Abstracts
Most of the recent astonishing export growth in Brazil came from firms which already exported. The export base was not able to grow at the same pace. Since expanding the export base may have several positive effects on firms' productivity and size, in this paper we provide a four-level classification of Brazilian industrial firms according to their potential to export, and we identify a subset of non-exporting firms which are potential exporters. The effects of export stimulating policies may be improved by focusing more closely on these firms.
Export potential; export base; export promotion policies
O recente crescimento das exportações no Brasil se baseou fortemente naquelas firmas já exportadoras, e a base exportadora não conseguiu acompanhar o mesmo ritmo de crescimento. Considerando que expandir a base exportadora pode ter efeitos positivos sobre a produtividade e tamanho das firmas, neste artigo classificamos as firmas industriais brasileiras em quatro níveis de acordo com o potencial exportador, e especialmente identificamos um subconjunto de firmas que não exportam mas que são potenciais exportadoras. Os efeitos das políticas de promoção às exportações podem ser maximizados se elas forem focalizadas nestas firmas.
Potencial exportador; base exportadora; políticas de promoçãoàs exportações
Export potential of Brazilian industrial firms
Bruno César Pino Oliveira de AraújoI; Donald Matthew PiantoII
IResearcher at Ipea, Brasília, bruno.araujo@ipea.gov.br, SBS - Quadra 1 - Bloco J - Ed. BNDES - Sala 1114 70076-900 - Brasília - DF
IIProfessor, Department of Statistics, University of Brasília, dpianto@unb.br, Campus Universitário Darcy Ribeiro, 70910-900 - Brasilia - DF
ABSTRACT
Most of the recent astonishing export growth in Brazil came from firms which already exported. The export base was not able to grow at the same pace. Since expanding the export base may have several positive effects on firms' productivity and size, in this paper we provide a four-level classification of Brazilian industrial firms according to their potential to export, and we identify a subset of non-exporting firms which are potential exporters. The effects of export stimulating policies may be improved by focusing more closely on these firms.
Keywords: Export potential, export base, export promotion policies
JEL classification: C40, F14, F15
RESUMO
O recente crescimento das exportações no Brasil se baseou fortemente naquelas firmas já exportadoras, e a base exportadora não conseguiu acompanhar o mesmo ritmo de crescimento. Considerando que expandir a base exportadora pode ter efeitos positivos sobre a produtividade e tamanho das firmas, neste artigo classificamos as firmas industriais brasileiras em quatro níveis de acordo com o potencial exportador, e especialmente identificamos um subconjunto de firmas que não exportam mas que são potenciais exportadoras. Os efeitos das políticas de promoção às exportações podem ser maximizados se elas forem focalizadas nestas firmas.
Keywords: Potencial exportador, base exportadora, políticas de promoçãoàs exportações
1 Introduction
Even with the notable increase in Brazilian exports over the past few years, the export challenge persists. As noted by Pinheiro (2002), in order to maintain external sustainability, large surpluses in the trade balance will be necessary, given the prospects of growing deficits in the balance of services.
The recent growth of exports in Brazil since 2002 was largely based on those firms which already exported. The export base was not able to grow at the same pace. Indeed, while Brazilian exports grew 149.5% in only 4 years (they were approximately US $ 60 billion in 2002 and in 2006 amounted to US $ 130 billion), the export base in fact decreased from firms to 14 360.1 1 However, both estimates of the export base are likely underestimated since they do not take into account courier exports, such as the Exporta Fácil program. This may be the preferred export medium for micro and small enterprises, because of its simplicity. Expanding the export base may have several positive effects over the economy, since Brazilian entrants into foreign markets become from 1.1% to 23.7% more productive than comparable non-exporters and they also enhance their turnover by about 50% and employ 21% more workers in the first year after becoming exporters (Araújo 2006). These positive effects are associated with the newcomer's better access to imported inputs and equipment, greater exposure to competition and the possibility of technological cooperation with other firms in the productive chain (Aw & Hwang 1995, Clerides et al. 1998). In fact, the results for Brazilian industrial firms are in line with international evidence, which shows that productivity and size gains derived from foreign trade are more likely to occur in developing countries.
Even so, there is a certain discomfort from the evidence of the "failure of the export promotion agencies" during the early 1990s. Traditional export promotion policies had a small impact on total exports and, when successful, their effect rarely lasted (Gusso et al. 2004). Given this result, the policy recommendation was to increase the volume exported by already exporting firms rather than increase the export base (Pinheiro & Moreira 2000, Markwald & Puga 2002).
But the agencies' "failure" needs to be put into the context of rapid changes in the structure of trade, especially given the increasing global integration of production chains and the resulting increased importance of intra-industry and intra-firm trade. In this context, the few large international players gain great importance. In a way, this inhibits, at least in part, the effects of actions such as product diffusion and participation in commercial fairs and missions. Moreover, it explains why the export increases come primarily from the firms that already export, given that they have greater chances of being integrated into the production chains.
