Abstract
Internet-based customization tools can be used to design service encounters that maximize customers' utility in the present or explore their tastes to provide more value in the future, where these two goals conflict with each other. Maximizing expected customer satisfaction in the present leads to slow rates of learning that may limit the ability to provide quality in the future. An emphasis on learning can lead to unsatisfied customers that will not only forego purchasing in the current period, but, more seriously, never return if they lose trust in the service provider's ability to meet their needs. This paper describes service design policies that balance the objectives of learning and selling by characterizing customer lifetime value as a function of knowledge. The analysis of the customization problem as a dynamic program yields three results. The first result is the characterization of customization policies that quantify the value of knowledge so as to adequately balance the expected revenue of present and future interactions. The second result is an analysis of the impact of operational decisions on loyalty, learning, and profitability over time. Finally, the quantification of the value of knowing the customer provides a connection between customer acquisition and retention policies, thus enhancing the current understanding of the mechanisms connecting service customization, value creation, and customer lifetime value.
Service customization; customer learning; customer lifetime value; dynamic programming
Design to learn: customizing services when the future matters
Dan ArielyI; Gabriel BitranII; Paulo Rocha e OliveiraIII, * * Corresponding author
IDuke University - Fuqua School of Business, 2024 W. Main Street, Durham, NC 27705. E-mail: dandan@duke.edu
IIMIT - Sloan School of Management, 77 Massachusetts Avenue, Cambridge, MA 02042, USA. E-mail: gbitran@mit.edu
IIIIESE Business School, Avinguda Pearson 21, 08034 Barcelona, Spain. E-mail: paulo@iese.edu
ABSTRACT
Internet-based customization tools can be used to design service encounters that maximize customers' utility in the present or explore their tastes to provide more value in the future, where these two goals conflict with each other. Maximizing expected customer satisfaction in the present leads to slow rates of learning that may limit the ability to provide quality in the future. An emphasis on learning can lead to unsatisfied customers that will not only forego purchasing in the current period, but, more seriously, never return if they lose trust in the service provider's ability to meet their needs. This paper describes service design policies that balance the objectives of learning and selling by characterizing customer lifetime value as a function of knowledge. The analysis of the customization problem as a dynamic program yields three results. The first result is the characterization of customization policies that quantify the value of knowledge so as to adequately balance the expected revenue of present and future interactions. The second result is an analysis of the impact of operational decisions on loyalty, learning, and profitability over time. Finally, the quantification of the value of knowing the customer provides a connection between customer acquisition and retention policies, thus enhancing the current understanding of the mechanisms connecting service customization, value creation, and customer lifetime value.
Keywords: Service customization, customer learning, customer lifetime value, dynamic programming.
1 INTRODUCTION
Electronic interfaces for service delivery present unprecedented opportunities for customization. Companies can identify returning customers at almost no cost and present each of them with a unique interface or service offering over a web page, voice interface, or mobile device. Customization is important in these interactions because the variety of products, services and information that companies can offer can be enormous and customers are often not able to find and identify the alternative that best suits their preferences. More generally, the amount of information available over the Internet is overwhelming and the costs to search and evaluate all potentially relevant pieces of information can be prohibitive.
This situation can lead to interactions of low net utility to customers. Hence the need for software applications generally called intelligent agents (or smart agents) that present customers with customized recommendations from large sets of alternatives. These agents have the potential to create great benefits for both consumers and companies. It is certainly desirable for customers to reduce search costs and by going directly to a company that already knows them and gives them high utility. At the same time, companies that learn about their customers can use this knowledge to provide increasingly better service as the relationship progresses, making it difficult for competitors to "steal" their customers.
In order to fully reap the benefits of customization, companies must overcome two obstacles. First, the strategies that maximize selling (or their customers' utility in the current period) do not coincide with strategies that maximize learning. Second, the cost of making bad recommendations (and learning form them) might be prohibitive because customers rarely forgive and return to service providers that make bad recommendations. Overcoming these obstacles depends on the agents' ability to adequately balance the goals of learning at the expense of selling and selling at the expense of learning.
In addition to being an important tool in the reduction of search costs, smart agents are also cost-efficient. Consequently, as more and more services are delivered through automated interfaces, smart agents have been playing increasingly important roles in everyday decision-making and are gaining considerable more autonomy to act on the consumers' behalf (Maes [1995] documents the early days of this tendency).
The essential task of learning about customers in order to provide better recommendations has been made more difficult in many ways as interfaces change from human-human to human-computer. Human intermediaries can, in many cases, observe their clients and make a number of inferences on the basis of their interactions. The lack of cues typical of the personal encounter diminishes the opportunities for correcting incomplete advice or wrong guesses. Software agents only register click patterns, browsing behavior, and purchase histories. These agents can only learn about customers by asking them questions and by observing their behavior. The issues related to the benefits and limitations of asking questions have been adequately addressed elsewhere: clients may not be willing nor have the patience to answer questions (e.g., Toubia et al., 2003), and their replies are not always truthful for various (and sometimes intentional) reasons (White, 1976). Regardless of how they answer surveys, customers spend their time and money in the activities from which they derive the most utility. In the offline world, Rossi et al. (1996) found that the effectiveness of a direct marketing campaign was increased by 56% due to the customization of coupons based on observations of one single purchase occasion. Intelligent agents can learn about customers by observing the purchases they make. Furthermore, firms interacting with customers through automated interfaces can also lean by observing the products that customer reject. Therefore, companies must strive to learn about their customers by observing how they react to the recommended products and services to which they're exposed.
