Abstracts
In the present paper we obtain the infimal signal-to-noise ratio (SNR) required for the stabilizability of a linear output feedback loop over an additive white Gaussian noise (AWGN) channel in closed-form. The focus on AWGN channels allow us to then define the minimal channel capacity required for stabilizability. Finally, the infimal SNR for stabilizability also allow us to identify in closed-form the related stabilizing Hermitian positive semidefinite solution to the continuous-time algebraic Riccati equation of LQ control with vanishing state weight and repeated eigenvalues.
Control over networks; Infimal signal-to noise ratio; Additive white Gaussian noise channel; Repeated unstable poles; Repeated nonminimum phase zeros; Time delay; Channel capacity; Continuous-time algebraic Riccati equation
Neste artigo, obtemos a relação sinal-ruído ínfimo (SNR) necessário para a estabilizabilidade de um laço linear saída de realimentação ao longo de um canal de Gaussian aditivo branco ruído (AWGN) em forma fechada. O foco em canais AWGN nos permitirá, então, definir a capacidade do canal mínima exigida para estabilizabilidade. Finalmente, o SNR ínfimo para estabilizabilidade também nos permitem identificar em closed-formar o relacionado estabilização solução positiva Hermitiana semidefinida para a equação de Riccati de tempo contínuo algébrica de controle LQ com desaparecer o peso do Estado e autovalores repetidos.
Controle sobre redes; sinal-ruído ínfimo; Canal de ruído gaussiano aditivo branco; pólos instáveis repetidos; zeros de fase não minimos repetidos; Tempo de atraso; capacidade de canal; equação algébrica de Riccati contínua no tempo
TEORIA DE CONTROLE
Stabilizability of nonminimum phase unstable plants with arbitrary multiplicity over AWGN channels
Estabilizabilidade de plantas instáveis de fase não mínima com multiplicidade arbitrária através de canais AWGN
Alejandro J. Rojas
Departamento de Ingeniería Eléctrica, Universidad de Concepción, Concepción, Chile. arojasn@udec.cl
ABSTRACT
In the present paper we obtain the infimal signal-to-noise ratio (SNR) required for the stabilizability of a linear output feedback loop over an additive white Gaussian noise (AWGN) channel in closed-form. The focus on AWGN channels allow us to then define the minimal channel capacity required for stabilizability. Finally, the infimal SNR for stabilizability also allow us to identify in closed-form the related stabilizing Hermitian positive semidefinite solution to the continuous-time algebraic Riccati equation of LQ control with vanishing state weight and repeated eigenvalues.
Keywords: Control over networks; Infimal signal-to noise ratio; Additive white Gaussian noise channel; Repeated unstable poles; Repeated nonminimum phase zeros; Time delay; Channel capacity; Continuous-time algebraic Riccati equation.
RESUMO
Neste artigo, obtemos a relação sinal-ruído ínfimo (SNR) necessário para a estabilizabilidade de um laço linear saída de realimentação ao longo de um canal de Gaussian aditivo branco ruído (AWGN) em forma fechada. O foco em canais AWGN nos permitirá, então, definir a capacidade do canal mínima exigida para estabilizabilidade. Finalmente, o SNR ínfimo para estabilizabilidade também nos permitem identificar em closed-formar o relacionado estabilização solução positiva Hermitiana semidefinida para a equação de Riccati de tempo contínuo algébrica de controle LQ com desaparecer o peso do Estado e autovalores repetidos.
Palavras-chave: Controle sobre redes; sinal-ruído ínfimo; Canal de ruído gaussiano aditivo branco ; pólos instáveis repetidos ; zeros de fase não minimos repetidos; Tempo de atraso; capacidade de canal; equação algébrica de Riccati contínua no tempo.
1 INTRODUCTION
The main objective of control design is to direct the output of a system to a given desired target. It is well known that if the system, or plant model, is stable then a simple open loop configuration can potentially suffice. However, in practice, the use of feedback control is advised since it allow us to reject disturbances, deal with plant model uncertainties, and to include the case of unstable plant models.