This scenario presents new challenges for the formulators of export promotion policies. Firstly, we defend the necessity of such policies, even if in new forms. Also, in the near future it will become necessary to increase the export base in order to increase export volume since firms which already export cannot increase their exports indefinitely.
Secondly, regardless of the form that they take, the costs of such policies require an increasingly well defined focus. Herein lays the main contribution of this article. Non-exporting firms are not a homogeneous group. The traditional exporter versus non-exporter dichotomy gives the impression that it is very difficult to make any non-exporter firm enter into the international markets. The fact is that the group of non-exporter firms is predominantly made up of low-performance firms such that, on average, it truly is difficult for them to engage in foreign trade. However, if we consider that there exists a subset of non-exporter firms which are "on the brink" of exporting, the short run impacts of export base expansion policies may increase if they are focused on this subset.
In this sense, the goals of the present work are: (i) elaborate a classification of Brazilian industrial firms according to their export potential, surpassing the traditional exporter/non-exporter dichotomy, and (ii) characterize the resulting groups. This classification results from an initial effort to find non-exporter firms with high export potential.
Intuitively, we denote as potential exporter firms those non-exporter firms which present similar characteristics to those of exporting firms. However, there still remains a difficulty: how can we compare a large number of different indicators simultaneously? The solution we have employed is the use of propensity score matching based on a probit model for the probability of exporting. Even though the technique is applied in its usual form, the economic significance of the treatment and control groups and of the results are non-traditional.
The remaining text is organized in the following manner. The following section presents the data. In the third section, details of the methodology and matching algorithm are presented. In the fourth section, the results of the probability model and the export potential classification are presented. In section five we characterize the different groups. Concluding remarks are in the sixth and final section.
2 Data
The data used are the result of the Instituto de Pesquisa Econômica Aplicada's (IPEA, the Institute for Applied Economics Research) effort to merge several different databases: the Pesquisa Industrial Anual (PIA, the Annual Industrial Survey) and Pesquisa de Inovação Tecnológica (PINTEC, the Survey of Technological Innovation) both from the Instituto Brasileiro de Geografia e Estatística (IBGE, the Brazilian Institute of Geography and Statistics); a foreign trade database from the Secretaria de Comércio Exterior (SECEX, the Secretary of Foreign Trade) of the Ministério do Desenvolvimento, Indústria e Comércio exterior (MDIC, the Ministry of Development, Industry, and Foreign Trade); the Relação Anual de Informações Sociais (RAIS, the Annual Report of Social Information), from the Ministério do Trabalho e Emprego (MTE, the Ministry of Labor and Employment); and the Censo de Capitais Estrangeiros (CEB, the Census of Foreign Capital) from the Banco Central do Brasil (BACEN, the Central Bank of Brazil). We do not physically possess this data and, with the cooperation of IBGE, all the statistical procedures strictly followed confidentiality rules. Data are the same as that in and the comments made therein about the data apply to this work as well.
All data refer to the year 2000, to industries with CNAEs from 15 to 36 (Classificação Nacional de Atividade Econômica, the National Classification of Economic Activities, the Brazilian equivalent to SIC, the Standard Industrial Classification), and to the certain extract (extrato certo) of the PIA. The certain extract of the PIA contains all the firms which employ 30 or more employees the year before the survey.
Of all the surveys cited above, the PINTEC is the only one that is not a census of the firms from the certain extract. Since technological innovation is a rare phenomenon, the sampling scheme of the PINTEC is biased towards innovating firms. However, IBGE calculates expansion factors for each firm in the PINTEC such that statistical inference is not compromised. Given the quality of these expansion factors and the fact that in giving up the PINTEC we would lose important information about technological determinants of foreign trade, we chose to perform the analysis both with and without the inclusion of the variables from the PINTEC. However, all presented results refer to the analysis without the inclusion of the PINTEC.
The year 2000 was chosen both because it is the reference year for all quantitative information from the PINTEC (the qualitative information refers to the period 1998-2000) and because of the ability to analyze both the entrance into (1997-1999) and exit from (2001-2003) foreign trade according to firm's export potential.
The sample used to classify the firms, excluding the PINTEC, contains 21 890 companies which, despite representing only 18% of all Brazilian industrial firms, represent 73% of the employment, 88% of both the total revenue and the industrial transformation value (a proxy for value added), as well as 89% of Brazilian industrial exports.
2.1 Differences between exporters and non-exporters
From the data, we can already establish, for the certain extract, differences between exporters and non-exporters.
The comparison is found in Table 1. From the total of 21 890 firms, 6492 are exporters (29.7%), for whom 11.6% of their revenue come from exports. One can clearly see the traditional dichotomy between exporters and non-exporters: the non-exporters are, on average, smaller, less efficient, employ workers with less education, and innovate less than the exporters. Actually, Ellery Jr. & Gomes (2005) claim that foreign trade data at the firm level confirm, for the Brazilian case, the stylized facts identified by Tybout (2003): exporters are in the minority, are more productive, and tend to export a small portion of what they produce. The variables chosen for this comparison will be described in greater detail when the probabilistic model is presented.2 2 As well as Ellery Jr. & Gomes (2005), the reader in search of a more detailed characterization of Brazilian exporters is encouraged to consult the work of De Negri (2004).
