Abstract
Increased heart rate variability (HRV) and high-frequency content of the terminal region of the ventricular activation of signal-averaged ECG (SAECG) have been reported in athletes. The present study investigates HRV and SAECG parameters as predictors of maximal aerobic power (VO2max) in athletes. HRV, SAECG and VO2max were determined in 18 high-performance long-distance (25 ± 6 years; 17 males) runners 24 h after a training session. Clinical visits, ECG and VO2max determination were scheduled for all athletes during thew training period. A group of 18 untrained healthy volunteers matched for age, gender, and body surface area was included as controls. SAECG was acquired in the resting supine position for 15 min and processed to extract average RR interval (Mean-RR) and root mean squared standard deviation (RMSSD) of the difference of two consecutive normal RR intervals. SAECG variables analyzed in the vector magnitude with 40-250 Hz band-pass bi-directional filtering were: total and 40-µV terminal (LAS40) duration of ventricular activation, RMS voltage of total (RMST) and of the 40-ms terminal region of ventricular activation. Linear and multivariate stepwise logistic regressions oriented by inter-group comparisons were adjusted in significant variables in order to predict VO2max, with a P < 0.05 considered to be significant. VO2max correlated significantly (P < 0.05) with RMST (r = 0.77), Mean-RR (r = 0.62), RMSSD (r = 0.47), and LAS40 (r = -0.39). RMST was the independent predictor of VO2max. In athletes, HRV and high-frequency components of the SAECG correlate with VO2max and the high-frequency content of SAECG is an independent predictor of VO2max.
Long-distance runners; Signal-averaged electrocardiogram; Maximal aerobic power; Mathematical model
Braz J Med Biol Res, February 2007, Volume 40(2) 199-208
The effect of an aerobic training program on the electrical remodeling of the heart: high-frequency components of the signal-averaged electrocardiogram are predictors of the maximal aerobic power
M. Marocolo1, J. Nadal1 and
Correspondence and Footnotes
P.R. Benchimol-Barbosa2
1Programa de Engenharia Biomédica, Coordenação dos Programas de Pós-graduação de Engenharia, Universidade Federal do Rio de Janeiro, Rio de Janeiro, RJ, Brasil
2Departamento de Cardiologia, Universidade Gama Filho, and Instituto Nacional de Cardiologia, Rio de Janeiro, RJ, Brasil
References
Correspondence and Footnotes
Correspondence and Footnotes
Correspondence and Footnotes
Abstract
Increased heart rate variability (HRV) and high-frequency content of the terminal region of the ventricular activation of signal-averaged ECG (SAECG) have been reported in athletes. The present study investigates HRV and SAECG parameters as predictors of maximal aerobic power (VO2max) in athletes. HRV, SAECG and VO2max were determined in 18 high-performance long-distance (25 ± 6 years; 17 males) runners 24 h after a training session. Clinical visits, ECG and VO2max determination were scheduled for all athletes during thew training period. A group of 18 untrained healthy volunteers matched for age, gender, and body surface area was included as controls. SAECG was acquired in the resting supine position for 15 min and processed to extract average RR interval (Mean-RR) and root mean squared standard deviation (RMSSD) of the difference of two consecutive normal RR intervals. SAECG variables analyzed in the vector magnitude with 40-250 Hz band-pass bi-directional filtering were: total and 40-µV terminal (LAS40) duration of ventricular activation, RMS voltage of total (RMST) and of the 40-ms terminal region of ventricular activation. Linear and multivariate stepwise logistic regressions oriented by inter-group comparisons were adjusted in significant variables in order to predict VO2max, with a P < 0.05 considered to be significant. VO2max correlated significantly (P < 0.05) with RMST (r = 0.77), Mean-RR (r = 0.62), RMSSD (r = 0.47), and LAS40 (r = -0.39). RMST was the independent predictor of VO2max. In athletes, HRV and high-frequency components of the SAECG correlate with VO2max and the high-frequency content of SAECG is an independent predictor of VO2max.