Early on in the development of control theory it has been recognized that the design of a feedback control configuration is subject to unavoidable limitations, also known as fundamental limitations. Seminal results in this research area are the work by (Bode, 1945) and (Horowitz, 1963), followed more recently by results from (Freudenberg e Looze, 1985), (Looze e Freudenberg, 1991), (Middleton, 1991). For the linear time invariant (LTI) case it is thus well understood that the cause of such limitations resides on the presence and interaction of unstable poles, nonminimum phase (NMP) zeros and time-delay (see for example (Seron et al., 1997) and references therein).
In the last decade, the study of fundamental limitations has been extended to design problems of control over communication networks. The authors of (Braslavsky et al., 2007) and (Braslavsky et al., 2005) obtained the expression for the infimal SNR required to stabilize a finite dimensional unstable LTI plant over a memoryless additive white Gaussian noise (AWGN) channel when considering unstable plant poles, NMP zeros and plant time-delay. On the other hand, the additive colored Gaussian noise channel with memory has been studied for example in (Rojas, 2011), whilst performance limitations have also been considered for example in (Rojas, 2009c), (Silva et al., 2010) and (Wang et al., 2011). Here, motivated by the potential insights that can be gained, we retake the AWGN channel approach focusing on output feedback stabilizability of plant models with repeated NMP zeros.
Our first contribution in this paper is to present the infimal SNR required for output feedback stabilizability of a plant with time delay, repeated unstable poles and NMP zeros over an AWGN channel. This result differs from (Rojas, 2009b, Theorem 4) in that we explicitly solve the Laplace variable derivative left stated in (Rojas, 2009b). This simple fact allow us to investigate in more depth the implications stemming from the closed-form infimal SNR solution as shown by our other results reported here. Our second contribution, based on the known fact that the capacity of an AWGN channel does not increase with feedback, is to restate the infimal SNR result into a necessary and sufficient condition on the channel capacity.
Riccati equations (Lancaster e Rodman, 1995; Abou-Kandil et al., 2003), in particular algebraic Riccati equations (AREs), are a recurrent and important feature in many theoretical control design results, (Goodwin et al., 2001). The infimal SNR problem, here solved in closed-form, can also be addressed numerically as an LQ control problem by solving a continuous-time algebraic Riccati equation with vanishing state weight, see for example (Rojas, 2009a). It is then perhaps not entirely surprising that our third contribution, based on the equivalence of the infimal SNR result developed here with the result presented in (Braslavsky et al., 2005), is a closed-form characterization of the stabilizing Hermitian positive semidefinite solution to the continuous-time algebraic Riccati equation with vanishing state weight that lays behind the infimal SNR problem. To the best knowledge of the author such closed-form solution is novel and differs from (Rojas, 2010a) in that repeated unstable poles are explicitly considered.
The paper is organized as follows: In Section 2 we introduce the assumptions for the present work. In Section 3 we present the infimal SNR for stabilizability result. In Section 4 we discuss the implications in terms of the channel capacity and connect the infimal SNR for stabilizability result to the stabilizing Hermitian positive semidefinite solution in closed-form of a related class of continuous-time algebraic Riccati equations with vanishing state weight. Finally, in Section 5, we give concluding remarks for the present work. A preliminary version of the present results has been communicated in (Rojas, 2010d).
Terminology: let denote the complex plane. Let
-,
-,
+ and
+ denote respectively the open left-plane, closed left-plane, open right-plane and closed right-plane of
. Let
denote the set of real numbers,
+ the set of positive real numbers,
+o the set of non-negative real numbers and
- the set of real negative numbers. Let
+ denote the set of positive integers. A continuous-time signal is denoted by x(t), and its Laplace transform by X(s), s ∈
. The expectation operator is denoted by
. A rational transfer function of a continuous-time system is minimum phase if all its zeros lie in





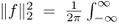










2 PRELIMINARIES
The assumptions for the closed loop system shown in Figure 1 are for the continuous-time plant with time-delay to be defined as
G(s) = Go(s)e-sτ,
where Go(s) is a nonminimum phase, rational transfer function with relative degree ng > 1, containing m distinct unstable poles pi ∈ +, i = 1,...,m each with multiplicity ni and q distinct NMP zeros ζj∈
+, j = 1,...,q each with multiplicity oj, also distinct of each and every unstable pole. We further assume that the transfer function Go(s) can be alternatively represented by a state-space description (A,B,C,0) that satisfies:
(1) (A, B, C, 0) is a minimal realization of Go(s) such that
where A ∈
n×n, B ∈
n×1, C ∈
1×n, Au∈
with nu =
ni, Bu ∈
, Cu∈
.