Intelligent agents face a dilemma: they must either sell at the expense of learning or learn at the expense of selling. More precisely, they can either make recommendations that they expect their customers to like and learn about their customers' preferences at slow rates or they can take more risks by suggesting products their customers might not accept and learn at higher rates. The agent's dilemma captures the essence of the selling versus learning trade-off faced by companies that customize services on the Internet. Observing an action that was already expected to happen only reconfirms something one already knew or suspected to be true. But should an agent recommend a product with no prior knowledge of how their customers would react? By taking this course of action, agents may learn a lot about their customers' responses to recommendations of such products, regardless of whether or not the item is purchased. However, they run the risk of causing an unsuccessful service encounter that may lead to defection.
Customers who receive very bad recommendations can lose trust in the smart agent's ability to help them make better decisions and never return. Research in psychology and marketing has repeatedly shown the importance of trust as the basis for building effective relationships with customers in e-commerce as well as in regular commerce ( e.g., Kollock, 1999; Lewicki et al., 1998; Ariely et al., 2004). Doney & Cannon (1997) established an important link between trust and profitability by noting the importance of trust to build loyalty. Low trust leads to low rates of purchase, low customer retention and, consequently, meager profits. Studies on human and non-human agents have extended the findings of previous research by showing that trustworthy agents are more effective, i.e., they are better at engaging in informative conversation and helping clients make better decisions. Urban et al. (2000) have also shown that advice is mostly valued by less knowledgeable and less confident buyers, and those are exactly the same customers less likely to trust external agents to act as surrogates on their behalf. The human element involved in company-customer relationships makes it imperative that firms strive to build and maintain trust if they are to maintain profitable customers in the long run. The question is how these principles can be operationalized in the strategies adopted by agents.
Intelligent agents must strive to be trustworthy in two ways. First, customers must trust that agents have their best interest in mind. This will be true whenever the agent's payoffs are proportional to each customer's utility. Second, the customer must trust that the agent is good. Models of online consumer behavior must take into account the fact that if the agent makes a very bad recommendation the consumer loses trust and may never come back. The consequences of giving bad advice are much worse for software agents than for human agents. Ariely et al. (2004) conducted a series of experiments to compare trust online and offline and found that even though customers are sometimes willing to forgive a mistake made by a human agent, they rarely do so for a software agent. In one of his experiments, half of the subjects received financial advice from a software agent, and the other half from a human agent. Both agents were manipulated so that they made exactly the same serious mistake. After realizing they had received bad advice, customers of the human agent were much more likely to continue the relationship than customers of the software agent. People forgive people but they don't forgive software. Turning off the computer or clicking on a different website entails much less psychological costs than terminating a personal relationship. Consequently software agents face much higher churn rates than their human counterparts. The levels of tolerance for incomplete, wrong or misleadingadvice are lower, yet the electronic agent must rely on much more precarious information and cues regarding consumers' tastes and preferences.
The customization policy used by the agent affects the customer lifetime value (CLV) in two ways. CLV is typically defined as the net present value of the stream of cash flows the firmexpects to receive over time from a given customer (e.g., Berger & Nasr, 1998; Jain & Singh, 2002). The customization policy determines expected quality, which in turn influences (1) the customer defection decision and (2) the expected revenue per interaction. The model analyzed in this paper does not make any assumptions about the retention rate and profitability as the relationship progresses. These parameters are determined by the business setting and the manager's decisions. This flexibility is an important contribution of this paper, as it presents a tool that can accomodate situations where profits accelerate over time as well as those when profits do not accelerate over time.
The next section of this paper formalizes the customer behavior model, describes the dynamics of customer/company interactions, and formulates the agent's customization problem as a dynamic program. Section 3 establishes the optimality conditions and solution procedures for the model introduced in Section 2. Section 4 discusses the significance of the results, paying close attention to their meaning in the context of CLV research. Finally, Section 5 offers some directions for further research.
2 MODEL DESCRIPTION
This section is divided into three parts. The first one presents a mathematical choice model that captures the essential behavioral features of the choice process customers go through when interacting with software agents. This is followed by a description of a dynamic system used to model the interactions between customers and companies over time. The third part shows how the company's decision of how to customize products and services can be framed in terms of a Markov Decision Process using the customer behavior model of Section 2.1 and the dynamical system of Section 2.2.
2.1 Customer Behavior
This section describes a customer behavior model that captures the ways in which customers react to recommendations of products and services made by software agents that is consistent with the behavior qualitatively described in the psychology and marketing literature ( e.g.,Zauberman, 2000; Ariely et al., 2004). Customer observe the true quality of the recommendation, plus or minus an error term. The probability that the customer will accept the recommendation, reject the recommendation, or leave the company is defined through a random utility model (e.g., McFadden, 1981; Meyer & Kahn, 1990). According to random utility theory, when a customer is offered product X the utility that is actually observed has two components: a deterministic component u(X), which corresponds to the true underlying utility and an error term e. The error term accounts for factors such as unobserved attributes, imperfect information, or measurement errors that make the customer unable to determine the exact utility of a product upon initial examination. Formally, this can be expressed as
û(X) = u(X) + ε
where û(X) is the observed utility.