Key words: Long-distance runners, Signal-averaged electrocardiogram, Maximal aerobic power, Mathematical model
Introduction
Regular aerobic exercise training brings about beneficial changes in the cardiovascular system, with impact on individual and community health (1-3). The cardiovascular remodeling that follows aerobic fitness, reflected by both structural and functional changes of the heart, can be easily assessed by routine clinical examination. Mechanical remodeling following progressive upgrade in training level aiming at maximal aerobic power fitness is characterized by increased cardiac performance and a mild to moderate increase in left ventricular mass, and by increased contractile force of the cardiomyocyte in laboratory animal models (1,4-7). Mainly characterized by parasympathetic dominance, autonomic remodeling is characterized by decreased resting heart rate and increased heart rate variability (HRV) indexes. On the other hand, the electrical remodeling that occurs with both mechanical and autonomic remodeling is characterized by a redistribution of the activation time throughout the ventricular wall, which is expressed on the surface electrocardiogram (ECG) (4-7).
The presence of high-frequency components in the terminal region of the surface ECG has been described on athletes (6,8,9). The nature and the genesis of these components on the surface ECG in athletes, however, are still subject to investigation, and have been associated with both improvement of aerobic training level and incipient ventricular hypertrophy (6,8,9). Conventional methods aiming at estimating maximal aerobic power consist of exercise testing (either field or ergospirometry) usually lasting 12 to 25 min.
The objectives of the present study were: 1) to investigate HRV parameters and high-frequency content of the signal-averaged ECG (SAECG) as predictors of maximal aerobic power in trained athletes and healthy untrained subjects, and 2) to propose a mathematical model based on HRV and SAECG parameters to classify both trained and untrained healthy subjects according to maximal aerobic power.
Subjects, Material and Methods
The study protocol was in accordance with the principles of the Helsinki Declaration and was approved by the Ethics Committee in Clinical Research of the Laranjeiras National Institute of Cardiology, Rio de Janeiro, RJ, Brazil, and all subjects provided written informed consent before enrollment.
The study population was recruited from January to July 2004 and comprised 36 subjects divided into two groups: 18 trained long-distance runners (athletes) and 18 healthy untrained subjects (controls). The sample size used in the present study was calculated from numerical variables as described by Smith et al. (6) and Raineri et al. (10). From reference data, the sampling procedure was based on the difference of the 40-µV terminal (LAS40, ms) of at least 10 ms, with values of a = 0.05 and ß = 0.1, on the vector magnitude, filtered by a 4-pole bi-directional bandpass Butterworth filter with cut-off frequencies at 40 and 250 Hz, and using XYZ Frank orthogonal leads. The subjects participating in the present study were slightly younger but had similar anthropometric characteristics and gender distribution as those in the studies used as reference in the sampling procedure (6,10). Inclusion criteria were volunteers over 18 and less than 40 years old, in good mental and physical health, without a previous history of cardiovascular disease or systemic arterial hypertension. Subjects less than 18 and more than 40 years old, with a previous history of diabetes mellitus, thyroid dysfunction or liver disease, alcohol consumers, or tobacco smokers, or those currently taking any medicine were excluded.
Subjects had their maximal oxygen consumption (VO2max) estimated by the Cooper 12-min field test (Table 1), and calculated by the following equation (11,12):
where D is the distance achieved in the field test, expressed in meters, and VO2max is estimated as mL kg-1 min-1. Next, the resulting VO2max was divided by the constant 3.5 mL kg-1 min-1 to be converted into metabolic equivalents (METs). Control and athlete groups were separated according to VO2max as estimated by the Cooper test, arbitrarily defined as less than 11.5 METs for controls and more than 16.0 METs for athletes. The VO2max gap between groups was defined a priori with the purpose of enhancing eventual differences in SAECG parameters caused by conditioning level.
The athletes discontinued training 24 h before testing, and fasted for 4 h before signal recording. All subjects replied to a questionnaire about age, health condition, use of medication, and physical activities, and their height, weight, anteroposterior and laterolateral thoracic diameters were measured with the supervision of a primary care physician. The two groups were matched for age, gender, and body surface area calculated by a nomogram (Table 1) in order to minimize inter-group physiological and anthropometric variability (e.g., chest diameter) and to reduce the potential effect of thoracic geometry on the surface ECG signals.