(2) The eigenvalues of Au are all in
+.
(3) Au is block-diagonal,
Au = diag{Ai}, ∀i = 1, ... , m,
with
and Bu =
with
for all i = 1, ..., m.
(4) The eigenvalues of As are all in
-.
Assumption (1) implies that the pair (Au, Bu) is controllable, (Kailath, 1980). Also notice that, in order to satisfy Assumption (3) for Au, we are implicitly assuming for any original real coefficients system to be transformed into the equivalent system with A diagonal, (potentially) containing complex conjugate coefficients. This can always be achieved by means of the transformation matrix collecting all the eigenvectors as explained in, for example, (Strange, 1988).
As depicted in Figure 1 the channel model is a memoryless AWGN channel with the additive noise process n(t) assumed to be an i.i.d. zero-mean Gaussian white noise process with power spectral density Φ.
3 INFIMAL SNR FOR STABILIZABILITY
We further consider C(s) such that the closed loop system is stable in the sense that, for any distribution of initial conditions, the distribution of all signals in the loop will converge exponentially fast to a stationary distribution.
The channel input power , under reasonable stationarity assumptions (Åström, 1970, §4.4), can be computed by means of its spectral density Su(ω) as follows
In turn the power spectral density Su(ω) can be obtained as Su(ω) = |Tun(jω)|2Φ, where the transfer function Tun(s) maps the closed-loop from the channel noise n(t) to channel input u(t) and is equal to
If the feedback system is stable, then the power of the channel input signal is thus given by =
Φ. The channel input power P is then lower bounded by
. This fact can then be restated as a constraint imposed on the transfer function (2) by the admissible channel SNR
Denote the Blaschke products containing the unstable poles and NMP zeros of G(s) (that is the poles and zeros in +) by
We follow-up next with what is the first contribution of the present work.
Proposition 1 (Continuous-time Infimal SNR for Stabilizability) Consider the output LTI feedback presented in Figure 1 with G(s) a nonminimum phase, rational transfer function with relative degree ng > 1, containing m distinct unstable poles pi ∈ +, i = 1, ..., m each with multiplicity ni, and containing q distinct NMP zeros ζj ∈
+, j = 1, ..., q each with multiplicity oj, also distinct from each and every unstable pole. The necessary and sufficient memoryless AWGN channel SNR
that guarantees stabilizability for the closed loop satisfies
where
Proof: From (Rojas, 2009b, Theorem 4) we have that
with
Notice first that ri,l can be rewritten as
Introduce now a change of variable such that k = - l + 1 so that we have
which can again be rewritten as
with ri,l,k as in (4). Similar steps can be applied to rj,z to observe that
We now focus on the term
We explicitly develop the differentiation on s to obtain
Now we complete the factorial in the numerator and observe the resulting combinatorial number
We finally conclude by replacing (6), (7) and (8) into (5) and obtain as a result (3), which concludes the proof.
□
The expression in (3) extends the result in (Rojas, 2009b, Theorem 4) for a memoryless AWGN channel (see (Rojas, 2009b) for more details) in two directions: first it explicitly develops the derivative left implicit in (Rojas, 2009b) and second it clarifies the impact of the plant time delay τ on the infimal SNR, this time through the residue factors ri,l.
Example 1: Consider here the case of three unstable poles p1∈ [0,4] with multiplicity n1 = 2 and p2 = with multiplicity n2 = 1. The residue coefficients ri,l,k predicted by(4) (with i = 1,2, l = 1,2 and k = 1,2) are
The channel SNR sufficient for stabilizability is then lower bounded by the quantity
which can be further specified as
Notice that if τ = 0 then the residue factor r1,1,2 will not play a role on the infimal SNR required for stabilizability.