Once customers evaluate the utility of X, the service they are being offered, they can take one of three actions:
-
Accept product X if û(X) > 0
-
Reject product but come back next time if -c < û(X) < 0
-
Leave the company and never come back if û(X) < -c
Customers who observe a very low utility (-c, which can be interpreted as a switching cost) immediately lose trust in the agent as a reliable advisor and never return. In the case of services provided over smartphone apps, this can be interpreted as unistalling the app. The recommendation is accepted if the observed utility exceeds a given threshold, which can be assumed to be 0 without loss of generality. Customers who accept the suggested product or service are satistfied and return the next time they require service. If a recommendation is barely below the acceptance level, customers will purchase elsewhere (or forego purchasing in the current period) but return in the future. The cost of not making a sale by experimenting with different customization policies is captured by the probability that the observed utility will fall in the interval [-c,0].
The probabilities of accepting, leaving, or rejecting a product X (denoted pA(X), pL(X), and pR(X), respectively) can be computed by defining the distribution of ε. If ε is normally distributed with mean 0 and variance σ2, the parameter σ can be altered to represent different types of products or business settings, where the customer observes products with more or less error. This model corresponds to the ordered Probit model, and its properties are thoroughly discussed in Greene (2000). The general formulation of the choice probabilities is shown in the first column of (1), and the specific form they take when ε ~ N(0,σ) is shown in the second column (where Φ represents the cumulative normal function). Figure 1 depicts the probabilities taking each of the three possible actions when the customer is offered a recommendation with a deterministic utility component u. In this particular case, u is below the acceptance threshold, but since the observed utility is u + ε the probability that the product will be accepted is positive.
2.2 Dynamics of Customer-Company Interactions
Figure 2 describes the dynamics of customer/company interactions. Since the interactions take place through an automated interface controled by a smart agent, the words "company" and "agent" are used interchangeably. This system is the basis of the formulation of the agent's dilemma as a Markov decision problem.
Each customer belongs to a segment Si ∈ S. The company does not know the segment to which the new customer belongs, but has prior beliefs. These beliefs are represented by a |S|-dimensional vector b0∈ B, with each component corresponding to the probability that the customer belongs to a given segment. If S* denotes the true segment to which the customer belongs, the i'th component of the prior belief vector is bi0 = Pr(S* = Si) ∈ [0,1]. Since all customers must belong to some segment, bi0 = 1. The learning problem faced by the agent is the assignment of an individual customer to one of the |S| segments.
Each segment Si is defined by a utility function ui(X) that maps products and services X into real numbers. One way to define these utility functions is by associating each segment Si with a vector βi. The components of βi are the weights a customer of segment i gives to each attribute of the products in X. The corresponding utility function could be defined as ui(Xj) = (βi'·Xj) , where ui(Xj) denotes the utility that product Xj has to a customer of segment Si.
When the customer requests service, the company chooses a product from the set X = {X1, X2, ..., X| X|}. X is a set of substitute products or services, and can be thought of as the different ways in which a service can be customized. Each Xi ∈ X is a vector whose components correspond to different levels of the attributes of the product or service. The recommendation made during the t'th interaction is denoted Xt. The decision problem of which service configuration should be chosen is directly addressed as an optimization problem in Section 2.3.
The customer will either accept the recommendation, reject the recommendation, or leave, as explained in the beginning of this section. The probabilities with which each of these actions take place are given by (1). The company will observe the customer's action as described in Figure 2. The observation made during the t'th interaction is denoted θt, and the set of possible observations is Θ = {θA, θR, θL}, corresponding to accepting the recommendation, rejecting the recommendation, and leaving the system. For example, θ5 = θA means that the customer accepted the product offered during the fifth interaction.
After each observation, beliefs are updated in light of the previous belief and the action/observation pair of the last interaction. Bertsekas (1995a) proved that the last belief, bt-1 is a sufficient statistic for (b0, X1, θ1, X2, θ2, ..., Xt-1, θt-1). Thus, if bit denotes the i'th component of the belief vector after t interactions, then
bit = Pr(S* = S1|bt-1, Xt, θt) .
The new belief can be computed using
where
is a normalizing factor. The update function φ can then be defined by
2.3 Optimization Problem
The company earns a payoff of 1 if the customer accepts the recommendation and 0 otherwise. Since increasing the utility always increases the probability of purchase, the incentives of the customer and the company are perfectly aligned. It is always in the company's best interest to please the customer, since the more utility the company provides to the customer, the higher the payoff. The expected payoff of suggesting product Xj to a customer from segment Si isgiven by
where piA(Xj) is the probability of purchase as defined in (1).
If the company knows the customer's true segment, the problem of making recommendations reduces to always suggesting the product with the highest expected utility for that segment. This is a Markov chain model, which can be easily extended to other models such as those of Pfeifer & Carraway (2000) through state augmentation.
The problem of making recommendations is not as simple if the company does not know the customer's segment. The real decision is to find the best recommendation for each possible belief so as to maximize the expected revenue (or customer's utility). This policy, denoted µ* must solve the equation
where γ ∈ (0,1) is a discount factor and rt is the reward at time t. The set of possible beliefs is [0,1]|S|, which is uncountably infinite. This stands in sharp contrast with customer migration models, where the possible states of the system are defined by a finite (or countably infinite) set.
In the belief-state problem, the payoff function maps belief states and actions to revenues. Therefore, the payoff function is defined by
The three possible transitions from any given belief state are given by
where L corresponds to the state when the customer has left the system. Figure 3 depicts the dynamics of the belief-state problem for the two-segment case.