12-Lead surface ECG acquisition
Each subject also had a 12-lead surface ECG acquired with a CardiMax electrocardiograph (Fukuda Denshi, Seattle, WA, USA) in resting supine position. ECG signals were analyzed for left ventricular hypertrophy and assessed by the voltage-duration product, as previously described (13). The voltage-duration product was considered to be abnormal if greater than 2440 mV/s, defining left ventricular hypertrophy.
ECG acquisition and signal averaging
SAECG was acquired shortly after application of the questionnaire and physical examination. Before a 15-min continuous signal acquisition, the subjects remained in the supine position for 5 min for stabilization of autonomic modulation after the change from the orthostatic position, thus preventing an "autonomic memory" in the acquired signals (14,15).
The ECG signals were acquired from the body surface using the modified bipolar Frank XYZorthogonal leads and an electrocardiograph amplifier model AEG03 (Lynx Tecnologia Eletrônica, São Paulo, SP, Brazil), with 10-GW input impedance, 120-dB/channel common mode rejection, and a 12-VDC power supply. Each ECG lead was amplified (gain = 1000), analogically filtered from 0.05 to 300 Hz by a 3rd order Butterworth filter, and converted into digital format by a data acquisition system with a 14-bit AD converter with a ±10-V dynamic range, and -1000 Hz/channel sampling frequency (Lynx Tecnologia). The ECG was digitized and immediately stored in the PC for off-line processing.
The skin was carefully prepared with a mildly abrasive pad and washed with alcohol for ECG acquisition, and 6 self-adhesive silver/silver-chloride electrodes were attached to the horizontal X-lead electrodes, placed on the right and left mid-axillary lines at the fourth intercostal space. The vertical Y-lead electrodes were placed on the middle clavicle line and second intercostal space and costal border, and the sagittal Z lead was placed anteriorly and posteriorly at the level of the 4th intercostal space just to the left of the midline. A seventh ground electrode was placed on the tenth rib on the mid-axillary line. The paired electrode-to-electrode impedance was measured and was less than 7 kW for all electrode pairs. ECG acquisition was carried out from 8 am to 1 pm in a quiet and air-conditioned environment, with a temperature of 25ºC. Digital data were processed with pattern recognition software to reject ectopic or excessively noisy beats (16,17). The coherent weighted averaging was carried out independently on each lead using a previously described and validated technique for R-wave alignment and was stopped at a final noise below 0.35 µV (16,17). The SAECG was analyzed in the time domain on the vector magnitude (Vm), defined by:
where X, Y and Z are the averaged orthogonal leads after 4-pole bi-directional Butterworth filtering, with band pass ranging from 40 to 250 Hz.
Four parameters were calculated from the vector magnitude (Figure 1): 1) duration of the signal-averaged filtered QRS complex (DUR, ms), 2) duration in the terminal region of ventricular activation with a magnitude below <40 µV (LAS40, ms), 3) the root mean squared (RMS) value of the potentials in the 40-ms terminal of the filtered QRS (RMS40, µV), and 4) the RMS value of the total filtered QRS (RMST, µV). Although terminal portion analysis has been used to identify the risk of ventricular arrhythmias, these parameters have been also tested as markers of cardiac fitness in athletes in general. SAECG was considered abnormal if two of three (DUR, RMS40, LAS40) parameters were outside the normal range (18).
Heart rate variability
The raw ECG was processed simultaneously on the X and Z leads to extract the series of normal RR intervals by rejecting ectopic or extremely noisy beats. The following parameters were thus calculated for the analysis of HRV: Mean-RR (ms), the average duration of RR intervals; RRmin (ms), the shortest RR interval value; RRmax (ms), the largest RR interval value; RMSSD (ms), the RMS standard deviation of the difference between two consecutive normal RR intervals. During the analysis of RR interval, rejected beats per acquired ECG ranged from 0.16 to 5.22% with a mean (± SD) of 0.66 ± 0.93%.
