3).
Becker, J. L. & Lemgruber, E. F. (1989). Uma análise de estratégias de negociação no mercado brasileiro de opções: Evidências a partir das opções de compras mais negociadas durante o Plano Cruzado. In Brito, editor, Gestão de Investimentos. Editora Atlas.
Beckers, S. (1981). Standard deviations implied in options prices as predictors of future stock price volatility. Journal of Banking and Finance, 5:363-81.
Berg, A. & Pattillo, C. (1998). Are currency crises predictable? A test. IMF Working Paper Series. n. 98/154.
Black, F. (1976). The pricing of commodity contracts. Journal of Financial Economics, 4:167-179.
Black, F. & Scholes, M. (1972). The valuation of option contracts and a test of market efficiency. Journal ofFinance, 27.
Blejer, M. I. & Schumacher, L. (1998). Central bank vulnerability and the credibility of commitments: A value-at-risk approach to currency crises. IMF Working Paper Series, N. 98/65.
Breeden, D. T. & Litzenberger, R. H. (1978). Prices ofstate contingent claims implicit in option prices. Journal ofBusiness, 51:621-652.
Castro, P. C. (2002). Opções sobre dólar comercial e expectativas a respeito do comportamento da taxa de câmbio. Banco Central do Brasil, Brasília.
Chesney Marc, S. L. (1989). Pricing european currency options: A comparison of the modified Black-Scholes model and a random variance model. Journal ofFinancial and Quantitative Analysis.
Christensen, B. & Prabhala, N. R. J. (1998). The relation between implied and realized volatility. Journal of Financial Economics, 50(3):125-150.
Craig, B. R. & Keller, J. G. (2004). The forecast ability of risk-neutral densities of foreign exchange. Federal Reserve Bank of Cleveland, WP 04-09.
Cunha Jr., D. & Lemgruber, E. F. (2003). Opções de dólar no brasil com taxas de juro e de cupom estocásticos. III Encontro Brasileiro de Finanças. FEA, USP.
Da Costa, M. N. & Yoshino, J. A. (2004). Calibração do modelo de heston para o mercado brasileiro. IV Encontro Brasileiro de Finanças.
Day, T. & Lewis, C. (1992). Stock market volatility and the information content of stock index options. Journal ofEconometrics, 52:267-287.
Dickey, D. A. & Fuller, W. A. (1979). Distribution of the estimators for autoregressive time series with a unit root. Journal ofthe American Statistical Association, 74:427-431.
Doran, J. S. & Ronn, E. I. (2006). The bias in Black-Scholes/Black implied volatility: An analysis ofequity and energy markets. Florida State University Working Paper.
Duan, J. (1995). The GARCH option pricing model. Mathematical Finance, 5(1):13-32.
Feiger, G. & Jacquillat, B. (1979). Currency option bonds, puts and calls on spot exchange and the hedging of contingent foreign earnings. Journal of Finance, 34:1129-139.
Fisher, R. A. (1922). On the interpretation of χ2 from contingency tables, and the calculation of P. Journal of the Royal Statistical Society, 85(1):87-94.
Frankel, J. A. & Rose, A. K. (1996). Currency crashes in emerging markets: An empirical treatment. Journal of International Economics, 41:351-366.
Garman, M. & Kohlhagen, S. (1983). Foreign currency options values. Journal of International Money and Finance, 2:231-237.
Glosten, L. R., Jagannathan, R., & Runkle, D. (1993). On the relation between the expected value and the volatility of the nominal excess return on stocks. Journal of Finance, 48:1779-1801.
Gomes, F. P. (2002). Volatilidade implícita antecipada de eventos de stress: Um teste para o mercado brasileiro. Departamento de Estudos e Pesquisas, Banco Central do Brasil, Brasília.
Grabbe, J. O. (1983). The pricing of call and put options on foreign exchange. Journal of International Money and Finance, 2:239-253.
Granger, C. W. J. (1969). Investigating causal relations byeconometric methods and cross-spectral methods. Econometrica, 34:424-438.