4 IMPLICATIONS OF THE INFIMAL SNR RESULT
The result from Proposition 1 gives insight into the fundamental limitations in a control over networks setting by establishing the presence of a lower bound on the channel SNR. The choice of AWGN channel model can be criticized as highly idealized, however it is useful in clarifying what are the causes of the SNR limitation, namely the plant unstable poles, nonminimum phase zeros and time delay. The interplay between these elements allow us, for example, to observe that
1) As the real part of the unstable poles tends to zero, the value of the infimal SNR for stabilizability will tend to zero.
2) As the value of any unstable pole, independent of its multiplicity, approaches the value of any given nonminimum phase zero the infimal SNR required for stabilizability will tend to infinity. This can be interpreted as the onset of instability.
3) The presence of the time-delay increases the infimal SNR required for stabilizability, and its effect is worsened by the multiplicity of the unstable poles.
The above observations are in line with classical results, such as (Freudenberg e looze, 1985), (Looze e Freudenberg, 1991), (Middleton, 1991). However Proposition 1 is novel in that it characterizes the fundamental limitation in terms of a communication channel feature, the SNR, instead of a closed-loop relationship like the sensitivity function.
Another option is to quantify the fundamental limitation imposed by the presence of the AWGN channel in terms of its channel capacity. The channel capacity for a memoryless AWGN channel in continuous time is given by C = (log2e) (see (Cover e Thomas, 1991, §10) or (Braslavsky et al., 2007)). Direct substitution of infimal SNR result into this definition gives
where is the channel infimal capacity for stabilizability. Observe that, of course, the SNR limitation is directly "mapped" into a channel capacity limitation, whilst the difference from one to another is given by only the constant factor (log2sqrte).
For the very simple case of one unstable pole p1∈ + we then have
From the above results and Proposition 1 we have that the effect of the time delay is a polynomial in p1τ of order 2n1 - 2, whilst for the very simple case of τ = 0 we obtain (see (Rojas, 2010c) for the details on the simplifying argument) C > p1n1(log2), thus the channel capacity limitation (as the SNR limitation) grows linearly with the value of the unstable pole and its multiplicity, as observed in (Braslavsky et al., 2007).
We follow up the discussion in the present section by using the infimal SNR for stabilizability result of Proposition 1 to study the continuous-time algebraic Riccati equation solution that lays behind the infimal SNR solution.
4.1 Continuous-time Algebraic Riccati Equation
Another rather unexpected implication that we derive from the main result developed in the previous section is the characterization in closed-form of the related continuous-time algebraic Riccati equation (Kailath, 1980)
with vanishing state weight, that is Q = ε2I with ε → 0. Under the assumptions for A and B, with R > 0, there is a unique Hermitian positive semidefinite solution to (9), such that A - R-1BTP has all its eigenvalues in the open left half-plane. The unique stabilizing Hermitian positive semidefinite solution of (9) satisfies the following lemma.
Lemma 2 (Adapted from (Braslavsky et al., 199, Lemma 2)) Under the proposed assumptions the variance of the state satisfies the unique stabilizing Hermitian positive semidefinite solution of (9) satisfies
where Pu is the stabilizing Hermitian positive semidefinite solution to the algebraic Riccati equation
We now present the closed-form characterization of the non-trivial solution Pu to the continuous-time algebraic Riccati equation with vanishing state weight in (10).
Proposition 10 (Closed-Form Solution for R = 1) The closed-form u matrix that solves the continuous-time algebraic Riccati equation with vanishing state weight in (10) is given by the i-row, j-column block matrix Pij
In turn, each block matrix Pij is defined by the ε-row, η-column element
and ri,l,k as in (4) under a minimum phase assumption for the plant model, that is with Bζ(s) = 1.