3 Methodology
Intuitively, we can define potential exporters as non-exporters which have similar characteristics to those of exporters. However, knowing that international competitiveness depends on various factors, how can we compare all these factors simultaneously? In a certain way, it will be necessary to represent all these factors in a single scalar such that firms with scalars sufficiently close have similar levels of competitiveness.
The chosen technique is propensity score matching (PSM). This technique is usually applied in quasi-experiments to evaluate social programs (such as programs providing a minimum income, job training, or job placement) .3 3 In relation to natural and quasi-experiments in economics, see Meyer (1995) . The principal objective of PSM is to perform ex-post counter-factual evaluations, in order to respond to the question: "what would have happened if those who received a given treatment had not received the treatment?" In other words, "what is the average treatment effect?"4 4 For a discussion of the average treatment effect, see Wooldridge (2002). For the case in which the treatment is applied randomly within a given sample (if we had a natural experiment), this question is easily answered: simply test the difference of means of the variable supposedly affected by the treatment between the cases (treatment group) and controls (control group) .
However, in economics the distribution of a treatment within a sample generally is not random. Hence, the cases and controls may be very different. PSM is a form of generating a counter-factual in these situations, based on a probabilistic model, by creating subsets of the original cases and controls which are sufficiently similar to be compared. An individual's treatment status is regressed on the supposed determinants of that treatment and only cases and controls with estimated probabilities sufficiently close to each other are retained. This estimated probability of treatment is the scalar we were searching for above.
3.1 The matching algorithm and export potential
As mentioned above, our question is different from the one for which PSM was originally proposed. Even though PSM has been used to test a causal relation between exporting and productivity, 5 5 Girma et al. (2004) use PSM in its traditional form with this goal. They define that fact that a firm exports as a treatment and accompany exporters and non-exporters over time, applying a differences in differences approach (see Meyer (1995)). here we use the technique for another purpose.
The probability model condenses several firm characteristics into a scalar. Afterwards, these scalars are used to match non-exporters and exporters. Let (Xj) be the probability of exporting for exporter, j, with characteristics Xj. We denote firm i as a potential exporter if its estimated probability of exporting,
(Xi) , lies within a small interval centered at
(Xj) and is the closest to
(Xj) for non-exporters. The idea is that, if the model is well specified, the potential exporters and their matches will have similar characteristics, X.
More formally, we know that, for the probit model, (Xj) = Φ(Xj
) where Φ(x) is the standard normal distribution function, Xj is a row vector of export probability determinants for firm j, and
is a column vector of estimated coefficients. Since the effect of PSM is to make
(Xj) ≈
(Xi), we also have that Φ(Xj
) ≈ Φ(Xi
). In this way:
The interpretation of the above expression is the following: (i) either the potential exporters have characteristics very similar to their matches, or (ii) even though some characteristics and are distinct, these differences, weighted by the
k , compensate each other. As will be demonstrated later, our results favor the first interpretation. The use of PSM to find potential exporters presents a methodological advantage over other alternatives, such as defining potential exporters as non-exporters with (X) > 0.5. The first advantage is that the cutoff point is arbitrary: why 0.5 and not some other value? The second advantage is that PSM makes it possible to identify the "hidden export champions" (Wagner 2002), which a probability cut would likely miss. This can be illustrated in the following form. Suppose that the only export probability determinant was the size of the firm, in a linear form, and that this relation is positive. Hence, if we established a probability cut we would implicitly have established a cut in firm size. In this way, we would eliminate all firms below a certain size from being potential exporters, even though we know that there exist many smaller companies with excellent levels of international competitiveness.
After the application of the matching algorithm, as well as the potential exporters and their matches there remain two other types of firms: non-matched exporters and non-matched non-exporters. What is interesting is that all these groups, not just the matched firms, have an economic meaning.
If the model is well specified, the distribution of (X) will be skewed to the left for the non-exporters and to the right for the exporters. Hence, the non-matched non-exporters, having characteristics distinct from any exporter, are firms with low levels of export potential and international competitiveness. Analogously, non-matched exporters have the highest levels of export potential and international competitiveness, with characteristics distinct from all non-exporters.
Hence, we have a four-level classification for export potential:
Level 1:
Firms focused only on the domestic market (non-matched non-exporters);
Level 2:
Potential exporter firms (matched non-exporters);
Level 3:
Matched exporters; and
Level 4:
Singular exporters (non-matched exporters).