Greene, W. H. (2003). Econometric Analysis. Prentice Hall, 5th edition.
Heston, S. (1993). Aclosed-form solutionfor options with stochastic volatilitywith applications to bond and currency options. The Review ofFinancial Studies, 6(2).
Heynen, R., Kemna, A., & Vorst, T. (1994). Analysis ofthe term structure ofimplies volatilities. Journal ofFinancial Quantitative Analysis, 29:31-46.
Hilliard, J. E., Madura, J., & Tucker, A. L. (1991). Currency option pricing with stochastic domestic and foreign interest rates. Journal ofFinancial and Quantitative Analysis, 26(2):139-151.
Hull, J. (2003). Options, Futures and Other Derivatives Securities. Prentice Hall, New Jersey, 5 edition.
Hull, J. & White, A. (1987). The pricing ofoptions on assets with stochastic volatilities. JournalofFinance, 42:281-300.
Jorion, P. (1995). Predicting volatility in the foreign exchange market. The Journal ofFinance, 50(2):507-528.
Kaminsky, G., Lizondo, S., & Reinhart, C. (1998). Leading indicators ofcurrency crises. IMFStaffPapers,45(1):01-48.
Lemgruber, E. F. (1995). Avaliação de Contratos de Opções. BM&F, São Paulo, revisada e ampliada edition. Malz, A. M. (2000). Do implied volatilities provide early warning ofmarket stress? RiskMetrics Journal, 1(1):41-60.
Matos, J. A., Kapotas, J. C., & Schirmer, P. P. (2004). Pricing and hedging brazilian currency options. IV EBF.
Melino, A. & Turnbull, S. M. (1991). The pricing of foreign currency options. Canadian Journal ofEco-nomics,Canadian Economics Association, 24(2):251-81.
Merton, R. (1973). Theory ofrational option pricing. Bell Journal ofEconomics, 4:141-183.
Navatte, P. & Villa, C. (2000). The information content of implied volatility, skewness and kurtosis: Empirical evidence from long-term CAC 40 options. European Financial Management, 6(1):41-56.
Neeley, C. (2004). Forecasting foreign exchange volatility: Why is implied volatility biased and inefficient? And does it matter? Federal Reserve Bank ofSt. Louis Working Paper.
Pownall, R. A. J. & Koedijk, K. G. (1999). Capturing downside risk in financial markets: The case of the Asian crisis. Journal ofInterantional Moneyand Finance, 18:853-870.
Scott, L. (1992). The information content of prices in derivative security markets. IMF StaffPapers,39:596-625.
Scott, L. O. (1987). Option pricing when the variance changes randomly: Theory, estimators, and applications. Journal ofFinance and Quantitative Analysis, 22:419-438.
Scott, L. O. (1991). Random variance option pricing. Advances in Futures and Options Research, 5:113-135.
Stein, E.M. & Stein, J. C. (1991). Stock price distributions with stochastic volatility. The Review of Financial Studies, 4(4).
Vasicek, O. (1977). An equilibrium characterization of the term structure. Journal of Financial Economics, 5:177-188.
Wiggins, J. (1987). Option values under stochastic volatility: Theory and empirical estimates. Journal of Financial Economics, 19:351-372.
Following Heynen et al. (1994) we derive the formula of the average expected volatility for the TGARCH (1,1) volatility stochastic process, as follows:
where:
Rt is the daily log-return;
The average expected volatility between t and t + k is defined as:
Firstly, it is necessary to express ht+k in terms of ht. We start by:
From Glosten et al. (1993) we have that the condictional variance, at date t, k-days ahead, assuming P(et > 0) = 50%, is computed as:
where the inconditional (long-run) variance is defined as:
As the formula of the average expected volatility contains a geometruic series, we use the formula of the sum of the terms of the geometric progression and express the average express volatility as:
with , we finally have:
where
- Ahoniemi, K. (2006). Modeling and forecasting implied volatility: An econometric analysis of the VIX index. Helsinki School of Economics, Discussion Paper 129.