Statistical analysis
Continuous variables are reported as mean ± SD. SAECG (RMST, RMS40, DUR, LAS40) and HRV (RMSSD, mean-RR, RRmax, RRmin) parameters had their probability distributions assessed. The RMST and RMS40 variables showed asymmetric probability distributions and were normalized by natural logarithm transformation (Lnt) (18,19). The means were compared by the unpaired Student t-test. Categorical variables were compared by the Fisher exact test. Linear regression analysis using the Pearson correlation coefficient (r) was carried out between SAECG parameters and VO2max, as well as between HRV parameters and VO2max. The level of significance was set at a = 0.05 in all tests. Data were analyzed using the Statgraphics Plus 5.1 (Manugistic Inc., Rockville, MD, USA), MS Excel 2000 (Microsoft Corporation, Redmond, WA, USA), and Epi-Info 6.04 (Center of Disease Control, Atlanta, GA, USA) software.
Mathematical model
A multivariate stepwise logistic regression model was adjusted to significant HRV and SAECG variables in order to estimate VO2max (P < 0.05 in inter-group comparison). In the logistic model, variables with r² ³ 0.64 and hierarchically lower significance by the Wald test were considered to be co-variates and were excluded from the model (exclusion started with highest P value). The final model was achieved when all co-variates were excluded.
A binary variable Code, assuming a value of zero for controls and of one for athletes was defined as:
where P is given by:
where K is a fitting constant and Ai the regression constant of each Xi variable.
A decision-three algorithm based on the final variables of the model was built to stratify VO2max. The threshold cut-off levels of the final variables were detected by logistic regression model analysis, and were not included in the interval range as reference for higher VO2max estimates.
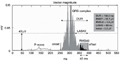
Vector magnitude parameters in the time domain. Onset and offset represent the limits of the ventricular activation, automatically detected (see text for details). For abbreviations see legend to
Figure 1. Vector magnitude parameters in the time domain. Onset and offset represent the limits of the ventricular activation, automatically detected (see text for details). For abbreviations see legend to Table 2.
[View larger version of this image (85K JPG file)]
Anthropometric and demographic characteristics of the subjects who participated in the study.
[View larger version of this table (150 K JPG file)]
Results
The voltage-duration product was not significantly different (1,676.1 ± 711 for athletes vs 1,325.0 ± 548.5 mV/s for controls) between groups. An electrical marker of left ventricular hypertrophy was detected in 3 subjects in the athlete group and in no subject in the control group (P = 0.23).
The HRV and SAECG parameters of each group and respective inter-group comparison are presented in Table 2. All variables demonstrated significant differences between the control and athlete groups, except for DUR, for equivalent numbers of coherentaveraged beats and final noise values.
Linear correlation analysis was carried out jointly on the control and athlete groups. Positive correlations were observed between VO2max and Lnt (RMST), and between VO2max and Lnt (RMS40) (r = 0.77, P < 0.001; r = 0.6, P < 0.001, respectively; Figure 2A and B). The correlation between LAS40 and VO2max was -0.393 (P = 0.018). These two parameters, Lnt (RMST) and Lnt (RMS40), also showed a positive correlation (r = 0.718, P < 0.001; Figure 2C). No correlation, however, was found between DUR and VO2max.
The prevalence of abnormal SAECG parameters in the control and athlete groups was 11 and 8.3%, respectively (P = NS). Both RMS40 and LAS40 were abnormal in one athlete and in three control subjects. DUR was within the normal range in all subjects.
Mathematical model
After excluding the significant co-variates, the variables included in the model were: LAS40, RMST, RMSSD, and Mean-RR. The model showed 88.9% positive predictive, 94.4% negative predictive, and 91.7% total predictive values, explaining 69.33% of total deviance (P < 0.001). RMST was the only independent predictor of VO2max (Table 3).
The threshold cut-off values of the variables involved in the classification of the highest VO2max were (according to the optimal dichotomization level as computed by logistic regression model): RMST > 120.9 µV, LAS40 < 28.1 ms, Mean-RR > 1003.8 ms, and RMSSD > 30.3 ms.