4 The probability model and the classification of export potential
4.1 The probability model
It is worth noting that the quality of this classification depends on the probability model. According to economic theory, the relative productivity of a firm, relative use of factors, size of the firm and efficiency of scale, as well as technological factors, determine a firm's participation in foreign trade. However, one must pay close attention to the difficulty in constructing firm level variables to test the predictions of some of these theories (notably the Hecksher-Ohlin theory), whose foundations are essentially macroeconomic. For this reason, caution is necessary when constructing these variables and interpreting the results, given that there may exist various ways to do so. Even so, there is a growing body of literature concerned with the determinants of foreign trade at the firm level, on which we based our modeling choices.6 6 For a detailed survey of this literature, see Araújo (2005).
The probit probability model used for matching has as its binary dependent variable whether or not a firm exported during the year 2000, as determined by SECEX.
We can divide the export probability determinants into six groups:
Productivity: this determinant is related to Ricardo's theorem. Using the PIA, we define productivity as the industrial transformation value divided by the average number of employees in 2000.
Factor intensity: this determinant is related to the Hecksher-Ohlin theorem. In order to capture the intensity of the use of capital, we use as proxy the electricity spending per worker from the PIA. The proportion of qualified workers, defined as the percentage of workers who have completed primary school (taken from the RAIS), captures the intensity of use of human capital.7 7 However, we recognize that these proxies are imperfect, such that the Heckscher-Ohlin theorem is not being tested.
Production Scale (Size): larger firms are more capable of taking risks and overcoming the fixed entry costs of exporting (Wagner 2002). In addition, exporting requires a production scale which is often incompatible with smaller firms. For this reason, we expect that the production scale of a firm is positively associated with its probability of exporting. We represent the production scale of a firm by its size as measured by the number of employees. We constructed seven size categories:
Category 1: from 1 to 30 employees;
Category 2: from 31 to 50;
Category 3: from 51 to 100;
Category 4: from 101 to 250;
Category 5: from 251 to 500;
Category 6: from 501 to 1000;
Category 7: 1001 or more.
Efficiency and Returns to scale: in addition to production scale, the possibility of taking advantage of increasing returns to scale through foreign trade (marginal decreases in unit cost due to marginal increases of firm size) is estimated by Data Envelopment Analysis (DEA), as applied in De Negri (2003). DEA allows the estimation of a deterministic production frontier with varying returns to scale based on an output and inputs (in this case, industrial transformation value as a function of the average number of employees and electricity spending). A firm's efficiency is measured relative to this frontier. A firm has three possibilities for returns to scale; it can be situated in the region of increasing, constant, or decreasing returns to scale (scale categories 1, 2, and 3, respectively). These possibilities are captured by indicator variables (dummies).
Technological determinants: innovation variables we include from the PIA are: spending on marketing as a fraction of sales revenue, in a quadratic form (Willmore 1992, Ozçelik & Taymaz 2001), and a dummy indicating whether or not the firm invested in technological training in 2000.8 8 The innovation variables from the PINTEC are if the firm introduced a new product for the company or for the market, or adopted a process that is new for the company or the market .The PINTEC also contains spending on Research and Development (R&D). Nevertheless, these PINTEC variables were not employed to estimate the probability model, since they are available only for a subset of firms.
Other determinants : to capture the importance of foreign control on export probability, we use an indicator of foreign participation greater than 50%, according to the CEB. The international literature also suggests other determinants, such as the age of the firm (as a proxy for past competitiveness), and the degree of competition in the sector (HHI, CR). Since we do not know when each firm opened, we use the maximum number of months that any current employee has been at the firm as a proxy. To capture the degree of competition in the sector we use the market share of the four largest firms (CR4) within the three digits CNAE. Both of these variables are included with a quadratic specification. Sector and state dummies are also included into the probability model.
We recognize that these independent variables may be endogenous in the regression on the export dummy. For instance, due to learning-by-exporting effects, not only will the most productive firms be more likely to become exporters but also exporters may become even more productive, as pointed out in Araújo's (2005) survey. In this case, the effect of endogeneity will be to match an exporting firm with a non-exporter whose productivity is even higher than the exporter's pre-export level. This is useful, since our goal is to find potential exporters and not to identify the structural parameters which relate the independent variables to the probability of exporting. The results of the model can be found in Table 2.
Practically all the variables in the model present the expected signs. Productivity, the capital/labor ratio, the size of the firm, and multinationality are positively related with a firm exporting in 2000. Firm size may present a slight inflection for firms with more than 1000 employees; however, we did not test if the coefficients are statistically different. These results are consistent with existing microeconometric studies of Brazil.
One must cautiously interpret the results corresponding to the technological proxies, given that we definitely are not testing the relation between innovation and exports. The signs for the linear and quadratic terms of marketing/sales (positive and negative, respectively) imply an inverted "U" relation with a maximum probability of exporting at 13%, even though this variable may be related to the degree of competition in the sector. Technological training (which certainly serves as a proxy for innovation) positively affects the probability of a firm exporting.