Proof: We begin this proof by evaluating the expression
Upon replacing
u as in , together with Au and Bu as in Assumption (3), we obtain
We now replace Pij as in to obtain
and perform explicitly the matrix multiplication between the last two matrices on the RHS. By replacing η = nj + 1 - w and noticing that the sum can be equally expressed as
, we obtain
We now perform the matrix multiplication between the two remaining matrices on the RHS of (13). We simultaneously substitute ε with k, such that k = ni + 1 - ε, and notice that the sum can be equivalently expressed as
. As a result we then obtain
which, upon taking conjugate on the RHS, matches the result in (3) (since the SNR lower bound is itself a positive real number). For any arbitrary vector w ∈ we have
Observe that the RHS of the above expression is positive since the sum is a squared H2 norm and wTw is a quadratic expression. Let us introduce the notation v = Buw and notice that since w is an arbitrary vector in
, then also v is an arbitrary vector in
. As a result we have that
u satisfies
and thus proved that
u is a positive semidefinite matrix.Let us consider now the lower bound to the channel SNR for LTI stabilizability stated in (Braslavsky et al., 2005), which is under the plant minimum phase assumption a result equivalent to the one presented in equation (3), and is given by
with δ = and P the stabilizing Hermitian positive semidefinite solution of (9). From Lemma 2 we have that δ =
. Also we notice from (Rugh, 1995, Exercise 7.12, p.217) that
whilst from (Braslavsky et al., 2007, Proof of Theorem 2.1) we have that
Thus the RHS of reduces to . In summary we have that:
- The results in (3) and in (14) are equivalent under the plant minimum phase assumption.
- The result in (14) can be restated as
where Pu is the stabilizing Hermitian positive semidefinite solution of (10).
-
u satisfies the restated form of (14), it is an Hermitian matrix by its definition and it is also a positive semidefinite matrix.- The stabilizing Hermitian positive semidefinite solution of a continuous-time algebraic Riccati equation is unique, (Lancaster e Rodman, 1995).
As a result of the above facts we conclude that
u = Pu, that is, the proposed closed-form solution
□
Notice that the choice of R = 1 is without loss of generality since the closed-form solution Pλ for R = λ can be stated as Pλ = λ. We follow up by presenting a suitable example.
Example 2: Let us consider the same case as in Example 1. The overall closed-form solution for Pu is then given by
In Figure 2 we observe a comparison between the above closed-form solution and the solution obtained with the Matlab command care. For matters of comparison we introduce the following error function
where the sum is over each row and column element quantifying the numerical mismatch between the LHS and RHS of (10). In Figure 2 we show the plot of 10log10(eP) as a function of p1 for both the closed-form solution and the algorithmic solution implemented by the command care. We observe that the closed-form solution, for this example, is slightly superior in terms of numerical precision to the one obtained with Matlab. Notice also that as p1 approaches we approach a loss of controllability in the system under study. This can be directly verified since the A and B matrices in this example are
and the controllability matrix results in
Thus we observe that as p1→ p2 the above matrix loses full rank, controllability is lost and the terms in the closed-form solution tend to infinity due to the definition of the residue factors ri,l,k (see Example 1).
4.1.1 Transformed Solution
The result from Proposition 3 can readily be extended in many directions. Consider, for example, the closed-form solution for R = 1 subject to a state-space transformation T =
. The transformed closed-form solution can then be obtained as
We make use of the above result to show how the closed-form continuous-time algebraic Riccati equation solution presented in (Rojas, 2010a) links to Proposition 3.
4.1.2 Rapprochement to Previous Continuous-Time Algebraic Riccati Equation Results
The result in (Rojas, 201a) does not account for multiplicities greater than one for the unstable eigenvalues. To better frame the present discussion we introduce the closed-form solution as stated in (Rojas, 201a) for two different unstable eigenvalues p1 and p2, both with multiplicity 1 and both in +
Notice that as p1→ p2, or viceversa, the above solution diverges due to the factor p1- p2 in the denominator of each term. Observe that, under the assumption that p1≠ p2 the same result is obtained from Proposition 3. However, we can also use an є argument to obtain a solution arbitrarily close in value to the one for repeated eigenvalues. More so, to avoid the explicit problem imposed by the factor p1 - p2, and as suggested by the result in (15), we can make use of the transformation
which gives, in turn, the following transformed closed-form solution
Now, as p1→ p2, the above solution converges to the value , which is the result predicted by Proposition 3 for an unstable pole with multiplicity 2. We thus have shown that the result in Proposition 3 and (Rojas, 2010a) agree under both scenarios that is p1≠ p2 and p1 = p2, for example by invoking a particular non-singular transformation T.