The optimal value function V*(b) = max E satisfies the Bellman equation
We know from (8) that b' can only take three possible values, namely φ(b, θA, X) , φ(b, θR, X), and φ(b, θL, Xt). Furthermore, since customers have no value after they leave V*(L) = 0.
Therefore, (9) can be written as
The dynamic programming mapping corresponding to the above value function is
This mapping is a monotone contraction under the supremum metric. Therefore, repeated application of the operator T to a value function V converges to the optimal value function (e.g., Bertsekas, 1995b). This result is the basis for the value iteration algorithm, which consists of repeatedly applying the operator T to the value function V1. Letting Vn = TnV, i.e., n applications of T to V1, then the algorithm is stopped when |V*(b) - Vn(b)| is within a tolerable error margin, which can be expressed as a function of |V*(b) - Vn-1(b)| (this is an application of a standard result found in Littman [1994] or Bertsekas [1995b], for example).
One important problem must be solved before value iteration can be used to find the optimal solution: there is an infinitely large number of beliefs. The continuity of the belief space could mean that there exists some n for which Vn cannot be represented finitely or that the value function updates cannot be computed in a finite amount of time. The next sections describes how these difficulties can be overcome in order to solve the agent's dilemma.
3 MODEL ANALYSIS AND SOLUTION
The value function after n iterations of the dynamic programming mapping is piecewise linear convex function and can be written as
Vn(b) = (b · α)
where An is a finite set of |S|-dimensional vectors. This implies that V*(b) can be approximated arbitrarily well by a piecewise linear convex function.
Proposition 1. The value function Vn(b), obtained after n applications of the operator T to the value function V1(b), is piecewise linear and convex. In particular, there exists a set An of |S|-dimensional vectors such that
Vn(b) = (b · α)
Proof. The proof is by induction, and therefore consists of the verification of the base case and of the induction step.
(1) Base case: V1(b) = (b · α).
(2) Induction step: If
∃An-1 such that Vn-1(b) = (b · α)
then
∃An such that Vn(b) = (b · α).
The statement above can be proved by verifying that the elements α ∈ An are of the form
where αi∈ An-1, αj ∈ An-1 and ΓA and ΓR matrices with elements
and
It then follows that
Vn(b) = (b · α)
which can be verified to be true by defining
The details of the proof are in Appendix A.1Appendix A.1.
Figure 4a shows an example of the convex hull of a hypothetical set An consisting of four vectors and Figure 4b depicts the corresponding value function Vn(b). Each vector corresponds to a different customization policy. On a more intuitive level, each component of the vector is the expected payoff the agent would receive given a sequence of recommendations and a customer segment. The optimal policy will be a function of the belief (represented by the slope of the dotted line in Figure 4a) and the set of feasible policies. Each piecewise-linear segment of the value function 4b corresponds to a different policy.
Theorem 1 provides the missing link to ensure that the value iteration algorithm is certain to arrive at the optimal solution for the finite-horizon problem and an arbitrarily good approximation for the infinite horizon problem in a finite amount of time. Unfortunately, this finite amount of time can be very large and often impractical. The difficulty lies in the efficient computation of the sets An since the size of these sets grows very fast with each step of the value iteration algorithm. In each iteration of the algorithm there is one new α vector for each possible product recommendation X for each permutation of size 2 of the vectors in An-1. Consequently, |An| = |X|2n-1.
The way in which the sets An are generated make it impossible to determine the optimal value function in closed-form, and the rate at which they grow can make numerical solutions impractical. Figure 5 show the convex hull of a sequence of sets An for increasingly large n. Fortunately, this property is shared by all partially observable Markov Decision Problems (POMDPs), and the important developments in the literature have generated methods to (i) make the sets An as small as possible so as to minimize the size of An+1 or (ii) find ways of quickly generating the sets An from sets An-1. Sondik (1978) made significant theoretical contributions to the understanding of the POMDP model and described the first algorithm for finding the optimal solution. Monahan (1982) formulated a linear program that finds the smallest possible set An that contains all the vectors a used in the optimal solution. Littman's (1994) Witness algorithm is one of the most important contributions to the generation of An from An-1. Applications in robot navigation resulted in a recent increase of interest in POMDPs in the artificial intelligence community (e.g., Kaelbling et al., 1996) which led to very important contributions in computational methods. Littman et al. (1995), for example, describe methods to solve POMDPs with up to nearly one hundred states. Tobin (2008) and Ross et al. (2008) provide a recent review of the state of the art in this field.
Figure 6 illustrates the results of numerical studies comparing the optimal policy µ*, defined in (6) and computed using the Witness algorithm, and the myopic policy µM, which satisfies
These results were obtained from simulations where companies started out with the same number of customers and gained no new customers over time. The graphs are typical of simulations done with varying numbers of customer segments and products. Graph 5(a) shows that the myopic policy is more likely to generate profits in the short-run, while the optimal policy does better in the long-run. The agent that acts optimally sacrifices sales in the beginning to learn about the customers to serve them better in the future. Graph 5(b) shows the cost of learning in terms of the customer base. The optimal policy leads to more customer defections due to low utility in the short-run, but the customers who stay receive consistently high utility in later periods and defect at much slower rates than customers being served by a myopic agent.