In this paragraph we discuss the results obtained when the technological variables from the PINTEC are included in the model (these results are not reported). We find that innovation has great importance, especially product and process innovation for the market. The exception is process innovation for the firm. This variable may reflect the effort a firm exerts to keep up with technical change in its sector, without generating competitive advantages in international markets. The signs for the quadratic specification of R & D spending (positive for the linear term and negative for the square term) reveal that the probability of exporting follows an inverted "U" as R & D spending is increased, with a maximum near 5% of the industrial transformation value.
We return to discussing the results in Table 2. More efficient firms have a higher probability of exporting and the fact that a firm is located in the region of increasing returns to scale does not affect its probability of exporting relative to firms with constant returns to scale (this is the only insignificant variable in the model). Actually, being in this region indicates an inefficiency of scale, since the firm could increase its production scale thereby lowering its unit cost. One way of increasing a firm's size could be through foreign trade (Helpman, 1984). Similarly, the fact that a firm is in the region of decreasing returns to scale indicates that there is no more advantage to be gained by growing larger. Hence expected signs for these variables in the model are negative for increasing returns and positive for decreasing returns. The explicit inclusion of efficiency in the model may be the cause of the insignificance of increasing returns to scale. In Davis & Weinstein (2003) our expected result is found in both tobit and probit models, although the authors interpret it as a puzzle. Finally, the results suggest a "U" relation between both firm age and the probability of exporting and competitiveness of the sector and the probability of exporting.
4.2 The classification of export potential
We implemented PSM by using the "greedy" algorithm in SAS.9
9
The algorithm is available in Parsons (2001).
This algorithm matches pairs of firms according to a specified precision. For example, a two digit match means that an exporter with (X) = 0.5674 could be matched with a non-exporter with
(X) = 0.56####, with #### representing arbitrary digits. In this way, the difference between matched probabilities is no larger than 1%. In a three digit match this same firm would be matched with a non-exporter with
(X) = 0.567###, and so on.
The principal advantage of this algorithm is its computational simplicity, which is extremely important when working with samples of thousands of observations. The algorithm was applied successively with an initial match precision of six digits. The algorithm was then reapplied, at five digits, to those firms which were not matched. This continued until we arrived at a two digit match. After each pass, the matched firms are removed from the sample. If more than one non-exporter exists, which can be matched to an exporter, the match is performed randomly.10 10 The literature on causal inference of treatment effects includes other matching algorithms, such as kernel matching and radius matching. These algorithms exhibit a trade-off between bias and variance as the number of matches per treated firm is increased. In our case, bias is more important so we employ a kind of "nearest neighbor" 1:1 algorithm. However, one can extend the definition and find more potential exporters using, for example, an 1:N matching algorithm, but this will increase the bias and provide worse estimators for our purpose.
This process yielded 5 pairs at 6 digits, 72 at 5 digits, 587 at 4 digits, at 3 digits, and at two digits. After the application, firms were classified with level 1 export potential (the majority, as expected), firms each with levels 2 and 3 and firms were considered singular exporters (level 4). Table 3 presents descriptive statistics for the estimated export probability of each group.
The matching algorithm applied here does not force matches to be in the same sector and state, because if this were the case, it wouldn't be possible to estimate a probit in most cases. However, the great advantage of PSM is that the propensity score condenses all the relevant export determinants (among them sector and state) into a scalar.
We verified the percentage of intra-sectorial and intra-regional matches. Matches were considered intra-sectorial when firms from sectors with the same technological intensity, according to the UNCTAD classification, were matched.11 11 The bridge table from CNAE to the UNCTAD classification (primary commodities, labor and natural resource intensive sectors, low, medium and high technological intensity) is available in Chudnovsky, López and Orlicki (2005) . Similarly, when the matched firms were from the same macro-region (North, Northeast, Center-West, South, and Southeast), matches were considered intra-regional. The fraction of intra-sectorial matches decreases as the estimated export probability increases. However, in these cases, endogenous factors such as firm productivity and size assume a more dominant role than the sector in determining the probability of exporting12 12 The proportion of intra-sectorial matches when ( X) < 0.5 was 47%, whereas when ( X) > 0.5 this proportion was approximately 30%. . The proportion of intra-regional matches remained constant (around 70%) as a function of export probability.
5 Characterization of the firms by export potential
5.1 Similarities and differences between the categories
The histograms in Figure 1 have already provided indications that the potential exporters (level 2) and their matched exporters (level 3) have quite similar characteristics. However, as discussed in Section 3, the similarity of the export probabilities of the level 2 and 3 firms may not come about because of similarities between the firms, but rather because differences in characteristics compensate each other.
Fortunately, this is not the case. We demonstrate this by performing tests of means for the quantitative variables and distributional tests for the qualitative variables. Formally speaking, this is the balancing condition test. The means and the tests of the equality of means can be found in Table 4.
In the literature on causal inference of treatment effects, the balancing condition refers to the similarity of treated and control groups before the treatment. Reaching the balancing condition is important because this is an indication that selection bias is being controlled for, since firms in the control group have characteristics that are very close to the treated firms before the treatment.