- Amin, K. & Jarrow, R. A. (1991). Pricing foreign currency options under stochastic interest rates. Journal of International Money and Finance, 10:310-329.
- Andrade, S. C. & Tabak, B. M. (2001). Is it worth tracking dollar/real implied volatility? Revista de Economia Aplicada, 5(3).
- Becker, J. L. & Lemgruber, E. F. (1989). Uma análise de estratégias de negociação no mercado brasileiro de opções: Evidências a partir das opções de compras mais negociadas durante o Plano Cruzado. In Brito, editor, Gestão de Investimentos. Editora Atlas.
- Beckers, S. (1981). Standard deviations implied in options prices as predictors of future stock price volatility. Journal of Banking and Finance, 5:363-81.
- Berg, A. & Pattillo, C. (1998). Are currency crises predictable? A test. IMF Working Paper Series. n. 98/154.
- Black, F. (1976). The pricing of commodity contracts. Journal of Financial Economics, 4:167-179.
- Black, F. & Scholes, M. (1972). The valuation of option contracts and a test of market efficiency. Journal ofFinance, 27.
- Blejer, M. I. & Schumacher, L. (1998). Central bank vulnerability and the credibility of commitments: A value-at-risk approach to currency crises. IMF Working Paper Series, N. 98/65.
- Breeden, D. T. & Litzenberger, R. H. (1978). Prices ofstate contingent claims implicit in option prices. Journal ofBusiness, 51:621-652.
- Castro, P. C. (2002). Opções sobre dólar comercial e expectativas a respeito do comportamento da taxa de câmbio. Banco Central do Brasil, Brasília.
- Chesney Marc, S. L. (1989). Pricing european currency options: A comparison of the modified Black-Scholes model and a random variance model. Journal ofFinancial and Quantitative Analysis.
- Christensen, B. & Prabhala, N. R. J. (1998). The relation between implied and realized volatility. Journal of Financial Economics, 50(3):125-150.
- Craig, B. R. & Keller, J. G. (2004). The forecast ability of risk-neutral densities of foreign exchange. Federal Reserve Bank of Cleveland, WP 04-09.
- Cunha Jr., D. & Lemgruber, E. F. (2003). Opções de dólar no brasil com taxas de juro e de cupom estocásticos. III Encontro Brasileiro de Finanças. FEA, USP.
- Da Costa, M. N. & Yoshino, J. A. (2004). Calibração do modelo de heston para o mercado brasileiro. IV Encontro Brasileiro de Finanças.
- Day, T. & Lewis, C. (1992). Stock market volatility and the information content of stock index options. Journal ofEconometrics, 52:267-287.
- Dickey, D. A. & Fuller, W. A. (1979). Distribution of the estimators for autoregressive time series with a unit root. Journal ofthe American Statistical Association, 74:427-431.
- Doran, J. S. & Ronn, E. I. (2006). The bias in Black-Scholes/Black implied volatility: An analysis ofequity and energy markets. Florida State University Working Paper.
- Duan, J. (1995). The GARCH option pricing model. Mathematical Finance, 5(1):13-32.
- Feiger, G. & Jacquillat, B. (1979). Currency option bonds, puts and calls on spot exchange and the hedging of contingent foreign earnings. Journal of Finance, 34:1129-139.
- Fisher, R. A. (1922). On the interpretation of χ2 from contingency tables, and the calculation of P. Journal of the Royal Statistical Society, 85(1):87-94.
- Frankel, J. A. & Rose, A. K. (1996). Currency crashes in emerging markets: An empirical treatment. Journal of International Economics, 41:351-366.
- Garman, M. & Kohlhagen, S. (1983). Foreign currency options values. Journal of International Money and Finance, 2:231-237.
- Glosten, L. R., Jagannathan, R., & Runkle, D. (1993). On the relation between the expected value and the volatility of the nominal excess return on stocks. Journal of Finance, 48:1779-1801.