In spite of significant recent advances in computational methods, the optimal policy cannot always be computed in a reasonable amount of time. Hence the need for approximate solution methods that produce good policies in an efficient manner. If V*(b) can be approximated by (b), then the corresponding policy
(b) satisfies
There are several ways to approximating V*(b). Bertsekas (1995b) discusses traditional approaches, and Bertsekas & Tsitsiklis (1996) suggest alternative methods based on neural networks and simulations. The paragraphs that follow describe the Limited Learning (LL) approximation. This approximation is of interest because its corresponding customization policy yields good results and can be stated in simple terms which give insights into relevant managerial settings.
Limited learning policies are derived by assuming that the agent will stop learning after a fixed number of interactions. This restriction in the agent's actions implies that the same product will always be recommended after a given point. These approximations are very good in situations where most of the learning occurs in the early stages.
Proposition 2 derives an expression for the policy µLL(b) , which maximizes profits when agents are not allowed to change their beliefs between interactions.
Proposition 2. If agents are constrained to recommend the same product in all interactions with customers, then the infinite-horizon value function is given by
and the corresponding policy by
Proof. See Appendix A.2Appendix A.2.
The µLL(b) policy can be re-written as
µLL(b) = arg {b' · Λ (X) · pA (X)}
where Λ (X) is a diagonal matrix that can be interpreted as the inverse of the risk associated with each recommendation for each segment. There are many ways to choose the elements of Λ (X) . In the particular case of (18), the risk is fully captured by the probability of leaving. In a real world application, this would be an appropriate place to incorporate the knowledge of managers into the control problem. If all the diagonal elements of Λ (X) are the same, µLL reduces to the myopic policy. The matrix Λ (X) scales the terms piA(X) by a risk factor unique to each product/segment combination. Therefore, under µLL products that are very good (or very safe) for only a few segments are more likely to be chosen than products that are average for all segments. The opposite would be true with the myopic policy. Consequently, agents acting according to µLL observe more extreme reactions and learn faster.
The µLL(b) policy can be improved upon by allowing the agent to learn between interactions. In other words, the agent acts according to µLL(b) but updates the beliefs after each interaction. This idea can be generalized to a class of policies where during each period the agent solves a n- period problem and chooses the action that is optimal for the first period, in a rolling-horizon manner. More precisely,
In the specific case where n = 1 the corresponding policy is given by
Figure 7 shows the results of a numerical experiment designed to compare the performance of policies µLL(1) and µM, the myopic policy. This experiment consisted of simulating purchase experiences for two sets of 500 customers, each being exposed to one of the two control policies. Figure 7a shows the customer a base as a function of the number of interactions. The myopic policy takes less risks and performs better in the short-run, but agents using µLL(1) learn faster and are able to retain a higher percentage of customers after 4 or so interactions. The net result is that policy µLL(1) leads to a consistently higher customer base after 9 interactions. Figure 7b shows that the initial investment in learning by µLL(1) pays off in the long-run in the form of higher cumulative profits.
The policy µLL(N) consistently yields good results in empirical tests. The expression for VLL(b) can be combined with an upper bound based on the assumption of perfect information to compute performance bounds as a function of the belief state:
Note, first of all, that the upper and lower bounds are very close to each other in the extreme points of the belief space. The worst-case scenario happens when the company knows very little about the customer. In this case, however, we would expect the upper bound to be very loose, since it is based on the assumption of perfect information. This suggests that using the lower bound value function as a basis for control may yield good results even if the upper and lower bounds are not very close.
Figure 8 discretizes the continua of customer heterogeneity and product variety to explain why µLL(1) performed well in our simulations and is likely to perform well in real-world scenarios. The first column refers to the situation when there are few customer segments and they are all very similar. Customization is of little value in this situation because one standardized product should suit all customer segments equally well. The cases described by the first row are equally uninteresting. If all products are very similar, the agent will learn very little by observing customers over time. However, profits will not be affected because the limited product variety would prevent the agent from catering to specific tastes in the first place. Cell (4) accurately captures the situation where online customization is most valuable (and most commonly used): different customer segments and different products. Since customers differ in their reactions to recommendations, early observations are very informative. This is precisely the situation when the policy performs well, because it observes "extreme" reactions from different segments and learns fast, moving to one of the regions in the belief space where µLL(1) is more likely to coincide with the optimal policy.
We conclude this section listing five important properties of the µLL(1) policy.
1. Convergence to the optimal policy
As the belief state approaches the extreme points of the belief space, the upper and lower bounds are very close to each other. This implies that over time, as the company learns about the customer, the policy µ(LL)(b) will be the same as the optimal policy.
2. Lower the probability that the customer will leave after each interaction due to a bad recommendation.
This can only be guaranteed probabilistically because the observation error could theoretically be large enough to cause the customer to behave suboptimally. The likelihood of this event happening decreases as the utility of the recommendation increases, and the utility of the recommendations will increase over time.
3. Better revenues than the myopic policy.
When the company already knows the customer well, µLL(1) the myopic policy, and the optimal policy perform equally well. In situations where tthe agent needs to learn, µLL(1) loses to the myopic policy in terms of short-run revenue, wins in terms of expected revenues over time.
4. Learn faster than the myopic policy.
The myopic policy is more likely to suggest products that have similar utilities across all customer segments when the agent is not sure about the customer's true segment. This situation is likely to happen at the beginning of the relationship, and suggesting such products leads to little, if any, learning.