In our case, the equality of means of the quantitative variables was tested by using ANOVA and Tukey tests. Despite assuming normality for the distribution of the variables, these tests are robust to violations of this hypothesis in large samples. In addition, there exists bias towards rejection of the null hypothesis for non-parametric tests in large samples. While ANOVA tests equality of means simultaneously between all four levels, Tukey tests equality between 2 or 3 groups. ANOVA rejected the null hypothesis of equality of means at 1% for all the quantitative variables, while the results of the Tukey test at 5% are reported in Table 4.
One can observe that groups 2 and 3 present equivalent means for almost all of the quantitative variables, with the exception of average monthly wage and number of employees. Even variables which were not considered in the probabilistic model have equal means for the potential exporters and their matches, such as average employee education, total sales, and industrial transformation value. If we compare the firms which were sampled in the PINTEC, we find that Internal R & D/Revenue also presents equal means for levels 2 and 3. These results demonstrate the robustness of our matching algorithm in discovering potential exporters.
As far as the exceptions are concerned, the first suggests the existence of a wage premium for exporting firms - which is not verified in where firms which begin exporting are compared with similar non-exporting firms; the second exception seems to reinforce the importance of firm size as a determinant of exports.
Whereas levels 2 and 3 stand out because of their similarity, level 1 is notable for its low performance indicators, with the opposite occurring for level 4. An emblematic case is the productivity: the singular exporters present average productivity almost 2.3 times as large as that of the matched exporters, while the firms directed toward the domestic market reach half the productivity of the potential exporters. This demonstrates that both the exporters and non-exporters are not characterized by homogeneity.
The same pattern of differences between the groups persists for the qualitative variables. Table 4 also presents the distribution of these variables among the groups. One sees that the distributions for levels 2 and 3 of export potential are also quite similar for the classes of returns to scale, the innovation variables (taken from the PINTEC, as R & D spending was), and multinational. The level 4 firms tend to be larger, more innovative and to situate themselves in regions of constant or decreasing returns to scale, while the opposite is found for the level 1 firms. It is worth noting the high concentration of multinational firms in level 4 (36.9% of the singular exporters are multinationals in Brazil) .
Technically, the tests necessary to verify the differences between the distributions for the different groups are the Chi-squared, the Spearman correlation, and the residual tests (Agresti 1996). We do not report these tests, since it is sufficient to note that the Chi-squared tests reject the null hypothesis of equality of the distributions between all levels for all of the qualitative variables. The Spearman and residual tests indicate that there is a tendency for the extremes of the distribution to concentrate themselves in levels 1 and 4.
One can see that the group of non-exporters is formed in large part by firms with low indicators of competitiveness, which "pulls" the mean of those indicators downwards and is largely responsible for the differences between exporters and non-exporters presented in Table 1. This helps us to understand why estimates which follow 's decomposition find that the variations in total exports come predominantly from increases in exports by firms which already export, rather than from increases in the probability of exporting.13 13 In the tobit model, this decomposition separates the resulting effect from variations in the conditioning variables on the dependant variable in the following way: · E( y|X,y > 0) + P ( y > 0|X) · , where the first term on the right hand side represents the effect from increasing the export base while the second term represents the increased volume from firms that already export. Such estimates are not very encouraging when one considers expanding the export base.
Ellery Jr. & Gomes (2005) argue that, on average in Brazil, exporters sell 6.1 times more to the domestic market than non-exporters. This pattern is also seen in other countries such as France and the United States. In terms of industrial transformation value (ITV) , the difference is even larger according to Table 4; level four firms present an average ITV 9.45 times larger than the potential exporters (level 2). The division of the exporters into levels 3 and 4 demonstrates the heterogeneity of this group, given that the singular exporters have total sales 6.44 times and exports 9.64 times larger than the matched exporters. The potential exporters are slightly smaller, but have sales revenue 4.13 times that of the level 1 firms (directed towards the domestic market) .
In the extreme case in which all the potential exporters start exporting, given their similarity to the matched exporters, it is reasonable to assume that the average value exported in their debut year would be around US $ per firm. In 2000, this would imply an export increase of 14.7% for Brazilian industry and an increase in the export base of 62.5%, which would represent an intense energizing of Brazilian industry.
Regarding export volumes, a recent paper by Kannebley Jr. et al. (2010) showed that the longer a firm persists in export activity, the more it will export. As a matter of fact, the authors show that on average firms which have been exporting for two years manage to export more than double of those exporting firms which have been exporting for only one year; similarly, those which have been exporting for three years export more than three-fold new exporters. Though average exports do not keep on growing at the same pace after the third year and around 50% of new exporters fail to continue exporting14 14 Kannebley Jr. et al. (2010) argue that the persistence in export activity is related to two basic factors: pre-conditions before entry and entry costs at the sector level. The former positively affects the likelihood of survival in international markets, as well as the latter (the reason is that higher entry costs lead to higher hysteresis in export activity) . , the point is that the potential exports derived from new entrants in foreign markets may be non-negligible over time.