- Gomes, F. P. (2002). Volatilidade implícita antecipada de eventos de stress: Um teste para o mercado brasileiro. Departamento de Estudos e Pesquisas, Banco Central do Brasil, Brasília.
- Grabbe, J. O. (1983). The pricing of call and put options on foreign exchange. Journal of International Money and Finance, 2:239-253.
- Granger, C. W. J. (1969). Investigating causal relations byeconometric methods and cross-spectral methods. Econometrica, 34:424-438.
- Greene, W. H. (2003). Econometric Analysis. Prentice Hall, 5th edition.
- Heston, S. (1993). Aclosed-form solutionfor options with stochastic volatilitywith applications to bond and currency options. The Review ofFinancial Studies, 6(2).
- Heynen, R., Kemna, A., & Vorst, T. (1994). Analysis ofthe term structure ofimplies volatilities. Journal ofFinancial Quantitative Analysis, 29:31-46.
- Hilliard, J. E., Madura, J., & Tucker, A. L. (1991). Currency option pricing with stochastic domestic and foreign interest rates. Journal ofFinancial and Quantitative Analysis, 26(2):139-151.
- Hull, J. (2003). Options, Futures and Other Derivatives Securities. Prentice Hall, New Jersey, 5 edition.
- Hull, J. & White, A. (1987). The pricing ofoptions on assets with stochastic volatilities. JournalofFinance, 42:281-300.
- Jorion, P. (1995). Predicting volatility in the foreign exchange market. The Journal ofFinance, 50(2):507-528.
- Kaminsky, G., Lizondo, S., & Reinhart, C. (1998). Leading indicators ofcurrency crises. IMFStaffPapers,45(1):01-48.
- Lemgruber, E. F. (1995). Avaliação de Contratos de Opções. BM&F, São Paulo, revisada e ampliada edition.
- Malz, A. M. (2000). Do implied volatilities provide early warning ofmarket stress? RiskMetrics Journal, 1(1):41-60.
- Matos, J. A., Kapotas, J. C., & Schirmer, P. P. (2004). Pricing and hedging brazilian currency options. IV EBF.
- Melino, A. & Turnbull, S. M. (1991). The pricing of foreign currency options. Canadian Journal ofEco-nomics,Canadian Economics Association, 24(2):251-81.
- Merton, R. (1973). Theory ofrational option pricing. Bell Journal ofEconomics, 4:141-183.
- Navatte, P. & Villa, C. (2000). The information content of implied volatility, skewness and kurtosis: Empirical evidence from long-term CAC 40 options. European Financial Management, 6(1):41-56.
- Neeley, C. (2004). Forecasting foreign exchange volatility: Why is implied volatility biased and inefficient? And does it matter? Federal Reserve Bank ofSt. Louis Working Paper.
- Pownall, R. A. J. & Koedijk, K. G. (1999). Capturing downside risk in financial markets: The case of the Asian crisis. Journal ofInterantional Moneyand Finance, 18:853-870.
- Scott, L. (1992). The information content of prices in derivative security markets. IMF StaffPapers,39:596-625.
- Scott, L. O. (1987). Option pricing when the variance changes randomly: Theory, estimators, and applications. Journal ofFinance and Quantitative Analysis, 22:419-438.
- Scott, L. O. (1991). Random variance option pricing. Advances in Futures and Options Research, 5:113-135.
- Stein, E.M. & Stein, J. C. (1991). Stock price distributions with stochastic volatility. The Review of Financial Studies, 4(4).
- Vasicek, O. (1977). An equilibrium characterization of the term structure. Journal of Financial Economics, 5:177-188.
- Wiggins, J. (1987). Option values under stochastic volatility: Theory and empirical estimates. Journal of Financial Economics, 19:351-372.
APPENDIX A
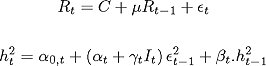

Publication Dates
-
Publication in this collection
18 Nov 2008 -
Date of issue
June 2008