5. Ease of computation (or, better yet, closed-form solution).
The value function VLL(b) can be described in closed-form. Therefore, policies µLL(n) are very easy to compute and implement for small n.
4 DISCUSSION
4.1 Solving of the Agent's Dilemma
The main question of this paper is how companies should customize their products and services in settings such as the Internet. The analysis unambiguously shows that the pre-dominant paradigm of maximizing the expected utility of the current transaction is not the best strategy. Optimal policies must take into account future profits and balance the value of learning with the risk of losing the customer. This paper describes two ways of obtaining good recommendation policies: (i) applying POMDP algorithms to compute the exact optimal solution, (ii) approximating the optimal value function. The heuristic we provide worked well on the tests we performed, but we do not claim this heuristic to be the best one for all possible applications. Indeed, there are better heuristics for the solution of POMDPs with certain characteristics and the development of such heuristics for specific managerial settings is a topic of great interest to current researchers.
The bounds analyzed in the previous section make explicit why agents acting according to a myopic policy perform so poorly. These agents fail to recommend products that can be highly rewarding for some segments because they fear the negative impact that these recommendations could have on other segments. This fear is legitimate - companies can lose sales and customers can defect - but it can be quantified through a matrix Λ (X) that captures the risk associated with each possible recommendation for each segment. Optimally-behaving agents make riskier suggestions than myopic agents, learn faster, and earn higher payoffs in the long-run.
4.2 Learning and the Value of Loyalty
The more quality (utility) a company provides the customer, the more likely the customer is to come back. The better the company knows the customer, the more quality it can provide. Yet, learning about the customer can mean providing disutility (less quality) in the short-run. The interaction policy described in this paper reconciles these two apparently contradictory statements. The model suggests that the concept of loyalty must be expanded to incorporate learning. The extent to which repeated purchases lead to high value and loyalty depends on the company's ability to learn about their customers as they interact.
The benefits of loyalty to profitability have been abundantly discussed in the managerial literature. Reichheld (1996) gives the following examples of how loyalty leads to profits:
-
In an industrial laundry service, loyal customers were more profitable because drivers knew shortcuts that made delivery routes shorter and led to cost reductions.
-
In auto-service, if the company knows a customer's car, it has a better idea of the type of problem the car will have and will be able to identify and fix the problem more efficiently.
-
A shop that sells to the same group of people year after year saves on inventory costs because it can stock only the items its customers like.
These examples suggest an intimate relationship between learning and loyalty. In the laundry example, the fact that the customers have made a number of purchases from the same service provider has no direct effect on cost reduction. If a particular customer were to leave the company and its neighbor became a new customer, the cost savings would be the same in spite of the newness of the new customer. What seems to matter is knowledge, not length of tenure or number of previous purchases. Changes in profitability and retention rate over time depend on the firm's ability to learn from past interactions and customize its services so as to provide customers with more quality.
The observations of the previous paragraph are corroborated by the analysis of Section 3. Figure 9 reproduces and labels the graph of Figure 6b. When the curves intersect, both firms have a customer base of size N who have been customers for T interactions. Even though both customer bases might be assigned the same value by a number of metrics (e.g., size of customer base, length of tenure) the difference in knowledge acquired during the first T interactions makes an enourmous difference in future profits. A metric such as RFM (recency, frequency, monetary value) might even assign a higher value to customers of the myopic agent. The ability to offer a large variety of products to a heterogeneous customer population (cell 4 in Figure 8) is not sufficient to generate rising revenues over time. It is also necessary to learn over time and customize services accordingly. Reinartz & Kumar (2000) make no mention of learning about customer preferences and customized services in their study, and their conclusion is that profitability per customer does not increase over time. Reichheld & Schefter (2000) conduct their study in the context of the Internet, where customer information is easily gathered and customization is commonplace. Not surprisingly, they find that profits per customer rapidly increase over time and that the benefits of loyalty on the online world far exceed those of the brick-and-mortar world.
4.3 The Value of Knowing the Customer
The model presented in this paper shows how to quantify the value of learning about cus-tomers. Therefore, the optimal value function V*(b) can be interpreted as the lifetime value of a customer as a function of how well the company knows that customer. The fact that V*(b) is convex (proved in Theorem 1) has interesting managerial implications.
Consider, for example, a company operating in an industry where customers of Segment 1 are half as profitable as customers in Segment 2. Which marketing campaign would be most profitable: (i) one that only brings in customers from Segment 1 or (ii) one that brings customers from both segments with equal probability? The answer is that it depends on the shape of the value function. Figure 10 shows three possible value functions. If V*(b) = V1*(b), then campaign (ii) is better; if V*(b) = V3*(b), then campaign (i) is better. Both campaigns are equally profitable if V*(b) = V2*(b). The intuition behind this result is that sometimes it can be costly to learn about one's own customers. In some situations the company is better off knowing for sure that they are providing excellent value to the least desirable segment. The lower the switching cost and the higher the variance in the utility observation error, the more companies should invest in targeted advertising. This result provides an important connection between a firm's acquisition and retention policies: the Vi* curves, which guide the acquisition policy, are determined by the customization policy.