4.1.3 Extension to the Multivariable Case
The plant model representation discussed in Section 2 is restricted to a single-input single-output (SISO) system. We illustrate the point by using the simple case of one real unstable pole with multiplicity 2
where we have dropped the u-subindex for clarity. Notice that the choice of BT = [0 1] is without loss of generality since we can always choose C = . The same applies to the case of a single-input multiple-output (SIMO) system. The situation for a multiple-input single-output (MISO) system is different. Let us consider again the simple case of one real unstable pole with multiplicity 2, such a system would then be described by
Given the dimensions of B we can extend our focus to the multiple-input multiple-output (MIMO) case
For both the MISO and MIMO case the change in dimensions of B renders the approach of Proposition 3 unsuitable. More so, the amount of residue coefficients does not allow any element of B to be 1 without loss of generality as in the SISO and SIMO cases. The question is thus, can we deal with a B matrix with dimensions n×r with r > 1?. A partial answer can be found by observing the continuous-time algebraic Riccati equation with vanishing state weight in (10). Let us define
If we multiply (10) by Y from the left and right we obtain
where again we have dropped the u-subindex for clarity. Notice that equation (16) is a Lyapunov equation which can be solved in closed-form since each i - j component of Y is given by
with Yi+1,j = 0 if i = n and Yi,j+1 = 0 if j = n. For the simple case of one real unstable pole with multiplicity 2 the closed-form solution Ŷ to the Lyapunov equation is then
We then have, for the limited 2×2 case that the inverse of Y is given by
Thus, applying the above result to the MIMO case with B = with R =
gives
We then have that the answer to the question "can we deal with a B matrix with dimensions n×r with r > 1?" is in the positive and a closed-form solution to the continuous-time algebraic Riccati equation can still be found. At the same time we notice that the closed-form solution for such a B matrix is not a direct implication of the result in Proposition 1 and thus it is outside the scope of the present work.
4.2 Beyond Stabilization
The infimal SNR for stabilization result in Proposition 3 minimizes the power at the channel input. Therefore intuition suggests that performance should impose a greater SNR lower bound. This has been verified for a disturbance rejection type of performance in (Rojas, 2009c). Performance can also be introduced by modifying the convex functional as to find a stabilizing controller that minimizes the channel input power and simultaneously, for example, the plant output power. Such modification can be directly handled in the LQG/LTR setting, as suggested in (Rojas, 2009a), or through an LMI setting, as in turn suggested in (Elia, 2005). We expect that the resulting continuous-time algebraic Riccati equation will now include a non-vanishing state weight as in (9). Notice that performance subject to constrained SNR is also the focus, in a slightly different setting, of (Silva et al., 2010).
5 CONCLUSION
In this paper we have presented the infimal SNR for LTI stabilizability in closed-form when the plant LTI model has repeated unstable poles, repeated nonminimum phase zeros and time-delay. We then followed by presenting the solution in closed-form to the related class of continuous-time algebraic Riccati equations with vanishing state weight and repeated unstable eigenvalues. This result extends on the previously reported result for distinct unstable eigenvalues, (Rojas, 2010a). Future research should consider recent developments in the study of SNR limitations for MIMO systems (Shu e Middleton, 2011), as well as extending the class of continuous-time algebraic Riccati equations to the case of non vanishing state weight (as suggested by the preliminary results developed in (Rojas, 2010b)).
ACKNOWLEDGMENTS
The author thankfully acknowledges the support from CONICYT, through project grant FONDECYT 11100080.
Artigo submetido em 11/01/2011 (Id.: 1247)
Revisado em 05/05/2011, 05/11/2011, 01/12/2011
- Abou-Kandil, H., Freiling, G., Ionescu, V. e Jank, G. (2003). Matrix Riccati Equations, Birkhäuser.
- Åstrom, K. (1970). Introduction to Stochastic Control Theory, Academic Press.
- Bode, H. (1945). Network Analysis and Feedback Amplifier Design, Princeton, NJ: Von Nostrand.
- Braslavsky, J. H., Middleton, R. H. e Freudenberg, J. S. (2005). Effects of Time Delay on Feedback Stabilization over Signal-to-Noise Ratio Constrained Channels, Proceedings of the 16th IFAC World Congress, Prague, Czech Republic.