5.2 Where are the potential exporters?
As a result of the pattern of industrial concentration in Brazil, the potential exporters are concentrated in the states of São Paulo (45% of all potential exporters), Rio Grande do Sul (14.5%), Santa Catarina (9%), Paraná (7.9%) and Minas Gerais (6.6%). Leading the ranking of potential volume exported if all the potential exporters began exporting are São Paulo (US $ 2.38 billion), Rio Grande do Sul (US $ 562.08 million), Minas Gerais (US $ 549.16 million) and Paraná (US $ 404.51 million) .
Table 5 presents the distribution of firms by state and the volume exported by levels 3 and 4 in each state. Some states are aggregated to protect identifying information. Following the argument in the previous paragraph, one can assume that the volume which could be exported by the potential exporters is that which is already exported by the level 3 firms in the same state.
The potential export sectors15 15 Those sectors with an aggregate export potential of more than US $ 300 million (assuming that all potential exporters begin to export the same amount as the matched exporters) . are food and beverages (CNAE 15), textiles (CNAE 17) , leather, shoes, and travel articles (CNAE 19), timber, furniture and other industries (CNAE 20 and 36), and basic metallurgy (CNAE 27) , which are more traditional sectors. As expected, we do not identify great export potential in those sectors with large exports, but which are extremely scale intensive and/or which obey a unique dynamic of foreign trade (a dynamic determined by multinationals), such as transport equipment and chemical products, for example. These sectors' exports are highly concentrated in the singular exporters.
In Table 6 below, we desegregate by sector and level of export potential and report the average volume exported by level 3 and 4 firms in the chosen potential export sectors.16 16 A complete list of all sectors is available upon request.
5.3 Entry into and exit from foreign trade
If the potential exporters have such similar characteristics to firms which already export, then why do the level 3 firms export while the level 2 firms do not?
One of the answers, according to Table 7, is inertia: 76.8% of the potential exporters in 2000 continue as non-exporters from 2001 to 2003, while 85.3% of the matched exporters already exported from 1998 to 1999. Basically, what differentiates the matched exporters from the potential exporters is the fact that they have already exported before.
According to the international literature (Clerides et al. 1998, Roberts & Tybout 1995), this inertia is strongly associated with the existence of significant entry costs into the international market in the form of market prospection, product adequacy, the establishment of distribution channels, and logistics, etc. The level 3 firms export because they have already overcome these costs, or inversely, they don't leave international markets when they suffer negative shocks, in order to avoid re-entry costs.17 17 See Kannebley Jr. & Valeri (2006) for an extensive discussion about hysteresis and persistence in Brazilian exports.
Another possible explanation is the fact that the matched exporters may be integrated into the large worldwide production chains which form around the big players (usually multinationals). Such alliances with large companies, beyond reducing a significant part of the entry costs into foreign trade, permit special access to credit markets.
It also could be that the potential exporters fill very specific niches within a given sector, which the aggregated analysis which we have performed would be incapable of detecting. In this case, even though they present high levels of competitiveness, similar to firms which already export, these firms would face many difficulties entering into foreign trade. For the above reasons, future studies and analyses are necessary to determine the causes of this inertia.
6 Final comments and policy recommendations
In this article we have classified the Brazilian industrial firms in the certain extract of the PIA by their export potential. The classification was generated by a propensity score matching algorithm which was based on both theoretical and empirical studies of the micro-determinants of exports.
Rather than divide the firms between exporters and non-exporters, the classification comprises four levels, the most important of which identifies non-exporters with levels of international competitiveness similar to firms which already export. These firms constitute the preferential focus, at least in the short term, for policies and programs which aim to increase the export base.
What differentiates the potential exporters from the matched exporters is the fact that the latter group has already exported before. The precise causes of this inertia can only be determined by a study which focuses specifically on these potential exporters. There is an extensive literature which relates this inertia to fixed entry costs in international markets. The Agência de Promoção das Exportações (APEX-Brasil, the Export Promotion Agency) has been working (rather successfully) to reduce these entry costs by providing services to both exporters and potential exporters. However, this inertia may be attributable to the new dynamics of international commerce, with the increasing importance of integration into international production chains. This is especially true in Brazil, since strategic alliances with multinationals permit special access to credit markets and reduce exposure to economic volatility at the national and international level.
The expansion of the export base would not only bring many benefits to the Brazil's industry, but could constitute a viable option from the point of view of export volume amplification, especially if we consider the fact that the singular exporters may be insensitive to policies and programs to promote exports given their unique dynamics. However, the insistence on the argument for export base expansion does not mean that the other levels of export potential should be neglected.
Difficulties, such as sudden exchange rate movements or export regulation changes, lack of credit, distribution problems and others, when combined with an immature exporting culture, sometimes causes a firm to stop exporting. Araújo (2006) points out that productivity and size gains derived from the debut in exporting are only observable for those firms which keep exporting for at least two years. Hence, export promotion must also focus on stimulating firms which already export to remain in the international market, especially the matched exporters.