The more a company knows the preferences of a particular customer, the more it should want to learn even more about that customer in order to transform knowledge into cus-tomized, highly valued services. Intuitively, one may think the information is most valuable when the company doesn't know anything about the customer. The convexity of the value function implies the opposite. In qualitative terms, the change in the value of the customer as the company's level of knowledge changes from "very good" to "excellent" is greater than the change in value as knowledge goes from "average" to "good". This finding provides a theoretical justification for the empirical finding that the difference in loyalty between "very satisfied" and "satisfied" customers is much greater than the difference between "satisfied" and "slightly dissatisfied" customers (e.g., Heskett et al., 1994). If a company knows a customer well, that customer will receive consistently good recommendations and be very satisfied.
5 DIRECTIONS FOR FUTURE RESEARCH
The results obtained in this paper drew on previous research in Management Science and Consumer Behavior. Future research in this topic can be pursued in both these directions. From the Management Science perspective, the issue of the best way to implement web-based customization policies remains open. The value function approximations derived in this paper lead to a number of insights, but exact numerical methods generate better control policies. More work needs to be done to understand which approximation methods work best in situations where the problem is too large to compute the optimal policy. A second open question is how to recommend sets of products. In some situations agents are asked to recommend more than one product. One obvious way of addressing this issue is to consider each possible set as a single product and reduce the problem to the one solved in this paper. This method can be inefficient if the set of possible products is large, because of the exponential increase in the number of potential suggestions. Therefore, it is worthwhile to explore other ways to generate good policies.
From the Consumer Behavior perspective, there are two topics of interest. First, the de-velopment of efficient perception management devices to reduce the probability of defection. In some situations, it may be possible to have a "suggestion" to explore tastes as well as a "recommendation" to maximize utility. It has been shown that customers do not forgive bad recommendations, but could they forgive bad suggestions? Second, it is important to investigate how customers aggregate their experiences with agents over time. The model in this paper assumes that customer behavior is solely determined by the current product offering. In reality, the customers' perceptions of the agent's quality evolve over time. But how exactly does this evolution take place? On one hand, there is a stream of research which suggests that recency (the satisfaction derived in the last few interactions) is the most important determinant of current satisfaction (for a review see Ariely & Carmon, 2000). On the other hand, first impressions are important and it can be very difficult for a customer to change his or her initial perception of the agent's quality (e.g., Hogarth & Einhorn, 1992). These two views are in direct opposition, and it is not clear which is more important in web-based settings. Further research should also investigate how customers' expectations change as a function of the quality of the advice they receive.
Received April 25, 2012
Accepted May 10, 2012
A.1 Proof of Proposition 1
The proof is by induction, and therefore consists of the verification of a base case and the induction step.
(1) Base case: To prove that there exists a set of vectors A 1 such that
V1(b) = (b · α).
The optimal policy with 1 step to go is to act myopically with respect to immediate payoffs:
V1(b) = {
(b, X)} =
(b · α)
where the last equality holds by letting A1 = piA(X).
(2) Induction step
If
∃An-1 such that Vn-1(b) = (b · α)
then
∃ An such that Vn(b) = (b · α)
From the induction hypothesis, we can write, for θ ∈ {θA, θR},
where
αn-1(b, θ, X) = (φ(b, θ, X) · α).
Since Vn = TVn-1, we obtain Vn(b) by applying the mapping (TV) (b) to the Vn-1 function above:
Recall the definition of φ(b, θ, X) and note that φ(b, θA, X) and φ(b, θR, X) can be written as
and
If we substitute (b, X) =
bi · R(Si, Xj) and the expressions for φ(b, θ, X) above into the expression for Vn(b) we obtain
by cancelling out the terms Pr(θA|b, X) and Pr(θR|b, X) .
Factoring out the vector b and defining the set An as
we conclude that
Vn(b) = (b · α)
If agents are not allowed to learn, then the value function that maximizes future expected profits for the n-period problem is given by
where Â1 = A1 and the sequence of sets {Â1, Â2, ..., Ân} is defined by the recursion
where Γ(X) = ΓA(X) + ΓR(X) .
The value functions
n(b) can be explicitly written as maximizations over α ∈ Â1 as follows:
The sequence of functions
n(b) converges:
The last step follows from the fact that all the elements in Γ(X) are in (0,1) , and therefore [Γ(X)]n is a matrix of zeroes. Now consider the difference
Dn(b) = b · - Vn(b)
where
= arg
(b · [I - Γ(X)]-1 · [pA(X)]).
Substituting the full expression for Vn(b) yields
Since all the elements of Γ(X) are in (0,1) , the expression [I - Γ(X)]-1 is well-defined and can be expanded to
[I - Γ(X)]-1 = I + [Γ(X)]i.
Substituting the expansion above into the expression for Dn yields
It then follows that
The result from (20) ensures that the sequence Vn(b) converges to a limit V*(b) . Equation (21) asserts that Vn(b) also converges to b · . Since the dynamic programming mapping is a monotone contraction, the limit is unique, so
corresponds to the policy that maximizes the infinite horizon value function.
A.3 Simulation parameters
Parameters used for simulation in Figure 7:
c = 2.5
ε ~ Normal (0,3)
u1(1) = -2 u2(1) = 5
u1(2) = 5 u2(2) = -2
u1(3) = 0 u2(3) = 0
u1(4) = 3 u2(4) = 3
- [1] ARIELY D & CARMON Z. 2000. Gestalt characteristics of experienced profiles. Journal of Behavioral Decision Making, 13: 219-232.