The decision algorithm that best defined physical conditioning with 72.2% sensitivity, 94.4% specificity, and 83.3% total predictive accuracy was (pseudo-code language):
IF "RMST > 120.9" THEN
IF "LAS40 < 28.1" OR "Mean-RR > 1003.8" OR "RMSSD > 30.3" THEN "Athlete"
ELSE "Control"
where {IF...THEN} pairs represent condition commands in hierarchical order, and quoted parameters the condition codes; {ELSE} command points to the final alternative, and {OR} command allows logical non-excluding association of condition codes. The quoted "Control" and "Athlete" refers to control and athlete groups, respectively.
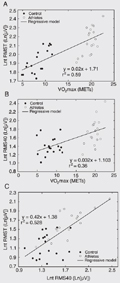
[View larger version of this image (151 K JPG file)
Signal averaged electrocardiogram and heart rate variability of control subjects and athletes.
[View larger version of this table (186 K JPG file)]
[View larger version of this table (188 K JPG file)]
Discussion
Aerobic training induces significant cardiovascular changes due to maximal aerobic power gain, and is reflected on both the electrical and autonomic properties of activation of the heart, as respectively detected by SAECG and HRV (1,20). Although Koutlianos et al. (21) have reported a significant correlation between HRV index and VO2max, to the best of our knowledge, the present study is the first to compare HRV parameters and high-frequency content of SAECG as predictors of VO2max in healthy sedentary subjects and athletes, and to show that the high-frequency content of the ventricular activation wavefront is an independent predictor of maximal oxygen consumption. The increase in VO2max caused by fitness results in a direct effect on ventricular mass (8,22,23) and increases ventricular activation voltage in the surface ECG (10,24). An increase in maximal aerobic power and myocardial mass is, therefore, expected to increase the total ventricular activation energy in SAECG.
Exercise training causes a well-established autonomic remodeling, which is characterized by a reduction in resting heart rate and an increase in HRV, representing a shift of the autonomic modulation toward parasympathetic over sympathetic dominance, as observed in healthy young and old subjects and in patients with ischemic heart disease (20,24). On the other hand, the duration of ventricular activation may be minimally affected by aerobic physical conditioning, as shown by Jordaens et al. (25), who did not find significant difference in QRS duration among four different groups comprising athletes with ventricular tachycardia and three control healthy groups: basketball players, cyclists and untrained controls. The RMS40, however, was remarkable and was greater in the control group than in the other groups (25). In the present study, in agreement with the findings of Jordaens et al. (25), no significant difference was observed between athletes and controls regarding the duration of ventricular activation, although other SAECG variables were significantly different (Table 2).
The QRS voltage-duration product derived from the 12-lead surface ECG has also been reported to predict left ventricular hypertrophy and is positively correlated with myocardial mass, as described by Okin et al. (26) and Ginefra et al. (27). On the other hand, Vacek et al. (28) applied SAECG to identify cardiac mass size and found a good correlation with echocardiography measurements. SAECG was shown to measure myocardial energy and to accurately assess left ventricular mass as well (28). In the present study, no significant difference between athletes and controls was found regarding QRS voltageduration product (P = 0.11), and left ventricular hypertrophy was found only in three athletes and in no controls (P = 0.23). However, a significant positive correlation was found between RMST (high-frequency ventricular activation energy) and VO2max as an effect of the conditioning level achieved. The decision algorithm based on the non-co-variate variables included in the multivariate model showed 83.3% total predictive accuracy, and provides a reference for predicting VO2max in trained athletes. Thus, a high-frequency content of SAECG seems to be able to identify the compensatory ventricular remodeling caused by aerobic training, undetectable by conventional electrocardiographic measurements (26). Electrophysiological, mechanical and histological remodeling of the heart, which includes re-arrangement of cardiomyocyte dimensions and orientation during mechanical remodeling, changes in fiber-to-fiber gap junction distribution, remodeling of potassium and calcium channels, and an increase in extracellular matrix, has been directly related to aerobic training, and, individually or in general, may influence the frequency content of the electrical wavefront propagation during normal ventricular activation, accounting for a very unique electrical environment in the trained heart of healthy athletes (7,29,30). In practice, the logistic regression model was suitably adjusted to maximal aerobic power data and further dichotomized both groups, enabling correct subject classification.