- Braslavsky, J. H., Middleton, R. H. e Freudenberg, J. S. (2007). Feedback Stabilisation over Signal-to-Noise Ratio Constrained Channels, IEEE Transactions on Automatic Control 52(8): 1391-1403.
- Braslavsky, J. H., Seron, M., Mayne, D. Q. e Kokotovic, P. V. (1999). Limiting performance of optimal filters, Automatica 35(2): pp. 189-199.
- Cover, T. e Thomas, J. (1991). Elements of Information Theory, John Wiley & Sons.
- Elia, N. (2005). Remote stabilization over fading channels,Systems & Control Letters 54(3): 237-249.
- Freudenberg, J. S. e Looze, J. (1985). Right-half plane zeros and poles and design trade-offs in feedback systems, IEEE Transactions on Automatic Control 30(6): 555-565.
- Goodwin, G. C, Graebe, S. F. e Salgado, M. E. (2001). Control System Design, Prentice Hall.
- Horowitz, I. M. (1963). Synthesis of Feedback Systems, Academic Press.
- Kailath, T. (1980). Linear Systems, Prentice Hall.
- Lancaster, P. e Rodman, L. (1995). Algebraic Riccati Equations, Oxford University Press.
- Looze, J. e Freudenberg, J. S. (1991). Limitations of Feedback Properties Imposed by Open-Loop Right Half Plane Poles, IEEE Transactions on Automatic Control 36(6): 736-739.
- Middleton, R. H. (1991). Trade-offs in Linear Control System Design, Automatica 27(2): 281-292.
- Rojas, A. J. (2009a). Linear Quadratic Gaussian Optimization Approach for Signal-to-Noise Ratio Constrained Control over Network, International Journal of Control, Automation and Systems 7(6): 971-981.
- Rojas, A. J. (2009b). Signal-to-Noise Ratio Fundamental Limitations in Continuous-Time Linear Output Feedback Control, IEEE Transactions on Automatic Control 54(8): 1902-1907.
- Rojas, A. J. (2009c). Signal-to-noise ratio performance limitations for input disturbance rejection in output-feedback control, Systems & Control Letters 58(5): 353-358.
- Rojas, A. J. (2010a). Closed-form solution for a class of continuous-time algebraic Riccati equations, Automatica 46(1): 230-233.
- Rojas, A. J. (2010b). On the Continuous-Time Algebraic Riccati Equation and its Closed-Form Solution, Proceedings of the 49th IEEE Conference on Decision and Control, Atlanta, Georgia, USA.
- Rojas, A. J. (2010c). On the Equivalence of Two Recent Control over Network Results, Proceedings of the 49th IEEE Conference on Decision and Control, Atlanta, Georgia, USA.
- Rojas, A. J. (2010d). On the Solution of a Class of Algebraic Riccati Equations with Repeated Unstable Eigenvalues,Proceedings of the 2010 American Control Conference, Baltimore, MD, USA.
- Rojas, A. J. (2011). Signal-to-noise ratio fundamental constraints in discrete-time linear output feedback control,Automatica 47(2): 376-380.
- Rugh, J. (1995). Linear System Theory, Prentice Hall.
- Seron, M. M., Braslavsky, J. H. e Goodwin, G. C. (1997). Fundamental Limitations in Filtering and Control, Springer.
- Shu, Z. e Middleton, R. H. (2011). Stabilization Over Power-Constrained Parallel Gaussian Channels, IEEE Transactions on Automatic Control 56(7): 1718-1724.
- Silva, E. I, Goodwin, G. C. e Quevedo, D. E. (2010). Control system design subject to SNR constraints, Automatica 46(2): 428-36.
- Strang, G. (1988). Linear Algebra and its Applications, third edn, Brooks/Cole.
- Wang, B., Guan, Z.-H. e Yuan, F.-S. (2011). Optimal tracking and two-channel disturbance rejection under control energy constraint, Automatica 47(4): 733-738.
Publication Dates
-
Publication in this collection
09 Aug 2012 -
Date of issue
Aug 2012
History
-
Received
11 Jan 2011 -
Reviewed
01 Dec 2011