- [2] ARIELY D, LYNCH JG & APARICIO M. 2004. Learning by collaborative and individual-basedrecommendation agents. Journal of Consumer Psychology, 14(1&2): 81-94.
- [3] BERGER PD & NASR NI. 1998. Customer lifetime value: Marketing models and applications. Journal of Interactive Marketing, 12: 17-30, (Winter).
- [4] BERTSEKAS D. 1995. Dynamic Programming and Optimal Control, Volume 1. Athena Scientific.
- [5] BERTSEKAS D. 1995. Dynamic Programming and Optimal Control, Volume 2. Athena Scientific.
- [6] BERTSEKAS D & TSITSIKLIS J. 1996. Neuro-Dynamic Programming. Athena Scientific, Belmont, MA.
- [7] DONEY PM & CANNON JP. 1997. An examination of the nature of trust in buyer-seller relationships. Journal of Marketing, 61: 35-51, (April).
- [8] GREENE WH. 2000. Econometric Analysis, 4th edition. Prentice Hall, New Jersey, U.S.A.
- [9] HESKETT JL, JONES TO, LOVEMAN GW, SASSER WE JR & SCHLESINGER LA. 1994. Putting the service-profit chain to work. Harvard Business Review. March-April, 164-174.
- [10] HOGARTH RM & EINHORN HJ. 1992. Order effects in belief updating: The belief adjustment model. Cognitive Psychology, 24: 1-55, (January).
- [11] JAIN D & SINGH SS. 2002. Customer lifetime value research in marketing: A review and future directions. Journal of Interactive Marketing, 16(2): 34-46.
- [12] KAELBLING LP, LITTMAN ML & MOORE AW. 1996. Reinforcement learning: A survey. Journal of Artificial Intelligence, 4: 237-285.
- [13] KOLLOCK P. 1999. The production of trust in online markets. Advances in Group Processes, 16: 99-123.
- [14] LEWICKI RJ, MCALLISTER DJ & BIES RJ. 1998. Trust and distrust: New relationships and realities. Academy of Management Review, 23, (July).
- [15] LITTMAN ML. 1994. The witness algorithm: Solving partially observable markov decision processes. Department of computer science, technical report, Brown University, Providence, R.I.
- [16] LITTMAN ML, CASSANDRA AR & KAEBLING LP. 1995. Learning policies for partially observable environments: Scaling up. In: PRIEDITIS A & RUSSELL S (Eds), Proceedings of the Twelfth International Conference on Machine Learning, 362-370. Morgan Kaufmann, San Francisco, CA.
- [17] MAES P. 1995. Intelligent software. Scientific American, 273(9): 84-86.
- [18] MCFADDEN D. 1981. Econometric models of probabilistic choice. In: Structural Analysis of Discrete Data with Econometric Applications, MANSKY CF & MCFADDEN D (Eds), MIT Press.
- [19] MEYER RJ & KAHN BE. 1990. Probabilistic models of consumer choice behavior. Handbook of Consumer Theory and Research, ROBERTSON T & KASSARJIAN H (Eds), Prentice-Hall.
- [20] MONAHAN G. 1982. A survey of partially observable markov decision processes: Theory, models, and algorithms. Management Science, 28(1): 1-15.
- [21] PFEIFER PE & CARRAWAY RI. 2000. Modeling customer relationships as Markov chains. Journal of Interactive Marketing, 12(2): 43-55.
- [22] REICHHELD F. 1996. The Loyalty Effect. Harvard Business School Press.
- [23] REICHHELD F & SCHEFTER P. 2000. E- loyalty: Your secret weapon on the web. Harvard Business Review, 78: 105-113, (July-August).
- [24] REINARZ WJ & KUMAR V. 2000. On the profitability of long lifetime customers: An empirical investigation and implications for marketing. Journal of Marketing, 64: 17-35.
- [25] ROSS S, PINEAU J, PAQUET S & CHAIB-DRAA B. 2008. Online Planning Algorithms for POMDPs. Journal of Artificial Intelligence Research, 32: 663-704.
- [26] ROSSI P, MCCULLOCH R & ALLENBY G. 1996. The value of purchase history data in target marketing. Marketing Science, 15(4): 321-340.
- [27] SONDIK EJ. 1978. The optimal control of a partially observable markov decision process over the infinite horizon: Discounted costs. Operations Research, 26: 282-304.
- [28] TOBIN. 2008. A Stochastic Point-Based Algorithm for Partially Observable Markov Decision Processes. M. Sc. Thesis, Faculté de Sciences et Génie, Université Laval, Québec.
- [29] TOUBIA O, SIMESTER DR & HAUSER JR. 2003. Fast polyhedral adaptive conjoint estimation.Marketing Science, 22(3): 273-303.
- [30] URBAN GL, SULTAN F & QUALLS WJ. 2000. Placing trust at the center of your internet strategy. Sloan Management Review, 42(1): 39-48.
- [31] WHITE III CC. 1976. Optimal diagnostic questionnaires which allow less than truthful responses. Information and Control, 32: 61-74.
- [32] ZAUBERMAN G. 2000. Consumer choice over time: The perpetuated impact of search costs on decision processes in information abundant environments. Ph.D. Dissertation, Duke University, Durham, NC.
Appendix A.1
Appendix A.2
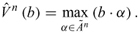
Publication Dates
-
Publication in this collection
24 May 2013 -
Date of issue
Apr 2013
History
-
Received
25 Apr 2012 -
Accepted
10 May 2012