On the other hand, increased total high-frequency content energy (RMS area) and no abnormal prolongation of the ventricular activation in athletes without structural heart disease seem to represent a physiological aspect of electrical remodeling of the heart. Moroe et al. (31,32) found an 8.5% prevalence of abnormal SAECG in a total of 796 athletes evaluated. Athletes who performed anaerobic exercise tended to exhibit a higher incidence of abnormal SAECG, which was associated with a smaller left ventricular mass (31,32). A comparable prevalence of positive SAECG was observed in the present study (8.3%). In a general population, the prevalence of abnormal SAECG was very similar (19).
Significant differences in HRV parameters between sedentary and aerobically conditioned subjects have been established (20, 21,24,33), as well as the changes in the electrical properties of ventricular activation in both SAECG (1) and surface ECG (10,25). Thus, it is possible to speculate that regular aerobic exercise and a further increase in VO2max due to conditioning cause autonomic as well as electrophysiological changes in the heart, which are expressed as increased, HRV indexes and highfrequency content of the ventricular activation, respectively.
In the present study, SAECG and HRV time domain parameters were able to compose a predictive profile, accurately classifying trained and untrained healthy subjects according to maximal aerobic power. Further comments on conventional methods of assessment of aerobic fitness and the role of both SAECG and HRV analysis in this setting are necessary. The authors acknowledge that both ergospirometry and in-field exercise testing are standards for the assessment of physical conditioning. The ergospirometric test usually takes 12 to 20 min to assess aerobic conditioning, involves interpretation of complex data, and requires both trained personnel and expensive equipment, causing this approach to be limited to professional centers. In turn, in-field exercise testing is expected to assess athletes in real training conditions, and requires appropriate tracks which are frequently limited to professional groups and associations.
SAECG, on the other hand, has been originally conceived to evaluate the presence of high-frequency potentials located at the terminal QRS complex, universally accepted as a marker of pro-arrhythmogenic states (18,19). HRV analysis aims at assessing autonomic modulation of the heart, which, particularly in trained athletes, is associated with increased parasympathetic modulation directly related to aerobic conditioning (20,33). Both are simple noninvasive methods and have been able to accurately perform a classification of maximal aerobic power in trained and untrained subjects. They take a few minutes to be analyzed and potentially extend the clinical evaluation of healthy subjects undergoing training programs.
Limitations of the study
In the present study, maximum oxygen consumption was estimated by the Cooper method. Although the method appropriately estimated VO2max in the protocol applied to both groups, a direct ergospirometric assessment of VO2max was not carried out.
The present data were derived from a group of high-performance long distance runners, a fact that may represent a limitation when these data are extrapolated to other athletic modalities. This study assessed healthy subjects with very distinct maximal oxygen consumption in order to enhance the differences in high-frequency content of the ventricular activation due to the level of aerobic conditioning. A longitudinal study with untrained subjects admitted to an aerobic training program with regular follow-up of VO2max, echocardiographic measurements of cardiac structures, and periodic SAECG acquisitions will provide information on both the mechanical and electrical remodeling process during physical conditioning, and permit further insights into the development of high-frequency components of the ventricular activation.
Both HRV parameters and high-frequency electrical content of the ventricular activation assessed during resting conditions were increased in long-distance runners as compared to untrained healthy subjects, and accurately predicted maximal aerobic power. In particular, resting root mean squared voltage of the ventricular activation (RMST) of SAECG is an independent predictor of on-track maximal oxygen consumption. We conclude that the SAECG represents a potential method to predict the state of aerobic conditioning of trained athletes.
Acknowledgments
The authors wish to acknowledge the Federation of Athletism of the State of Rio de Janeiro for kindly referring trained athletes for the present study, and Dr. Ivan Cordovil and Dr. José Barbosa-Filho, from the Laranjeiras National Institute of Cardiology for their support.