From a methodological standpoint, the principal contribution of this work is the alternative use of propensity score matching, which can be easily applied to other research problems (determining innovation potential, for example) .
Bibliography
Recebido em 14 de maio de 2009.
Aceito em 9 de agosto de 2010.
- De Negri , J. A. (2003), Desempenho exportador das firmas indutriais no Brasil: a influência da eficiência de escala e dos rendimentos crescentes de escala., Texto para discussão 997, IPEA, Brasília.
- Kannebley Jr. , S., Esteves, L. A., Silva, A. M. & Araújo, B. C. (2010), 'Auto-seleção e aprendizado no comércio exterior das firmas industriais brasileiras', Revista Economia .
- Agresti, A. (1996), An Introduction to Categorical Data Analysis, John Willey and Sons.
- Araújo, B. C. P. O. (2005), Os determinantes do comércio internacional ao nível da firma: Evidências empíricas, Textos para discussão, IPEA.
- Araújo, B. C. P. O. (2006), Análise empírica dos efeitos ex-post das exportações sobre a produtividade, emprego e renda das empresas brasileiras, in 'Tecnologia, Inovação e emprego', IPEA.
- Aw, B. Y. & Hwang, A. R. (1995), 'Productivity and the export market: A firm-level analysis', Journal of Development Economics 47, 313-332.
- Clerides, S., Lauch, S. & Tybout, J. R. (1998), 'Is learning by exporting important? micro-dynamic evidence from Colombia, Mexico and Marroco', The Quarterly Journal of Economics 113, 903-947.
- Davis, D. & Weinstein, D. (2003), 'Why countries trade: Insights from firm-level data', Journal of the Japanese and International Economies 17, 432-447.
- De Negri, F. (2004), Conteúdo tecnológico do comércio exterior brasileiro: o papel das empresas estrangeiras., Texto para discussão 1074, IPEA, Brasília.
- De Negri, J. A., Freitas, F., Costa, G. & Alves, P. (2005), Tipologia das firmas integrantes da indústria brasileira, in 'Inovações, Padrões Tecnológicos e Desempenho das Firmas Industriais Brasileiras', IPEA.
- Ellery Jr., R. & Gomes, V. (2005), Perfil das exportações, produtividade e tamanho das firmas no Brasil, Textos para discussão, IPEA.
- Girma, S., Greenway, D. & Kneller, R. (2004), 'Does exporting increase productivity? a microeconometric analysis of matched firms', Review of International Economics 12, 855-866.
- Gusso, D. A., Salerno, M. S., Moreira, R. L., Moreira, S. V. & Gehre, V. E. (2004), Promoção de comércio exterior: Experiências e aprendizagens, Textos para discussão, IPEA.
- Kannebley Jr., S. & Valeri, J. O. (2006), Persistência e permanência na atividade exportadora, in 'As empresas brasileiras e o comércio internacional', IPEA.
- Markwald, R. & Puga, F. P. (2002), Focando a política de promoção das exportações, in 'O Desafio das Exportações', BNDES.
- Mcdonald, J. F. & Moffitt, R. A. (1980), 'The uses of tobit analysis', Review of Economics and Statistics 62, 318-321.
- Meyer, B. (1995), 'Natural and quasi-experiments in economics', Journal of Business & Economics Statistics 13, 151-161.
- Ozçelik, E. & Taymaz, E. (2001), Does innovativeness matter for international competitiveness in developing countries? the case of turkish manufacturing industries, Technical report, Ankara: ERC.
- Parsons, L. S. (2001), Reducing bias in a propensity score matched-pair sample using greedy matching techniques, in 'Proceedings of the twenty-sixth annual users group international conference, SAS'.
- Pinheiro, A. C. (2002), Encarando o desafio das exportações, in 'O Desafio das Exportações', BNDES.
- Pinheiro, A. C. & Moreira, M. M. (2000), O perfil dos exportadores brasileiros de manufaturados nos anos 90: Quais as implicações de política?, Technical report, BNDES.
- Roberts, M. Tybout, J. R. (1995), The decision to export in colombia: An empirical model of entry with sunk costs, Technical report, Washington: World Bank.
- Tybout, J. R. (2003), Plant and firms level evidence on 'new' trade theories, in 'Handbook of International Trade', Oxford: Basil Blackwell.
- Wagner, J. (2002), Unobserved firm heterogeneity and the size-exports nexus: Evidence from German panel data, Technical report, Hamburg: HWWA.
- Willmore, L. N. (1992), 'Transnational and foreign trade: evidence from Brazil', Journal of Development Studies 28(2), 314-335.
- Wooldridge, J. M. (2002), Econometric Analysis of Cross Section and Panel Data, The MIT Press.


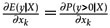

Publication Dates
-
Publication in this collection
04 May 2011 -
Date of issue
Dec 2010
History
-
Received
14 May 2009 -
Accepted
09 Aug 2010