Address for correspondence: P.R. Benchimol Barbosa, Departamento de Cardiologia, Universidade Gama Filho, Rua Pompeu Loureiro, 36/702, 20551-030 Rio de Janeiro, RJ, Brasil. Fax: +55-21-2255-1409. E-mail: ecgar@yahoo.com
Research partially supported by CNPq and CAPES. Received February 7, 2006. Accepted November 13, 2006.
- 1. Moore RL, Korzick DH. Cellular adaptations of the myocardium to chronic exercise. Prog Cardiovasc Dis 1995; 37: 371-396.
- 2. Welsh L, Kemp JG, Roberts RG. Effects of physical conditioning on children and adolescents with asthma. Sports Med 2005; 35: 127-141.
- 3. Ignone G, Giordano A, Tavazzi L. Effects of a short-term training programme in post-infarct patients with residual myocardial ischaemia. Eur Heart J 1988; 9 (Suppl M): 13-21.
- 4. Pelliccia A, Maron BJ, Spataro A, Proschan MA, Spirito P. The upper limit of physiologic cardiac hypertrophy in highly trained elite athletes. N Engl J Med 1991; 324: 295-301.
- 5. Sharma S, Maron BJ, Whyte G, Firoozi S, Elliott PM, McKenna WJ. Physiologic limits of left ventricular hypertrophy in elite junior athletes: relevance to differential diagnosis of athlete's heart and hypertrophic cardiomyopathy. J Am Coll Cardiol 2002; 40: 1431-1436.
- 6. Smith GS, Vacek JL, Wilson DB, Hawkins JW, Boyer TA. Exercise-induced alterations of signal-averaged electrocardiograms in marathon runners. Am Heart J 1989; 118: 1198-1202.
- 7. Barbosa EC, Barbosa PR, Bomfim AS, da Rocha PJ, Ginefra P. Precocious repolarization in athlete electrocardiogram: ionic basis and vectorial model. Arq Bras Cardiol 2004; 82: 103-107.
- 8. Warburton DE, McGavock J, Welsh RC, Haykowsky MJ, Quinney HA, Taylor D, et al. Late potentials in female triathletes before and after prolonged strenuous exercise. Can J Appl Physiol 2003; 28: 153-164.
- 9. Raineri AA, Traina M, Lombardo RM, Rotolo A. Relation between late potentials and echocardiographically determined left ventricular mass in healthy subjects. Am J Cardiol 1991; 67: 425-427.
- 10. Raineri AA, Traina M, Rotolo A, Lombardo RM. Quantitative analysis of ventricular late potentials in healthy subjects. Am J Cardiol 1990; 66: 1359-1362.
- 11. Cooper KH. A means of assessing maximal oxygen intake. Correlation between field and treadmill testing. JAMA 1968; 203: 201-204.
- 12. Cooper KH. Testing and developing cardiovascular fitness within the United States Air Force. J Occup Med 1968; 10: 636-639.
- 13. Gasperin CA, Germiniani H, Facin CR, Souza AM, Cunha CL. An analysis of electrocardiographic criteria for determining left ventricular hypertrophy. Arq Bras Cardiol 2002; 78: 59-82.
- 14. Lipsitz LA, Mietus J, Moody GB, Goldberger AL. Spectral characteristics of heart rate variability before and during postural tilt. Relations to aging and risk of syncope. Circulation 1990; 81: 1803-1810.
- 15. Ryan SM, Goldberger AL, Ruthazer R, Mietus J, Lipsitz LA. Spectral analysis of heart rate dynamics in elderly persons with postprandial hypotension. Am J Cardiol 1992; 69: 201-205.
- 16. Barbosa PR, Barbosa-Filho J, de Sa CA, Barbosa EC, Nadal J. Reduction of electromyographic noise in the signal-averaged electrocardiogram by spectral decomposition. IEEE Trans Biomed Eng 2003; 50: 114-117.
- 17. Barbosa PRB, Barbosa-Filho J, De Sá CAM, Nadal J. A new method for reduction of the muscular respiratory noise in signal-averaging based on the spectral analysis of the heart beat. Comput Cardiol 1999; 26: 323-326.
- 18. Barbosa PR, Sousa MO, Barbosa EC, Bomfim AS, Ginefra P, Nadal J. Analysis of the prevalence of ventricular late potentials in the late phase of myocardial infarction based on the site of infarction. Arq Bras Cardiol 2002; 78: 352-363.
- 19. Caref EB, Turitto G, Ibrahim BB, Henkin R, El-Sherif N. Role of bandpass filters in optimizing the value of the signal-averaged electrocardiogram as a predictor of the results of programmed stimulation. Am J Cardiol 1989; 64: 16-26.
- 20. Levy WC, Cerqueira MD, Harp GD, Johannessen KA, Abrass IB, Schwartz RS, et al. Effect of endurance exercise training on heart rate variability at rest in healthy young and older men. Am J Cardiol 1998; 82: 1236-1241.
- 21. Koutlianos NA, Kouidi EJ, Metaxas TI, Deligiannis AP. Non-invasive cardiac electrophysiological indices in soccer players with mitral valve prolapse. Eur J Cardiovasc Prev Rehabil 2004; 11: 435-441.
- 22. Warburton DER, Welsh RC, Haykowsky MJ, Taylor DA, Humen DP, Dzavik V. Effects of half ironman competition on the development of late potentials. Med Sci Sports Exerc 2000; 32: 1208-1213.
- 23. Peidro RM. Cardiologia del deporte: el corazón del deportista. Rev Argent Cardiol 2003; 71: 126-137.
- 24. Blumenthal JA, Sherwood A, Babyak MA, Watkins LL, Waugh R, Georgiades A, et al. Effects of exercise and stress management training on markers of cardiovascular risk in patients with ischemic heart disease: a randomized controlled trial. JAMA 2005; 293: 1626-1634.
- 25. Jordaens L, Missault L, Pelleman G, Duprez D, De Backer G, Clement DL. Comparison of athletes with life-threatening ventricular arrhythmias with two groups of healthy athletes and a group of normal control subjects. Am J Cardiol 1994; 74: 1124-1128.
- 26. Okin PM, Roman MJ, Devereux RB, Kligfield P. Electrocardiographic identification of increased left ventricular mass by simple voltage-duration products. J Am Coll Cardiol 1995; 25: 417-423.
- 27. Ginefra P, Barbosa EC, Barbosa PR, Bomfim AS, Boghossian SH, Salgado AA, et al. Detection of incipient left ventricular hypertrophy in mild to moderate arterial hypertension with normal electrocardiogram and echocardiogram: a new use for signal-averaged electrocardiography. Arq Bras Cardiol 2003; 81: 79-84.
- 28. Vacek JL, Wilson DB, Botteron GW, Dobbins J. Techniques for the determination of left ventricular mass by signal-averaged electrocardiography. Am Heart J 1990; 120: 958-963.
- 29. Kemi OJ, Haram PM, Loennechen JP, Osnes JB, Skomedal T, Wisloff U, et al. Moderate vs. high exercise intensity: differential effects on aerobic fitness, cardiomyocyte contractility, and endothelial function. Cardiovasc Res 2005; 67: 161-172.
- 30. Kemi OJ, Haram PM, Wisloff U, Ellingsen O. Aerobic fitness is associated with cardiomyocyte contractile capacity and endothelial function in exercise training and detraining. Circulation 2004; 109: 2897-2904.
- 31. Moroe K, Kimoto K, Inoue T, Annoura M, Oku K, Arakawa K, et al. Evaluation of abnormal signal-averaged electrocardiograms in young athletes. Jpn Circ J 1995; 59: 247-256.
- 32. Moroe K, Hiroki T. Signal-averaged electrocardiograms in young athletes - sudden death during exercise. Nippon Rinsho 1995; 53: 477-482.
- 33. Stein PK, Ehsani AA, Domitrovich PP, Kleiger RE, Rottman JN. Effect of exercise training on heart rate variability in healthy older adults. Am Heart J 1999; 138: 567-576.
Correspondence and Footnotes
Publication Dates
-
Publication in this collection
07 Jan 2007 -
Date of issue
Feb 2007
History
-
Received
07 Feb 2006 -
Accepted
13 Nov 2006